A Data-Driven Approach That Could Change Mental Healthcare In 2025… And Beyond
By Greg Hajcak, Ph.D., chief scientific officer, Universal Brain
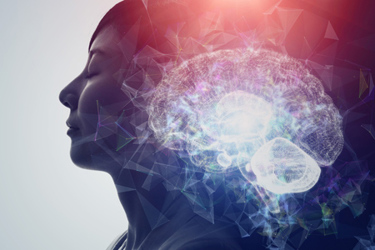
For over a century, mental health professionals have been diagnosing and treating mental health conditions based on their observations and patients’ reported symptoms. Clinicians gather this largely subjective information to determine whether a patient “meets the criteria” for a given disorder, according to the Diagnostic and Statistical Manual of Mental Disorders (DSM). The diagnosis of disorders is the requisite starting point for all clinical trials to evaluate new treatments.
The DSM permits a polythetic diagnostic approach, which means a condition requires many symptoms, but not all of them are necessary. This variability results in wide heterogeneity of patients with the same condition. A depression diagnosis, for example, can be reached using 200 different constellations of symptoms. As a result, two patients could both be diagnosed with depression — and have only a single symptom in common — like difficulty concentrating.
This wide heterogeneity combined with subjective clinical interpretation often results in imprecise treatments, frustrated patients, and poor outcomes. Momentum, however, is gathering around a data-driven approach, frequently referred to as “precision psychiatry,” that aims to personalize mental healthcare so patients can receive effective and tolerable treatments sooner.
The Search For Reliable Biomarkers
The data driving the growth of precision psychiatry involve biological measures. Researchers have studied many of these biomarkers for decades to identify and differentiate subtypes (or “neurotypes” when using brain data) of psychiatric conditions.
Some examples of biomarkers currently under investigation include memory, genes, cognitive performance, speech, hormones, and gut microbes. While some compelling associations have been published between these biomarkers and mental health, very few have yet been used to categorize patients into homogenous “biotypes” in the context of clinical trials. This approach could eventually lead to more targeted and effective treatment decisions.
One promising method for neurotyping involves measuring event-related potentials (ERPs), which are functional brain measurements collected through task-related brain activity evident in the electroencephalogram (EEG). These neural biomarkers, studied for decades, have consistently shown high test-retest reliability and interpretability across various mental health conditions. For instance, ERPs are associated with specific subtypes of depression and can accurately predict the course of depression and the outcomes of particular treatments.
The History Of ERPs
ERPs have been studied since the 1960s, primarily in the context of psychological and academic research, and have not yet found widespread use in clinical practice or pharmaceutical trials. Researchers have used ERPs to study a full range of cognitive domains, including memory, attention, vision, and emotion, to name just a few. In recent years, ERP biomarkers have helped unearth insights into mental health — including schizophrenia, which is among the most challenging conditions to manage for patients, families, and clinicians.
Promising ERP biomarkers of depression have focused on patients’ brain responses to rewards, emotional content, and decision-making and attention. Not only have these ERPs been linked to depression, but they also have accurately predicted individuals’ risk for depression and its development over time.
For example, a 2023 study of adolescents with depression found that an ERP associated with the response to emotional stimuli predicted a response to cognitive behavioral therapy. The finding suggested that this ERP could serve as a neural biomarker for predicting outcomes in adolescent depression.
A 2019 meta-analysis published in the Annual Review of Clinical Psychology concluded that ERPs can be used clinically to help identify psychopathology and chart the potential development of conditions beginning as early as childhood. One such ERP, the error-related negativity, was found to be instrumental in understanding the development of anxiety disorders and treatment opportunities.
A year later, the International Journal of Psychophysiology reviewed several ERP components associated with emotion regulation. Later research showed ERPs associated with receiving rewards were closely tied to depression and could predict remission. Combining emotion- and reward-related ERPs could help differentiate subtypes of depression and improve treatment precision.
Applying ERPs To Clinical Trials
Across numerous studies, ERPs measured through EEG have been shown to be instrumental in scientifically valid basic and applied research. There are significant opportunities for pharmaceutical companies to incorporate ERPs in clinical trials to develop more personalized treatments. Yet psychiatry has gravitated mainly toward using functional magnetic resonance imaging (fMRI) to measure brain activity. MRI-based imaging studies, however, are expensive and often cost-prohibitive for clinical trials and to utilize for tracking mental health. Given the cost and complexity of MRI, it is also difficult to scale this technology to serve a broad population of clinical trial participants or patients. Further reducing scalability, MRI requires technicians with specialized training and certification in some states. Lastly, MRI studies are not suitable for patients with claustrophobia or those with metal in their bodies. This could limit application across a broad population of individuals who would benefit from highly personalized mental health treatment.
Conversely, ERP data is imminently easier and less expensive to capture. EEG studies are relatively simple to administer thanks to technological advances in the decades since they were first studied. Using EEG devices does not require nearly as much training and experience to operate as MRI machines. In most cases, electrodes do not need a messy conductive gel to effectively capture ERPs, which makes the studies more tolerable for patients.
Above all, ERP measures of brain function have proven more reliable than fMRI-based measures. In clinical trial terms, EEG and ERPs’ reliability, speed, cost, and scalability can help pharmaceutical companies develop novel therapies in less time and by investing fewer resources.
Investigators can use ERPs to measure brain function at every phase. At participant recruitment, for example, a quick ERP assessment could promptly identify appropriate participants who would benefit most from a drug under development. This helps ensure homogeneity among different cohorts, supporting more streamlined clinical trials.
A Data-Driven Future
There are enormous advancements in data science techniques, such as generative AI. At the same time, ERPs are nearly a century old. Nonetheless, technological progress has simplified EEG studies and ERP data analysis, making these biomarkers more affordable, scalable, and easy to use for clinicians and patients.
For these practical reasons — as well as the growing accumulation of persuasive evidence published in peer-reviewed journals — we can expect ERPs to become more incorporated in mental health clinical trials in 2025. The time is right for ERPs to move from academic labs to real-world import. It is hopeful, if not likely, that ERPs will emerge in the coming years as a standard method of assessing brain function within the emerging and exciting field of precision psychiatry.
About the Author:
Greg Hajcak, Ph.D., is the chief scientific officer of Universal Brain and the Sheri Sobrato Professor of Child & Adolescent Mental Health at Santa Clara University.