Are Baseline Measurements Reliable? How RWD Can Help
By Terry Katz, senior director, biostatistics and data management planning and functional excellence, Daiichi Sankyo
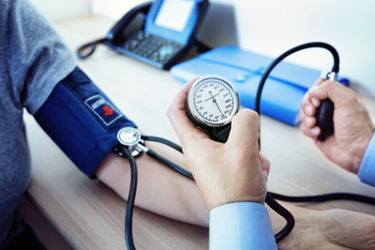
When a study begins, new study participants sign their informed consent form (ICF) and then undergo clinical measurements to determine if they meet the inclusion/exclusion (I/E) criteria. Along with these measurements are others that may be specific to the condition/disease being studied, such as laboratory blood samples for hematology, blood chemistry, and urinalysis.
But why take so many measurements?
These “baseline” measurements presume to establish physiological and/or metabolic status before introduction of test drug(s). Overall, they show the participant is either healthy or has the condition being studied. They establish that the participant meets enrollment criteria as part of the population of interest. On a variable level, they are used as a starting point, i.e., changes in these variables post-treatment would demonstrate the effect of a test drug.
Collectively, changes in these variables in a drug-treated group can be contrasted to a comparable control population who did not receive the test drug. At the participant level, the changes from baseline can show the individual study participants their benefit of receiving the drug by the lessening of the condition/disease-indicating variable magnitudes. Likewise, unfavorable changes in metabolic and laboratory variables can be detected and possibly classified as adverse events when measurements exceed the population normal ranges.
What If Baseline Measurements Aren’t Reflective For A Particular Participant?
Data quality is commonly established in relation to population norms, such as laboratory results falling in the normal ranges and confirmed by edit checks. Ranges can be wide, such as with systolic blood pressure in which readings range from under 90 mmHg to over 200 mmHg, with normal indicated as anything below 120 mmHg. Males and females, as well as pediatric versus adult versus geriatric populations, may have different but overlapping ranges.1 Depending only on population ranges, even when stratified by sex or age, may still not be enough to detect unexpected measurements for an individual patient.
For example, a metastatic non-small cell lung cancer patient who had a significant tumor growth trajectory history prior to receiving an investigational drug may represent a different disease situation than a patient with a static tumor burden, even though both patients are determined eligible for a trial. In that case, all or some of the attribution of positive or negative benefit may not be substantiated. Drugs may incorrectly be assessed as benefiting the participant, delaying a switch to a potentially more effective product. Adverse events may also incorrectly be attached to the product and thus unintentionally raise sponsor, IRB, or health authorities’ concerns.
Multiple Samples, Real-World Data Can Improve Reflectiveness
When a clinician takes a measurement, it is recorded in a patient’s electronic medical record (EMR). Clinicians, and clinical trial sponsors/CRO, then mentally or via a computer compare the measurement to the normal ranges for the given population. Most variables are measured only once per person per visit, including routine medical care or during measurement for a clinical trial. But some clinicians may go the extra step, and based on that patient’s history, decide if this measurement is consistent with previous measurements for that patient or declare this is a departure worth investigating.
Alternatively, multiple sampling could be built into the clinical trial protocol — regardless of intuition —and in alignment with American Heart Association instructions2. AHA suggests clinicians “take at least two readings 1 minute apart.” This adds a precision check to verify closeness of outcomes from multiple samples, i.e., precision is the amount of variation that exists in the values of multiple measurements of the same characteristic or parameter. Greater precision means less variation between measurements.3 If both blood pressure readings are similar in magnitude, then a higher confidence is placed on the value of that measurement outcome. This approach can be used both for baseline measurements and post-drug measurements. EMRs and clinical trial case report forms (CRF) may not, however, been designed to account for the multiple measurements.
But perhaps even more promising is making available the patient’s historical records for the clinician to judge whether the current reading is in line with historical readings. In a clinical trial setting, however, that real-world data (RWD) is typically not available. That could potentially be rectified by gaining approval from the study participant for proactive access to their historical records. Historical values could then be used to project the range for a new value to establish if a baseline is within or outside of historical expectations.
During the course of a trial, accruing measurement data could be tracked to determine if there are any trends (like a steadily increasing magnitude) or gross changes (like an abrupt increase that is considered clinically relevant). With the advent of Clinical Central [Statistical] Monitoring, detection of this type of trend is becoming more common during a trial. But at baseline, it has already been established that there is no knowledge of the historical magnitude of the variable for the study participant. Many patient portals, however, show years of historical data for the clinician to know means, high/low, and visualization of trends. Tolerance intervals to project ranges for individual measurements can potentially be determined4,5 to assess if the single baseline value is representative for that participant. If the clinical trial baseline measurement is outside of the expected range, it may reflect some acute underlying cause that misrepresents that participant’s norm, and a repeat measurement would be stipulated by the clinical protocol to mitigate a perpetuation during the post-treatment period.
There are challenges to this approach. Gaining access to the historical clinical data would require the study participant’s explicit authorization per data privacy policies and laws6. The ICF would need to clearly state the data collection of prior relevant medical records outside of the study period. Extracting that information might require support from the investigational site’s IT team. Source data verification can be a challenge since historical data was not recorded for medical research and could result in inconsistency with clinical trial data structure. Dynamically enabling the electronic database (EDC) to require and record each repeat sample would be another complication, as would final determination of “baseline.”
While there are complexities to work out, using the pool of existing real-world-data7 for participants is becoming more common in clinical trials. While the position presented is applicable for all clinical studies, appropriately characterizing “baseline” is paramount in early-phase trials that usually do not have large sample sizes and suffer more from unrepresentative “baselines.”
References:
- Centers for Disease Control and Prevention (CDC), “High Blood Pressure Symptoms and Causes,” https://www.cdc.gov/bloodpressure/about.htm, accessed 19 February 2023
- American Heart Association, Blood Pressure Measurement Instructions, https://www.heart.org/-/media/files/health-topics/high-blood-pressure/how_to_measure_your_blood_pressure_letter_size.pdf, accessed 27 December 2022
- American Society for Quality Glossary, Quality https://asq.org/quality-resources/quality-glossary/p
- Mary Natrella, Experimental Statistics, National Bureau of Standards Handbook 91, 2nd ed, 1966
- National Institute of Standards and Technology (NIST), Engineering Statistics Handbook, https://www.itl.nist.gov/div898/handbook/prc/section2/prc263.htm, accessed 10 January 2023
- European Commission, Data Protection, https://commission.europa.eu/law/law-topic/data-protection_en, accessed 12 February 2023
- U.S. Food And Drug Administration, Real-World Data: Assessing Electronic Health Records and Medical Claims Data To Support Regulatory Decision-Making for Drug and Biological Products Guidance for Industry [Draft], https://www.fda.gov/media/152503/download, accessed 10 January 2023
About The Author:
Terry Katz is senior director of biostatistics and data management planning and functional excellence at Daiichi Sankyo. Previously, he was head of global data management and statistics at Merck Animal Health; head of biometrics at ImClone Systems; senior manager of analysis & reporting for PRA International; and a statistician at Schering-Plough. He holds accreditation as a professional statistician and certifications as a quality engineer and a Six Sigma Green Belt. He is chair of DIA's GCP-QA Community. Formerly, he was a core committee member for NJ CDISC User Group and chair of the Statistical Taskforce for the Animal Health Institute. Terry also completed a three-month fellowship in Kenya to improve capacity and capability for the local hospitals to run oncology clinical trials.