A Prepared Approach To Technology In Clinical Trials
By Alishah Chator, Johns Hopkins University, and Kinari Shah, DIA
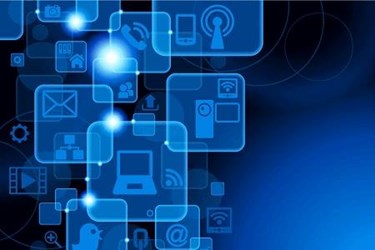
Advancements in technology create new avenues for optimizing clinical trials and the lessons that can be learned from them. With the gap between advancements in technology and regulatory uptake in clinical trials, it is important to rigorously examine technology applications. While the promise of new technology can lead to overenthusiastic adoption, mirroring the pace of other industries may not always lead to the best outcomes for patients. To increase regulatory buy-in to the use of these technologies, the clinical trial world needs a thorough understanding of the functionalities of each technical innovation, its potential utility and limitations, and how to build trust among patients, regulators, and other healthcare professionals.
This piece will take a critical look at the Internet of Things (IoT), natural language processing (NLP), artificial intelligence (AI), machine learning (ML), and blockchains, explore common pitfalls, and discuss ideas for a more prepared approach to utilizing technology advancements for data collection, analysis, storage, and access in healthcare. Such an approach would allow regulatory bodies to more easily assess the impact of a new application and for patients to benefit from these advancements at an accelerated pace.
Consistent Terminology
Before considering the impact of adopting one or more new technologies in clinical trials, it is important to have a clear understanding of the capabilities of each technology. Many current discussions of technological advancements refer to similar concepts interchangeably (such as artificial intelligence and machine learning), use incorrect terminology, or misidentify the area of application. Without consistent language, it is difficult to understand what a new innovation accomplishes. Once there is more clarity around each technological advancement that may be useful for handling clinical trial data, it will be easier to examine how best to adapt the different stages of data handling. Table 1 provides definitions and applications of technology innovations in clinical trials as well as where in the data handling process these technologies are a best fit.
Table 1: Taxonomy of New Technologies That Have Applications In Handling Clinical Trial Data
* Note: The CDC and FDA previously led a joint effort to create open source NLP platforms for national cancer data.
Data Collection
Collecting Data Using IoT
With the increased push for using real-world data (RWD) and real-world evidence (RWE) in drug development, healthcare professionals have turned to new technologies to collect data in the real world more effectively and reliably. With the help of the Medical Internet of Things (MIoT), for example, wearable and invisible devices (aka “wearables” and “invisibles”) are able to continuously collect real-time data from patients, even when they are at home. This “activities of daily life” (ADL) data may include a patient’s breathing and sleeping patterns, heart rate, exercise, and medication adherence, and can provide a more holistic picture of a patient’s life, potentially leading to better patient care and healthcare outcomes. Alongside collecting data, MIoT devices are connected to the internet and can transmit data or receive commands through this connection.
As with any new technology, however, there are a few important things to consider when introducing wearables or invisibles and the MIoT to a patient’s life. A minimally intrusive technology is important, as wearable and invisible devices can have a significant impact on the patient’s day-to-day life. If wearable trackers are not comfortable, versatile, and resilient, patients may be hesitant to use them. And if a wearable is not waterproof, patients must exercise added caution—a mental exercise they may not be capable or willing to perform. Clearly, the advantages of collecting data this way diminish if the continued, proper use of the device isn’t guaranteed, feasible, or comfortable. As this technology is maturing, a thorough assessment of patient comfort and ease of use will certainly aid in regulatory approval.
More concerning than comfort and ease of use, however, are the potential security and privacy vulnerabilities that these interconnected devices may introduce. Interactions between IoT devices are complex and not always transparent. They often lack thorough vetting for security vulnerabilities before they hit the market. These vulnerabilities can manifest in numerous ways:
- Devices may be directly at risk due to being interacted with in unexpected ways. For example, security researchers found that a home pacemaker monitoring system (connected to the internet) could be hacked in order to send pacemakers unauthorized commands. Such a vulnerability could result in a direct threat to the health and safety of the patient.
