AI's Role In Advancing Rare Disease Research
By Harsha K. Rajasimha, Ph.D., and Deepti Dubey, Ph.D., IndoUSrare
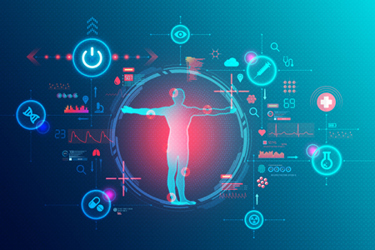
Rare diseases (RDs), though individually uncommon, collectively impact over 400 million people globally, presenting a formidable challenge to the healthcare community. The landscape of rare disease research is fraught with complexities, from inconsistent definitions to the scarcity of patient data. This article explores the global issues in rare disease research and examines how AI is poised to revolutionize the diagnosis and treatment of these often-overlooked conditions.
The Global Challenges In Rare Disease Research
While individually rare, the nearly 11,000 known RDs collectively impact over 400 million people worldwide. RD research and drug development faces multiple challenges globally. The crucial initial step in addressing RDs involves accurately identifying and cataloging them in knowledge databases, laying the foundation for diagnosing patients and progressing toward the development of effective treatments1. For nearly a decade, the count of RDs remained at 5,000 to 8,000, eventually being revised to 10,897 in a 2022 RareX report2. The discrepancy in the count stems from the inconsistent ways RDs are defined globally and the varying standards and measures employed by different knowledge bases when identifying such diseases.
Due to the low prevalence of RDs, patient data is both scarce and dispersed, posing significant limitations for research. Detailed studies on the pathology, symptoms, and disease progression for many RDs are often lacking. Patient registries and natural history studies, employing observational study methods to gather uniform data and assess specified outcomes for RD populations, serve as potent tools to overcome challenges stemming from small population sizes in research and clinical trials. The popularity of RD patient registries has surged, with over 800 registries listed in a December 2021 ORPHANET report. However, maintaining high-quality data collection in a registry and ensuring its relevance for future studies presents ongoing challenges3.
About 80% of RDs are genetic and present a range of phenotypic symptoms in patients with diverse genetic backgrounds due to genetic and clinical heterogeneity4. The complex and multifaceted nature of RDs leads to significant discrepancies in scientific understanding, clinical proficiency, accessibility to accurate diagnoses and treatments, patient outcomes, and overall quality of life.
Despite these challenges, recent advances in the low-cost and highly accurate DNA sequencing techniques and global databases have enabled the identification of causative genetic mutations and improved our ability to diagnose and understand RDs. Sequencing and “omics” technologies have made a significant surge in genomic data volume, necessitating the processes of selection, analysis, and integration5.
AI's Potential In RD Diagnosis And Progression
The use of AI, particularly machine learning (ML) algorithms, has garnered significant interest in recent years for its potential to reveal intricate patterns within extensive genomic data sets. These ML algorithms demonstrate the capability to learn from and act upon diverse data sets, enhancing the accuracy of RD diagnoses. Second-generation AI models focus on identifying clinical clues often overlooked in early RD identification. For example, ML has successfully identified systemic sclerosis patients at high risk of severe complications, detecting pulmonary involvement before deterioration, thereby improving survival rates and reducing healthcare costs6.
AI serves as a crucial tool for RD diagnosis, aiding in image recognition, genetic analysis, and supporting clinical decision-making. Algorithms are already being used to compile networks and registry information on RDs, facilitating the identification of new cases. For instance, a combination of brain function and structural imaging data can predict whether an individual with Huntington's disease (HD) will receive a clinical diagnosis within five years (pre‐HD) or provide quantifiable assessments of oculomotor function preceding HD. These applications showcase the promising potential of AI in the future of rare disease diagnoses6.
AI’s Advances In RD Treatment Development
Historically, drug discovery, burdened by a 90% failure rate, emphasized blockbuster drugs for larger populations to counterbalance financial setbacks. Initiatives like the Orphan Drug Act (U.S.) and the European Union Orphan Medicinal Products Regulation aim to incentivize RD drug development. Despite these efforts, only 5% of RDs have FDA-approved drugs, with a mere 15% witnessing the development of at least one promising drug for treatment, diagnosis, or prevention7. The challenging nature of the process is compounded by the potential for low revenue gains, resulting in expensive drugs, limited accessibility, and significant healthcare disparities for RD patients worldwide. However, the emergence of AI applications in new drug development and drug repurposing offers potential solutions to reduce costs and expedite the creation of treatments for RD patients.
