Addressing The Problem Of Feature Selection Using Genetic Algorithms
By Munshi Imran Hossain
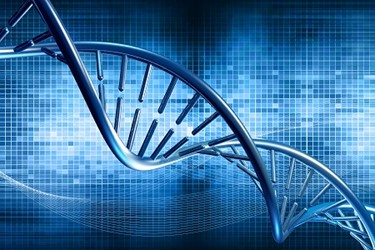
The problem of feature selection
The explosion in the availability of big data has made complex prediction models a conspicuous reality of our times. Whether in banking, financial services and insurance, telecoms, manufacturing or healthcare, predictive models are increasingly used to derive inference from data.
Most of these models use a set of input variables, called features, to predict the output on a variable of interest. For example, the concentration of characteristic biomarkers in the blood can be used to predict the presence, absence or progress of certain diseases.
The available data can provide a large number of features, but generally, it’s preferable to use a small number of really relevant features in a model. This is because a model with more features has a greater complexity which leads to greater demand on computational resources and time to train the model. Therefore it is desirable to restrict the number of features in a predictive model. Choosing the subset of features that will result in a model with optimum performance is the problem of feature selection. This is essentially a problem of plenty.
Get unlimited access to:
Enter your credentials below to log in. Not yet a member of Clinical Leader? Subscribe today.