- Due to the interconnected nature of these devices, even if one device is secure, it may communicate with a compromised device and become a risk that way. For instance, a compromised IoT device hub could issue valid but unauthorized commands to the devices it manages, leading them to misbehave as well.
- The protocols for communication themselves can be a target if no measures are taken to improve the protocols’ security characteristics and reduce potential ways to attack. For example, researchers found a way to use a single hacked smart lamp to infect all the similar lamps over a large area with the same virus.
- Beyond the risks to patient safety, vulnerabilities could also compromise sensitive patient-specific data. Addressing privacy issues, by for example, de-identifying patient information, is critical to enhancing regulatory uptake of these data sources. Implementers of these devices should acknowledge potential privacy risks and be open to input from the broader security community.
Tighter regulation of these devices and more transparency to allow for security audits is necessary to build trust in this technology. To maximize effectiveness of introducing MIoT into real-world data collection, steps must be taken so that patients trust and feel safe that these devices will do what they are designed to do.
Collecting Data Using AI And NLP
AI and NLP also offer new methods of data collection. These methods take existing, but not always digitized, records and convert them into formats that are conducive to processing and analysis. For example, a systematic review of NLP use in clinical notes for chronic diseases shows that can be used for transforming circulatory system disease notes, as these records typically have more unstructured data. Another study found NLP can have significant contributions between pathology and diagnosis, extracting data from mammogram reports to support clinical decision-making.
An important consideration with AI and NLP is that the methods do not have total accuracy, which could impact the validity of the data extracted. These techniques are still quite new, and the use of these algorithms is not yet well-regulated. An exploration of adapting NLP systems to new settings found that existing studies overfit their techniques to a single setting. As a result, a great deal of manual effort was required to reuse the same system in a different setting. Additionally, researchers found that attempts to extract certain types of data, such as temporal information, often fail due to the diversity of ways this data can be expressed in language. Misclassification of data from a patient’s charts adds an additional source of error to the collected data. It is important to understand and properly characterize the impact of misclassifications to ensure that the collected data has credibility.
Data Storage And Access
New ways of data collection lead to more data that necessitates secure data storage systems. Privacy laws and regulations differ by region and country and add complexity to the safe storage and transfer of health information. For example, the introduction of GDPR (General Data Protection Regulation) in the EU introduces constraints on what types of data can be stored and how. An increased search for technological innovations that help store, move, and access data in a secure fashion and in line with these new regulations is therefore inevitable.
In this context, a frequently heard buzzword is blockchains. Blockchains are verifiable and publicly available and therefore provide high levels of transparency on what data is stored, including for patients. Smart contracts take this a step further by enforcing conditions on how data will be used. These conditions are public and corroborate adherence to a protocol.
With all the recent attention on blockchains, it is important to understand the limitations of this technology:
- Perhaps the most glaring shortcoming from a privacy perspective is the immutability of data stored in a blockchain. For example, consider a naïve strategy to add privacy to data stored on the blockchain where it is encrypted before being uploaded. If the encryption keys are compromised, the data is everywhere and cannot be removed. Even blockchains with stronger built-in privacy features have not solved this fundamental problem.
- Additionally, the complex nature of how security is achieved in a blockchain opens itself up to various edge cases where security is weaker than expected. For example, there have been multiple instances where less than 51 percent control of the network was necessary to undermine blockchain protections. So-called “permissioned blockchains” attempt to avoid such security issues by relying on more conventional ways of establishing trust in the network. However, if a system is no longer relying on the novel security mechanisms of a public blockchain, it is crucial to consider whether a blockchain is necessary at all.
Blockchains are meant for an environment where there is a need to share data, but it is unclear of who should be in charge of managing that data. This is useful when multiple independent parties would like to share a database without having to find a third party to administer it. Thus, blockchains are most effective when there is not a preexisting trusted party to rely on. In clinical trials and healthcare in general, where there is regulatory oversight, administrative bodies (e.g., the FDA) are well poised to offer the required trust and auditability for managing shared databases. This would avoid the costs of moving to a new technology as well as reliance on our nascent understanding of blockchain security and privacy.
