AI In Drug Discovery And Development — Will It Live Up To The Hype?
By Matthew Featherstone, vice president, and Rowan Saada, consulting associate, Charles River Associates’ Life Sciences Practice
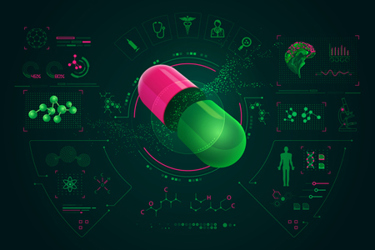
The recent explosion of generative AI has fueled interest in and concern over the use of AI in drug discovery and research and development. Already, the market for AI in drug discovery is estimated to be worth about $1.4B, and this is expected to increase to about $3.7B by 2027.1 AI-driven startups promise “unprecedented level[s] of efficacy and precision,”2 “revolutionary drug discovery engine[s],”3 and to “radically improve the timely creation of high-quality drug candidates.”4 Given the significant time and monetary investments associated with conventional drug discovery and development (see Figure 1A), it is no wonder that the hope for faster and more successful drug development has created considerable hype in the biopharmaceutical industry. However, while there is growing evidence that AI may transform the drug discovery and research and development process, there are still several barriers, both technical and ethical, to the rise of AI in the biopharmaceutical industry.
AI-Driven Biotechnology Is “Rewriting The Playbook For Drug Discovery”5
There is considerable excitement for the potential of AI to reduce costs and development timelines in candidate selection and preclinical research stages. The recent success of INS018-055, the first AI-generated drug to enter Phase 2 trials, suggests that AI may indeed deliver on these objectives6 (Figure 1A). Developed by Insilico Medicine, INS018-055 is a small molecule inhibitor designed to treat idiopathic pulmonary fibrosis. Both the undisclosed biological target and INS018-055 were identified using two AI platforms, PandaOmics (target identification) and Chemistry42 (molecule generation).5,6 Together, these platforms dramatically reduced the time to preclinical candidate selection from between three to six years to only 18 months,7 with an overall cost of only about $1.8M.5
This impressive feat does not appear to be a mere coincidence — similar trends have been seen across the industry. All three of Exscienta’s drug candidates in clinical trials were designed by AI in under 12 months,8 while Benevolent AI’s BEN-8744 was proposed for clinical studies within two years of program initiation.9 In academic settings, machine learning (ML) has accelerated the search for candidate antimicrobials, with Dr. Gary Liu and his research team using a message-passing neural network to identify a narrow-spectrum antibiotic against A. baumannii. The process of prediction and molecule prioritization was completed in a matter of hours, again demonstrating the power of AI in reducing early drug discovery time frames and costs.10
Could AI truly “rewrite the playbook” for drug discovery? We would argue that, in early drug discovery, AI is less rewriting the playbook than expanding its scope and accelerating its progression (Figure 1A). In many ways, AI follows the same processes used in conventional drug discovery — selecting a target based on scientific data and generating and screening compounds for target selectivity. However, the computational power supporting AI allows these processes to occur at a much greater rate and, in some cases, simultaneously. This accelerated process has the potential to benefit patients with high unmet needs, particularly those with rare diseases with no approved targeted treatment options. It also could help tackle the growing antimicrobial resistance crisis by facilitating rapid development of novel drugs, which may help researchers to stay ahead of the resistance curve.
Beyond drug discovery, AI has the potential to dramatically improve the efficiency and success rate of drug research and development by improving clinical trial planning and execution. Clinical trials represent an enormous challenge to pharmaceutical companies. On average, only about 10% of drugs advance from Phase 1 clinical trials to FDA approval and with every failure comes a big loss — costing about $0.8B to $1.4B and five to 10 years.13 Clinical trial service-focused startups have invested significant efforts to address these challenges with AI, and their platforms are already being used throughout drug development to reduce trial size, optimize recruitment, enhance data analyses, and simulate clinical trials (Figure 1B).
While these novel AI-based platforms have great potential to mitigate risk associated with clinical trials, it is worth highlighting that the use of AI in drug development is not as advanced as its use in drug discovery. As discussed below, this is largely due to greater technical and ethical challenges surrounding the data sets required in each case.
