AI And Healthcare Disparities: Driving A Wedge Or Closing The Gap?
By Peter Bannister, managing director of Romilly Life Sciences
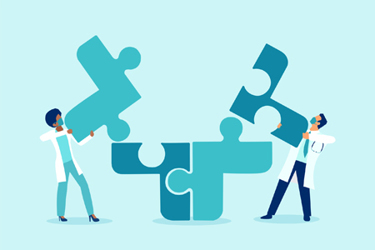
There are many reasons why we could be forgiven for thinking that the prospect of equitable, let alone preventive, health and care seems to shift further and further away into the horizon: healthcare systems are struggling to keep up with the increasing volume of data collected on patients, the last few years of the pandemic have only compounded treatment backlogs, including those for critical illnesses such as cancer, and the number of us living beyond retirement age and requiring some form of acute care continues to increase.
Given the current situation, it is understandable why many are looking to technologies such as AI — which seemingly promises to leapfrog today’s healthcare system capacity issues by rapidly augmenting if not replacing overwhelmed clinical experts with automated decision making based on real-world data inputs — as an effective solution for populations spanning diverse settings. However, realizing this technological potential requires us to consider the role of people and processes in an integrated approach.
AI Success Stories In Healthcare
Healthcare inequalities are a subset of health inequalities, which themselves can exist at local, regional, or international levels due to a wide range of socioeconomic factors aside from the capacity of the healthcare system itself. That said, if we consider the ways in which AI and other digital health technologies can overcome availability and access to healthcare services, there is a growing number of examples. This includes new screening diagnostics, software to identify novel drug compounds that are more likely to successfully progress through clinical trial to market, wearable devices to engage and retain patients in decentralized clinical trials (DCTs), and, more recently, chatbot software (for example, ChatGPT), which is being used to personalize the medical information that clinicians use when treating patients.
The Impact Of AI On Healthcare Is A Question of Access
The anticipation, if not impatience, many feel for the wider-scale impact that AI could have on healthcare is therefore understandable. Yet given the inherently high-tech nature of these innovations, it is important that we carefully consider how they will benefit those that do not currently have access to even relatively basic levels of digital infrastructure. An example would be the continuing trend to develop mobile phone apps to allow individuals to better understand and manage their own condition. Not all the world’s population owns a smartphone1, and lack of access is particularly marked among large populations such as China (68.4%) and India (46.5%), alone accounting for over 1.2 billion people. While looking more closely, other constraints exist. For example, in Brazil where mobile phone ownership is widespread, the cost of data plans makes the use of apps prohibitive, and other tools such as WhatsApp are favored by 99% of the 143.3 million people who own a smartphone2
AI Must Consider Proper Representation — The Who And How
When developing AI based on existing health data, it is fundamental that this information — training data — reflects the diversity of the patients it aims to help, for example, ensuring that software that seeks to automatically detect skin cancer has been developed and tested on examples of non-Caucasian patients. But when it comes to representation, the how is as important as the who: a recent study3 highlighted how algorithms used by U.S. healthcare insurers unfairly penalize those from marginalized communities as they do not consider the context (lack of transport to access care or absence of post-operative rehabilitation care facilities) that leads to poor compliance and outcomes for these groups.
Mapping The Whole AI Implementation Journey
And while for practical reasons we naturally focus on specific tasks that can be improved or delegated to AI, it is critical that any changes in the patient journey are assessed in terms of the full end-to-end clinical pathway: this is because innovations can lead to upstream and downstream effects as well as just local changes — all of which need to be rigorously evaluated. Think, for example, of an improved automated screening tool for cancer referrals: while the burden of increased radiology image reporting and increased hospital backlogs for cancer treatment is undeniable, a new method that is potentially more sensitive and able to detect cancer will have the effect of increasing clinical workload at the next stage, where additional suspected cases will now have to be investigated.
There is also the topic of trust and confidence in these new methods that must be considered – a clinical expert who has practised for many years reliant on manual diagnostic testing may rightly be sceptical of accepting results that have been produced by AI without appropriate and compelling evidence, which may in fact lead to more time needed to double-check the information than without the AI “upgrade.” Similarly, making screening available to patients who previously were not deemed high-risk enough may lead to resistance from those who may not wish to find out that they have an undiagnosed disease for fear of the impact it will have on their lives, as well as from those currently responsible for their care.