Differential privacy and multi-party computation are potential options to overcome the shortcomings of blockchain technology. Differential privacy preserves underlying features of data without leaking any personally identifying data. This allows for the handling of data without sacrificing patient privacy. Multi-party computation (MPC) allow multiple policy domains to collaboratively examine data without directly sharing datasets. This allows entities, such as different regulatory agencies, to share data without adjusting to differing privacy standards. For example, with the combination of Brexit and GDPR complicating data sharing between the EU and the U.K., MPC enables collaboration without facing these roadblocks.
Data Processing and Analysis
Recent innovations offer ways to improve efficiency as well as uncover new patterns to inform new clinical designs to investigate. Machine learning, and artificial intelligence more generally, can take both structured and unstructured data and produce models of the underlying features. Clinical trials based on these models can thus be tuned more effectively. These models may also uncover directions of research that have not yet been explored.
An important consideration when using ML and AI is how to avoid bias. This includes avoiding bias in underlying data and models, as these will translate into ML and AI. Statistical bias is an issue in clinical trials and must be accounted for across trial design, data collection, processing, and analysis. AI picks up on patterns in data, so if there are biases represented in the source data, they will translate into the model. For instance, models trained on a standard language corpus were found to reflect historical biases on controversial topics.
ML is a powerful tool for predictive modeling, but it will not solve problems inherent to the data collected; those still must be targeted through bottom-up approaches and training with samples that have sufficient statistical power. Additionally, ML models tend to be very complex and, as a result, very hard to analyze. From a research perspective, this makes it difficult to identify the features that a model has extracted. Even if an ML algorithm uncovers a new biomarker target, it is often not apparent why it may be of interest. This creates a communication challenge, as it makes it more difficult to articulate the rationale behind a treatment. And from a patient perspective, the black box nature of these algorithms makes it difficult to trust their accuracy. With a lack of trust, however, these algorithms are not viable. The FDA and other regulatory bodies are exploring how best to oversee the use of these innovations. Demonstrating precisely how models are trained and how conclusions are drawn will aid in adopting AI- and ML-driven techniques in the interim.
The way data is processed to build these models also raises privacy concerns. As ML algorithms add layers of complexity on how the underlying data is used, this raises the question of how to add transparency to this process. As discussed, smart contracts alongside blockchains offer a potential way to regulate and audit how patient data is used to generate these models. Rather than feed the data directly into the models, smart contracts can allow for algorithms to run on the data without leaving the system the data is stored on. These contracts would also log how the data was used, and the ML algorithm’s operation is publicly verifiable. This would simplify the work for regulators in gauging the privacy implications of a new data processing technology.
Conclusion
Although new technologies provide the potential to transform clinical trials, a prepared approach to the responsible integration of these technologies is key. The technology sector moves at a rapid pace, but when patients are an important stakeholder and voice, the drug development and clinical world needs to exercise necessary caution. Pharmaceutical industry and clinical trial leaders should be adaptable and best equipped to effectively integrate these technologies. By increasingly using more consistent language, offering clearer justification for a technology amidst its limitations, and focusing on building trust, the industry can tackle these advances in a way that truly benefits drug development and patients.
About The Authors:
Alishah Chator is a third-year Ph.D. student at Johns Hopkins University studying computer science. He is part of the Advanced Research in Cryptography group and advised by Professor Matthew Green. He is currently interested in applied cryptography and broadly on problems in computer security that impact technology policy. In particular, he seeks to improve privacy by designing schemes with rigorously proven guarantees and auditing existing schemes to understand how privacy loss arises.
Kinari Shah is currently a research fellow at DIA. Previously, she attended Johns Hopkins Bloomberg School of Public Health and earned an M.S. in public health focusing on global disease epidemiology and control, with courses in pharmacoepidemiology and clinical trials. She is particularly interested in the intersection of nutrition, microbiome, and drug development.