The Use Of AI In Drug Discovery And Development Still Faces Significant Barriers
The recent advances in AI technology also have brought to light the significant challenges facing its use in both drug discovery and drug development. In both cases, the analyses and predictions made by AI and ML software can only be as good as the data sets that support them. From a discovery perspective, this is primarily a technical challenge, as low-quality and poorly annotated data sets are more likely to yield non-viable drug candidates. Similarly, the use of AI in candidate molecule generation and screening often hinges on AI-generated protein structures, which in turn depend heavily on experimental structures. As such, the caliber of the experimental data used to support AI models is critical to their success.
The technical challenge associated with data set quality in drug development is far greater. The databases used in drug development, such as EHRs, are often extremely complex and heterogeneous,14 which makes it difficult to obtain and validate accurate predictions. Moreover, natural processing languages require data sets that are manually annotated and specific to different healthcare providers or indications, which takes a considerable amount of time and makes generalization of some AI platforms challenging.12
The use of AI in drug development also faces numerous ethical and regulatory challenges, as AI software can amplify existing biases within data sets.15 For example, biases in historical treatment decisions within training sets could be perpetuated in AI-driven analyses. Moreover, current clinical trial data does not often accurately represent population demographics, with underrepresentation of people of color, women, and the elderly.16 This is already a major issue in the pharmaceutical industry, as drugs may have varying safety and efficacy for different patient populations. These biases may be exacerbated given that clinical trial data is used to train AI. Thus, there is a need for careful regulation of bias mitigation and data set transparency. There has already been considerable pushback against the use of “black-box” AI models such as deep learning neural networks, in which the decision-making process of the system is difficult to interpret by humans.17 The need for transparency in AI models is further complicated by data protection requirements, such as the General Data Protection Regulation (GDPR) in the European Union and the California Consumer Privacy Act (CCPA) in the United States.13 These acts may restrict researchers’ use of AI tools and will certainly require AI models to be carefully designed to protect patient privacy while maintaining transparency. As such, legislation will be needed to enable the mainstream use of AI in drug development.
Conclusion
AI is already beginning to reduce the costs and timelines for drug discovery and, to an extent, research and development, making it a valuable resource for biopharmaceutical companies. With its processing power, AI may have the ability to identify novel candidates against “undruggable” targets,18 weed out unsuccessful candidates early in development, and accelerate clinical trials. This may reshape the drug discovery and development “funnel” (Figure 1A), allowing molecules from a vast chemical space to be screened, developed, and evaluated in a much shorter time frame. With the success of INS18-055, it seems as though AI may deliver on its promises. However, it is worth noting that the first AI-generated drug to reach Phase 1 clinical trials, DSP-1181, was discontinued as it did not meet the expected criteria.19 This highlights that the application of AI in drug discovery is not infallible. Further, there is currently a poor general understanding of AI technology and a considerable degree of mistrust toward AI systems. Human oversight and interpretation of AI predictions will almost certainly be required for the foreseeable future.
Matthew Featherstone is a vice president in CRA’s Life Sciences Practice. He has more than 20 years of consulting experience and specialises in commercial due diligence, portfolio optimisation, and pharmaceutical strategy development. He earned an MBiochem from the University of Oxford.
The views expressed herein are the authors’ and not those of Charles River Associates (CRA) or any of the organizations with which the authors are affiliated.