End-To-End Design
The good news is that there are established methods to avoid these pitfalls: we can and should lean heavily on a systems engineering approach, which is defined (by NASA and others) as a methodical, multi-disciplinary approach for the design, realization, technical management, operations, and retirement of a system. Here a “system” is the combination of elements that function together to produce the capability required to meet a need, in our case everything from technical compatibility with hospital electronic patient records systems through to the digital skills literacy of patients and clinicians, including concerns around being marginalized or displaced by the implementation of AI.
Co-Designing An AI Solution For Scale, Adoption, And Reach
Once we have comprehensively defined where AI could play a positive role in addressing healthcare inequality for a given problem, there are clear opportunities for meaningful and necessary collaboration between technical experts and other stakeholders. When initially defining the clinical problem we are trying to address, we can adopt a design thinking methodology, which is a nonlinear, iterative process that teams use to understand users, challenge assumptions, redefine problems, and create innovative solutions to prototype and test. Involving five phases — Empathize, Define, Ideate, Prototype and Test — it is most useful to tackle problems that are ill-defined or unknown4. In this process, patients, healthcare professionals, and other decision makers (for example, payers) play an active role in rigorously capturing what good looks like to inform subsequent validation that collects the most appropriate evidence, which in turn is essential to generating confidence in new approaches so they can scale, achieve sustained adoption, and reach the populations who need them the most.
To give a tangible example, imagine we have developed AI software that is now able to detect features in X-ray images that were previously imperceptible to even the most expert radiologist and show correlation with clinical outcomes for a currently underprivileged demographic. An engineer on their own will not be able to say if this pattern is practically useful, or even significant, without discussing the new feature with a clinical practitioner to understand if and how this insight could be used to improve care within the mapped-out clinical journey. There is also a requirement to understand how patients will routinely benefit from this insight: if the detection of this feature necessitates regular X-ray screening that is not readily available to the population it presents in, the benefit of this approach on its own is likely to be severely limited. Conversely, engaging early and often with the groups who have the greatest need for AI can proactively raise awareness of new treatment pathways, so they are better understood and accepted once implemented.
Clinical Evidence Is Needed For AI Validation
As already stated above, evidence of the right format and quality is fundamental for these methods to progress and be adopted. There is currently no shortage of innovation within healthcare. Yet, in too many cases, AI lacks the validation — whether it relates to accuracy on new patient groups, interoperability with homogeneous IT environments, or cost-effectiveness — and is stalled at the pilot stage. It may be tempting to think that this is due to a lack of clearly defined standards, but in the last few years, there has been real momentum and agility by industry bodies to define acceptance standards for AI. This includes specific adaptations to the CONSORT and SPIRIT clinical trial design and reporting standards5 as well as dedicated guidance for Clinical Decision Support Systems6, while regulators such as the FDA continue to publish increasingly detailed frameworks for the safe and effective development of such technologies7.
Quick Wins To Come, So Long As We Work Together
The enthusiasm for the role of AI in upgrading global health and care provision is justified, provided we understand that the path to success is just as much about communication and collaborative development as it is about the raw potential of the technology itself. If we recognize the role of systems engineering and design thinking, we will not only dramatically increase the likelihood of large-scale benefits from these innovative solutions, but we also will realize more immediate gains in the form of trustworthiness, patient engagement, and greater standardization of data collection and existing healthcare delivery. Once we commit to this path, we really can allow ourselves to imagine a future where technology has closed the gap.
About The Author:
Peter Bannister is managing director of Romilly Life Sciences, which provides evidence-led digital health product strategy and coaching to organizations of all sizes, from startups to global pharma. He is also honorary professor at the University of Birmingham Centre for Regulatory Science and Innovation and fellow of the Institution of Engineering and Technology. Connect with Peter on LinkedIn.
References:
- https://newzoo.com/insights/rankings/top-countries-by-smartphone-penetration-and-users
- https://www.forbes.com/sites/angelicamarideoliveira/2022/11/17/whatsapp-picks-brazil-to-launch-in-app-business-directory-and-shopping/
- https://www.healthaffairs.org/doi/full/10.1377/hlthaff.2021.01287
- https://www.interaction-design.org/literature/topics/design-thinking
- https://www.nature.com/articles/s41591-020-1034-x
- https://www.bmj.com/content/377/bmj-2022-070904
- https://www.fda.gov/medical-devices/software-medical-device-samd/artificial-intelligence-and-machine-learning-software-medical-device