Numerous studies demonstrate the use of ML algorithms in drug development, aiding in finding optimal therapeutic strategies, identifying disease pathways, and screening prospective therapeutic compounds in-silico8. Second-generation AI systems facilitate a patient-centered approach to RD treatment, adjusting regimens based on therapeutic responses and incorporating electronic data and patient-reported outcomes. These treatment response monitoring tools prove critical in diseases such as Gaucher disease, where patients show interindividual variation in response to multiple therapies6.
Drug repurposing stands out as an innovative and appealing option for the therapeutic development of rare diseases for several reasons, with the most significant being the potential for time and cost efficiency compared to de novo drug development9. AI tools also play a growing role in identifying drug repurposing candidates and analyzing vast knowledge graphs to predict new therapeutic uses for existing drugs. Platforms like EveryCure and REPO4EU utilize natural language processing and ML algorithms to uncover repurposing opportunities, integrating data from diverse sources including PubMed, clinicaltrials.gov, and medical records. Platforms such as OpenTargets and Biovista utilize AI to succinctly summarize evidence from the literature and calculate association scores between diseases and drugs or drug targets9. And platforms such as Jeeva clinical trials are unifying various fragmented point solutions into integrated systems for clinical trial management creating an opportunity to optimize operations using AI/ML.
Despite technological advances, challenges persist for drug repurposing in rare diseases due to limited data sets. However, collaborative efforts among biotech companies, academia, government organizations, and patient advocacy groups may streamline information compilation and aid in treatment development for rare diseases.
Lack Of Diversity In Patient Populations
AI tools predominantly train on data from large databases, such as GWAS/PheWAS, clinicaltrials.gov, EHRs, and the recently available U.K. Biobank’s whole genome sequencing database. However, these databases are primarily derived from populations of Caucasian descent, representing 10%-20% of the global population. Recognizing the potential bias introduced by inadequate representation, it becomes crucial to address the underrepresentation of diverse populations, including those of Asian, Middle Eastern, and African descent.
To fully realize the potential of AI in achieving inclusive and equitable universal health coverage, it is essential to ensure the participation of people with diverse backgrounds in genomic studies and clinical trials. This necessitates cross-border collaboration, active engagement with rare disease patient communities, and the sharing of resources and knowledge. Overcoming language, accessibility, and regulatory barriers is crucial for ensuring global participation in genetic testing and clinical studies. While some initiatives and organizations have made efforts to address these challenges by promoting collaboration and data sharing, more comprehensive endeavors are needed to drive innovation and advance rare disease research. Governments, industry leaders, and advocacy groups must collaborate to allocate sufficient resources, promote international collaboration, and streamline regulatory processes for the development of effective treatments for those affected by rare diseases. Organizations like IndoUSrare play a pivotal role in initiating collaborations and providing a platform for discussions crucial to rare diseases and orphan drug development, with the goal of equitable healthcare on a global scale.
References:
- Rare diseases: still on the fringes of universal health coverage in Europe. Lancet Reg Health Eur. 2023 Dec 11;37:100783.
- The Power of Being Counted. RARE-X report June 2022.
- A systematic overview of rare disease patient registries: challenges in design, quality management, and maintenance. Orphanet J Rare Dis. 2023 May 5;18(1):106
- https://globalgenes.org/rare-disease-facts/
- Improving diagnostics of rare genetic diseases with NGS approaches. J Community Genet. 2021 Apr;12(2):247-256.
- Artificial intelligence in rare disease diagnosis and treatment. Clin Transl Sci. 2023 Nov;16(11):2106-2111.
- Research on rare diseases: ten years of progress and challenges at IRDiRC. Nat Rev Drug Discov. 2022 May;21(5):319-320.
- Knowledge-based approaches to drug discovery for rare diseases. Drug Discov Today. 2022 Feb;27(2):490-502.
- Drug repurposing for rare: progress and opportunities for the rare disease community. Front. Med., 2024 Jan; 17 (11)
About The Authors:
Harsha K. Rajasimha, Ph.D., is the founder of the Indo U.S. Organization for Rare Diseases (IndoUSrare), a nonprofit organization with the mission to build collaborative bridges between various stakeholders of rare disease research between the U.S. and India, and founder of a venture-backed SaaS-based trial management solution on a mission to modernize clinical trials, improving efficiency and universal accessibility. Harsha chairs the annual Indo U.S. bridging RARE Summit to bring the stakeholders together to address grand challenges.
Deepti Dubey, Ph.D., is an accomplished researcher with expertise in molecular genetics, neurological disorders, and rare diseases from esteemed institutions. In collaboration with nonprofit patient organizations, she has driven research initiatives for accelerating breakthroughs for rare diseases. With a clinical trials specialization, Deepti is a scientific writer at Indo U.S. Organization for Rare Diseases.