References:
- Artificial Intelligence (AI) In Drug Discovery Global Market Report 2023. (2023, February). Www.researchandmarkets.com. https://www.researchandmarkets.com/report/drug-discovery-ai#rvp-4
- AI Technology | Evaxion Biotech. (n.d.). Www.evaxion-Biotech.com. Retrieved July 21, 2023, from https://www.evaxion-biotech.com/aitechnology
- Pharma.ai. (n.d.). Insilico.com. https://insilico.com/platform
- Exscientia Highlights “The Future of AI-enabled Drug Discovery” at SLAS Europe. (n.d.). Investors.exscientia.ai. Retrieved July 21, 2023, from https://investors.exscientia.ai/press-releases/press-release-details/2023/Exscientia-Highlights-The-Future-of-AI-enabled-Drug-Discovery-at-SLAS-Europe/default.aspx
- Insilico Medicine Achieves Industry First Nominating Preclinical Candidate Discovered by AI. (2021, February 24). https://www.prnewswire.com/news-releases/insilico-medicine-achieves-industry-first-nominating-preclinical-candidate-discovered-by-ai-301234654.html
- First drug discovered and designed with generative AI enters Phase II trials, with first patients dosed. (2023, June 27). EurekAlert! https://www.eurekalert.org/news-releases/993844
- PandaOmics 3.0 - the tool for OMICS Data Analysis, Pathway Analysis and Visualization, and Target ID. (2022, November 25). https://www.youtube.com/watch?v=KW8tGAOzsgw
- Exscientia - Pipeline. (n.d.). https://www.exscientia.ai/pipeline
- BEN-8744 | Ulcerative Colitis | BenevolentAI Pipeline. (n.d.). BenevolentAI (AMS: BAI). https://www.benevolent.com/what-we-do/drug-programmes/ulcerative-colitis/
- Liu, G., Catacutan, D. B., Rathod, K., Swanson, K., Jin, W., Mohammed, J. C., Chiappino-Pepe, A., Syed, S. A., Fragis, M., Rachwalski, K., Magolan, J., Surette, M. G., Coombes, B. K., Jaakkola, T., Barzilay, R., Collins, J. J., & Stokes, J. M. (2023). Deep learning-guided discovery of an antibiotic targeting Acinetobacter baumannii. Nature Chemical Biology, 1–9. https://doi.org/10.1038/s41589-023-01349-8
- MD, G. L. (2023, July 13). AI Poised To Revolutionize Drug Development. Forbes. https://www.forbes.com/sites/greglicholai/2023/07/13/ai-poised-to-revolutionize-drug-development/?sh=32f3e91b7ca4
- Woo, M. (2019). An AI boost for clinical trials. Nature, 573(7775), S100–S102. https://doi.org/10.1038/d41586-019-02871-3
- Harrer, S., Shah, P., Antony, B., & Hu, J. (2019). Artificial Intelligence for Clinical Trial Design. Trends in Pharmacological Sciences, 40(8), 577–591. https://doi.org/10.1016/j.tips.2019.05.005
- Bhatt, A. (2021). Artificial intelligence in managing clinical trial design and conduct: Man and machine still on the learning curve? Perspectives in Clinical Research, 12(1), 1. https://doi.org/10.4103/picr.picr_312_20
- Alexandre Blanco-González, Cabezón, A., Seco-González, A., Conde-Torres, D., Antelo-Riveiro, P., Ángel Piñeiro, & Garcia-Fandino, R. (2023). The Role of AI in Drug Discovery: Challenges, Opportunities, and Strategies. Pharmaceuticals, 16(6), 891–891. https://doi.org/10.3390/ph16060891
- Giusti, K., Hamermesh, R. G., & Krasnow, M. (2021, June 11). Addressing Demographic Disparities in Clinical Trials. Harvard Business Review. https://hbr.org/2021/06/addressing-demographic-disparities-in-clinical trials
- von Eschenbach, W. J. (2021). Transparency and the Black Box Problem: Why We Do Not Trust AI. Philosophy & Technology, 34. https://doi.org/10.1007/s13347-021-00477-0
- Drug The Undruggable. (n.d.). Atomwise. https://www.atomwise.com/drug-the-undruggable/
- Q3 FY2022 Conference Call. (2023, January 31). https://www.sumitomo-pharma.com/ir/library/presentation/
- LPIXEL Science Biz. Dept. (2021). Harnessing AI to improve drug discovery. https://www.nature.com/articles/d43747-021-00042-w#
- Novadiscovery - Conquer the complexity of biology with clinical trial simulations. (n.d.). Novadiscovery. Retrieved July 21, 2023, from https://www.novadiscovery.com/
- Infographic: Accelerating Study Design Through Study Startup. (2023, June 21). Deep6.Ai. https://deep6.ai/resources/the-value-of-an-ai-enabled-clinical trial/
- Ricotti, V., Kadirvelu, B., Selby, V., Festenstein, R., Mercuri, E., Voit, T., & Faisal, A. A. (2023). Wearable full-body motion tracking of activities of daily living predicts disease trajectory in Duchenne muscular dystrophy. Nature Medicine, 29(1), 95–103. https://doi.org/10.1038/s41591-022-02045-1
- Readout AI Secures Pre-Seed Funding to Decrease the Time from Clinical Trial Data to Clinical Trial Insight. (2023, July 14). https://www.prnewswire.com/news-releases/readout-ai-secures-pre-seed-funding-to-decrease-the-time-from-clinical trial-data-to-clinical trial-insight-301877621.html