Are We On The Cusp Of A Quantum Revolution In Clinical Trials?
By Scott Buchholz, quantum computing leader, Deloitte Consulting LLP
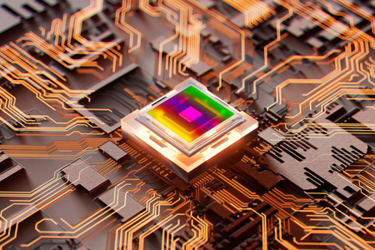
Ninety percent of drug candidates fail their clinical trials, often costing pharmaceutical companies billions of dollars and several years.1 The emergence of quantum computing can disrupt this status quo.
Clinical trials are the cornerstone of drug discovery, helping to ensure the safety and efficacy of new treatments. But traditional computers face several challenges with the increasing complexity in this pharmaceutical process, including areas like site selection, patient identification, and drug efficacy. Enter quantum computing, which leverages the principles of physics, instead of math, to process information beyond the binary restrictions of classical computing.
Though quantum computing is still in a nascent stage of research and development, life sciences companies are already investing in this technology that is poised to become mainstream over the next decade. Combined with advances in biotech and AI, quantum computing can offer promising solutions to improve clinical trial outcomes and optimize resource allocation. We delve into these impending transformations to clinical trials below.
Enhancing Predictive Models
Clinical trial simulations are crucial for predicting drug efficacy, side effects, and overall outcomes without conducting physical trials. Traditional machine learning (ML) and artificial intelligence (AI) methods, while effective, often struggle with the computational demands of simulating biophysical and physiological characteristics of molecules. This can lead to discrepancies between in vitro and in vivo studies, resulting in poor efficacy and toxicity predictions when transferring results from animal models to humans.
Quantum computing, on the other hand, with its ability to perform certain complex calculations much faster than current computers, can better simulate the outcomes of clinical trials and help companies narrow down the list of appropriate drug candidates, as Deloitte and others have written about elsewhere.
For instance, quantum machine learning (QML) and quantum simulation can improve the predictions in certain physiologically based pharmacokinetic (PBPK) models.2 These models describe the movement of drugs within the body and are essential for predicting drug behavior in different populations. Current techniques leveraging traditional AI models to solve PBPK problems require access to large and high-quality datasets for accurate prediction. QML algorithms can help address this issue by identifying patterns and correlations with less training data.3 With better prediction, life sciences companies would only be investing in the drug candidates with the most potential. Accordingly, it’s no surprise that pharmaceutical giants have already invested in developing quantum-related patents for their work.4
Improving Site Selection
A clinical trial site needs to have the necessary infrastructure and resources (such as staff, access to public transportation, etc.), but also, access to an area where enough participants can be recruited (especially considering factors like incidence of disease, environmental issues, regulatory requirements, etc.). Selecting the right sites for clinical trials is therefore a complex optimization problem that involves parameters as diverse as historical performance data, geographical considerations, and budgetary constraints. Traditional methods often rely on heuristic approaches, which can be time-consuming and prone to inaccuracy. Quantum optimization algorithms offer a more efficient and objective solution and allow for the evaluation of "what-if" scenarios based on different requirements.5
Outside of quantum optimization, QML also can help classify sites more accurately, even with limited historical data, which is often the case for the study of rare diseases. With QML, researchers can process and analyze complex demographic and medical information to better understand the level of patient diversity in clinical trial sites, a measure that is crucial to ensuring that trial results are generalizable across different populations.
Finding The Right Cohort
Similarly, identifying the right patient cohorts is critical for achieving statistically significant results. Traditional methods like rule-based systems can be manual and time-intensive, while data-driven techniques like ML/AI don’t always consider more personalized experiences. QML algorithms, on the other hand, could analyze complex biomedical data, such as electronic health records (EHRs), genomics, and imaging data to identify sub-populations with the highest likelihood of responding positively to treatments. Moreover, quantum feature maps can organize diverse data types in innovative ways beyond traditional computers’ capabilities. Quantum neural networks leverage natural patterns in medical data to improve performance and avoid issues like vanishing gradients.
In cases where patient recruitment is challenging, such as rare diseases, synthetic control groups can be used to supplement patient trials. Quantum generative adversarial networks (QGANs) generate synthetic patient data that mimics the statistical properties of real patients, while maintaining privacy and confidentiality.6 QGANs require less training data and could reduce the number of participants needed for trials, speeding up the process and reducing costs. That said, it’s worth noting the effectiveness of these methods depends on the specific type of trial data.
Conclusion
Quantum technologies hold immense potential to revolutionize clinical trials in the life sciences industry. By enhancing simulations, optimizing site selection, and creating new methods of cohort identification, quantum computing could usher in a new tech-powered reality; one where life sciences companies can expect more efficient use of their valuable resources and time. Life sciences executives should stay informed about these advancements and consider integrating quantum solutions into their clinical trial strategies. Embracing this transformative technology could pave the way to a better future of health.
Quantum computing also offers promise in drug discovery and preclinical development. Check out my companion article in Drug Discovery Online here.
References
- https://pmc.ncbi.nlm.nih.gov/articles/PMC9293739/
- https://www.sciencedirect.com/science/article/pii/S0165614724001676
- https://quantumzeitgeist.com/quantum-computing-applications-drug-discovery/
- https://www.clinicaltrialsarena.com/features/quantum-computers-drug-development/?cf-view
- https://quantumzeitgeist.com/quantum-computing-in-healthcare-transforming-medicine-and-research/
- https://www.sciencedirect.com/science/article/pii/S0165614724001676
About The Author:
Scott Buchholz is Deloitte Consulting LLP’s quantum computing leader and national emerging tech research director. He helps clients use technology to transform their organizations, missions, and businesses, and leads efforts in exploration of quantum computing and related technologies. He works across industries to provide actionable advice and insights to use technology to improve performance, effectiveness, and efficiency. In his role as CTO for Deloitte Consulting LLP’s Government and Public Services practice, he works with government clients to use technology to innovate in their operations, technology, and mission delivery.