AI's Potential To Improve Clinical Operations, Trial Matching

By Tyler Menichiello, contributing editor
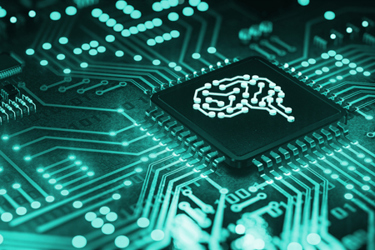
These days, it seems like nothing is as widely discussed (positively or negatively) as AI. Good or bad, the ball will continue rolling, so it’s important to dive head-first into the conversation. With the rise of generative AI models like ChatGPT, there come questions of how this technology can be used in industry, especially in healthcare. At this year’s DIA conference, Dr. Hoifung Poon, general manager of Microsoft Health Futures, spoke about AI’s potential to improve efficiency in healthcare and the life sciences industry. Though I didn’t attend DIA, I was lucky enough to speak to Dr. Poon about his work developing large-language models (LLM) for use in biomedical research. He says AI can be used in clinical research to improve patient care by improving clinical workflows and making it easier to match patients to trials.
Superhuman Curators
“What we really want is a data-driven, continuously learning health system where any new piece of information about a patient, trial, or drug can instantly feed back into the process.” The main challenge in achieving this is that medical data is largely unstructured. Unstructured data essentially refers to anything written or described in natural language, such as the information contained in a doctor’s note. “The reason they’re unstructured is because there’s a lot of variation,” Poon explains. For example, take type 2 diabetes, which can be referred to as type 2 diabetes mellitus or adult-onset diabetes. There’s also a lot of variation and ambiguity among medical acronyms, which can often have multiple meanings (e.g., ER and PDF). Structured data, on the other hand, is like the information in a database or numbers in a spreadsheet — it’s organized and neatly gathered in one place.
Right now, the healthcare system relies on humans (abstractors) to extract relevant information from medical notes and enter it into databases — to structure the unstructured data. For one patient alone, with potentially hundreds of doctors’ notes, this can take hours or even days. Multiply this by the number of patients on a clinical trial, and it puts into perspective just how time consuming (and costly) this necessary function of clinical research is. What Poon’s team is focused on is advancing AI technology to a point where it can automatically structure this data for the abstractors. The goal is not to replace these workers, but rather to “empower them to become superhuman curators.”
Keeping humans in the loop is important because, “despite all the advancement, the AI system is not going to be 100% anytime soon.” Because of the vastness of these datasets, there is potential for biases that humans need to watch out for, as well as accuracy problems. “What we’re focusing on is getting generative AI to not only produce a candidate answer, but to also provide an explanation of why it’s right,” Poon says. The human’s job in this scenario is to come in and quickly assess these answers, approving or rejecting them. In this way, they would safeguard the system and ensure accuracy. It also improves the system’s curation. “Every time a human fixes an error, it’s great feedback for the machine,” he says. In his team’s recent research, they showed that clinicians were able to verify results in 1-2 minutes using this assisted curation interface.
Trial Matching
One of the biggest applications for this technology in clinical research, Poon tells me, is trial matching. For many patients, clinical trials are their last hope. At any given time, there can be hundreds, if not thousands of applicable, active trials, so finding the right one for a patient is no easy feat. It’s a time-sensitive process of not only identifying the appropriate trials, but also ensuring that patients meet the eligibility criteria. This is where Poon thinks AI can really help. “The challenge right now is that all the information about a trial and the information about patients is all in this unstructured, free-text form, and that makes matching very difficult.” However, once the data is structured, “the matching becomes relatively straight forward.” He thinks using this technology to do “the heavy lifting” can improve efficiency and shorten the time it takes to get patients into trials. This human-in-the-loop trial-matching technology is already being used by Providence.
A Paradigm Shift
Poon says the emergence of this technology marks a paradigm shift in healthcare. “The exciting part is that this could potentially unlock a lot of new applications, including some that we haven’t even imagined yet.” While this kind of AI has promising utility in clinical trial matching, Poon says its use can be applied to the whole pipeline, from drug discovery to electronic data capturing and post-market, real-world data structuring.
He tells me that wide-scale adoption will require greater computing infrastructure and close collaboration among data scientists and trial coordinators. “Technology can only do so much,” he says. While technology can be a great catalyst, there are systemic changes that need to evolve to meet the inevitable challenges that will arise. Regulatory bodies need to meet these challenges head on and collaborate with developers to ensure responsible AI development and use. As Poon says, “the technology is very exciting, but it’s only a small part compared to these societal challenges.”