Speeding Research With Data Mining Expertise
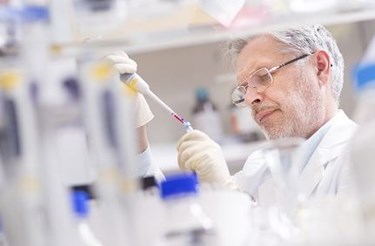
By Michael Iarrobino, Product Manager, Copyright Clearance Center
According to The STM Report (2015), more than 2.5 million peer-reviewed articles are published in scholarly journals each year. PubMed alone contains more than 25 million citations for biomedical journal articles from MEDLINE. The amount and availability of content for clinical researchers has never been greater – but finding the right articles to use is becoming more difficult.
Given the sheer volume of information, it’s almost impossible for clinicians to find and analyze the articles needed for their research. The velocity at which research needs to be done requires automated processes like text mining to find and surface the right content for the right clinical trial.
Text mining derives high-quality information from text materials using software. It’s often used to extract assertions, facts, and relationships from unstructured text in order to identify patterns or relationships between items. The process involves two phases. First, the software identifies the entities that a researcher is interested in (such as genes, cell lines, proteins, small molecules, cellular processes, drugs, or diseases). It then analyzes the full sentence where key entities appear, drawing a relationship connection between at least two named entities.
Most importantly, text mining can uncover relationships between named entities that may not have been found otherwise.
For example, take the drug thalidomide. Widely used in the 1950’s and 60’s to treat nausea in pregnant women, thalidomide was taken off the market after it was shown to cause severe birth defects. In the early 2000s, a group of immunologists led by Marc Weeber, PhD, of the University of Groningen in The Netherlands, hypothesized through the process of text mining that the drug might be useful for treating chronic hepatitis C and other ailments.
Text mining can speed research – but is not a panacea on its own. Licensing and copyright issues can slow productivity by as much as 4-8 weeks.
Here are three ways to maximize research productivity when leveraging text mining:
1. Use full-text articles, not abstracts.
Many researchers rely on article abstracts for use in text mining, because such content is easily accessible via biomedical databases and it’s typically provided in a format that’s suitable for text mining. However given the concise nature of abstracts, they often exclude, or underrepresent, data that’s less relevant or out of scope with the main idea of the publication. Abstracts also often don’t include essential facts and relationships, access to secondary study findings, or adverse event data.
To maximize text mining efforts, researchers should use full-text articles, as they provide detailed descriptions of methods and protocols, as well as complete study results. Full-text articles are also more likely than their abstracts to contain information on adverse events, and they contain more relationships between named entities than abstracts. In fact, according to a study published in the Journal of Biomedical Informatics, only 8% of the scientific claims made in full-text articles were found in their abstracts.
It’s also worth noting that while authors often include their most important findings in abstracts, critical insights like secondary study findings, discoveries, and observations are found only in full-text articles. Additionally, new discoveries are more likely to be mentioned in the full text of articles before appearing in abstracts.
2. Work with XML files, not converted PDFs.
The preferred format for mining full-text articles is Extensible Markup Language (XML). However, when researchers obtain full-text articles through company subscriptions or document delivery, often the documents are provided as PDFs, which is a suboptimal format for use with text mining software. Researchers are then forced to convert the PDFs — potentially thousands in a bulk delivery — to XML, which is incredibly inefficient and costly.
For best results, whenever possible, researchers should work with original, XML-formatted full-text content or leverage software to help them acquire such content. Because in addition to wasting time and money, converting documents from PDF to XML can cause problems with the original content, like loss of data and tables, conflation of document sections into a “blob of text,” or even the addition of bad characters and non-words. The conversion process can also result in poor character recognition for uncommon fonts, and tags can be inadvertently removed that indicate sections of the article, making the content difficult to mine.
3. Ensure the content is licensed for commercial mining.
The right to text mine content for commercial purposes isn’t always included in standard subscription agreements, so research organizations should carefully review a publisher’s terms and conditions before beginning any mining processes. Additionally, if researchers resort to converting their mining content from PDFs (which are intended for human consumption) into XML (which is intended for machines), be aware that this will result in the creation of additional copies. And creating and storing those reformatted copies will require further permission from the publisher.
Because text mining projects require such a broad base of content, researchers should consider working with a common set of terms and conditions for the licensed use of full-text XML content across publishers. With this approach, researchers won’t have to negotiate with individual rightsholders to obtain the content and right they need for text mining, and they aren’t faced with varying fee structures and inconsistent terms of use.
Text mining can drastically accelerate and enrich life sciences research. However, for the process to provide the most value, researchers should go beyond abstract-level searches, and download and mine full-text articles in XML format. They need to ensure the content they’re mining is licensed for commercial mining, and work with a common set of terms and conditions across publishers as much as possible. In doing so, researchers can focus on developing scientific discoveries, rather than wasting previous resources on article conversions, content management and negotiations with publishers.