Are You Overvaluing Your Clinical Trial P-Values?
By Ross Meisner and Bill Woywod, Navigant (a Guidehouse company)
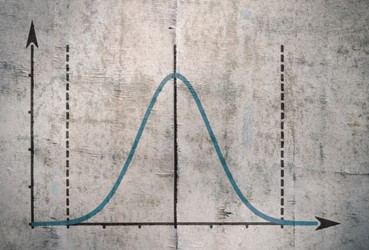
Could you be risking your business by relying on clinical study p-values to make critical decisions on research and development, market development, and commercialization strategies?
A growing body of statistical evidence shows putting too much reliance on p-values can — and often does — lead to false conclusions and misconceptions. Statisticians around the world are coming together, warning entire industries against rampant human misinterpretation and an overreliance on the concept of the acceptance threshold, as noted in a 2016 statement from the American Statistical Association. In fact, a petition recently signed by 800 statisticians calls for the elimination of this “statistical significance” concept.
In practice, p-values are used to weigh evidence against the risks of a hypothetical concept. So, why does the life sciences industry persist in gauging critical business decisions on the value of probabilities alone, especially as myriad examples of missed opportunities and wasted resources continue to accumulate?
P-Values Matter
Like the rest of the industrial and scientific world, medical technology and pharmaceutical companies commonly use a p-value threshold of 0.05, which means research results with a p-value of less than 0.05 are considered a positive indicator of the hypothesis set forth, and anything greater as negative. It is critical to keep in mind this threshold, while agreed-upon, is arbitrary.
Based on our collective experience researching, analyzing, and helping shift market dynamics for companies of all sizes around the globe, p-values serve as decent guides — but only as guides. Numerous examples exist within the life sciences industry in which companies gave up too soon on promising concepts — or invested too heavily in doomed ones — based on this threshold. While p-values do provide useful information, they do not tell the whole story. In other words, in order to use p-values effectively in determining whether to, say, advance to Phase 2 of a clinical trial, target a market subsegment, or cut bait on an innovation, you need to understand the data’s strengths, weaknesses, and the larger context.
Let’s take a look at how p-values frequently are misused in the life sciences industry – and how to use them to your advantage.
Get The Whole Story
Just as a borderline medical test result requires an experienced physician to interpret the result with knowledge of the test’s limitations in the context of all other available information, interpreting statistical results requires data experts. Consider by definition, p=0.10 means there’s a 90 percent chance of a true difference between two study populations, given the observed effect size. Conversely, with a p-value of 0.05, there’s a one in 20 chance that the two study populations are the same. Without investigating the data further or conducting additional studies, a research team could make the mistake of thinking a therapeutic option is significant, or vice versa, based solely on a single p-value. Rather, the research team should consider the information provided by the study directionally, and quantify and accept the uncertainty inherent in statistical inference.
Biogen’s recent Alzheimer’s treatment news represents a good example of how this might play out in real life. After sinking hundreds of millions of dollars into developing the experimental drug, the pharmaceutical company initially tabled its research due to futility. Then, new analysis of a larger data set from Phase 3 showed the drug was effective in one of the two studies in patients who took the highest doses for the longest duration. As a result, Biogen shifted gears again, announcing last October plans to submit a Biologics License Application to the U.S. Food and Drug Administration in early 2020 for its potential breakthrough treatment.
Critical Value Mistakes
Biogen's experience serves as a testament to why it is important to take the time and expertise to dig deeper into research results to avoid costly course corrections and/or missed opportunities.
Critical probability issues tend to arise when a study is underpowered, overpowered, does not account for statistical variability, and/or is based on bad data. Here are real-world examples of how these common research and analysis pitfalls can manifest:
- Thinking a therapy option is significant that isn’t, or vice versa. Based on the seemingly significant results of a small sample size, a company with an interventional renal denervation therapy decided to conduct a much larger study, only to find the results did not extrapolate to a more loosely defined patient indication.
Conversely, in another case, Pentoxifylline, an inexpensive drug with mild side effects already approved for the treatment of intermittent claudication, was tested for resolving recurrent venous leg ulcers. A 200-patient randomized controlled trial of the drug showed a 20.7 percent improvement in healing rates over placebo, but it failed to reach the p=0.05 level. Deemed as “non-significant” results, likely due to underpowered studies, the drug failed to gain approval for the treatment of venous leg ulcers. Later, meta-analysis found the drug is an efficacious treatment for venous leg ulcers.
- Particular-value hacking and multiple comparisons. After getting poor results in a randomized controlled Phase 2 trial, a biopharmaceutical company conducted a large number of post-hoc analyses on randomly selected subpopulations. One subpopulation happened to produce a significant effect in the Phase 2 post-hoc analysis, and this population became the focus of a Phase 3 trial. The Phase 3 trial also failed, and the project was terminated — after the company arguably wasted significant resources. A better approach to garner more meaningful and actionable data would have been for the company to use the best available evidence or clinical judgment and target the likely most appropriate subpopulation from the outset.
- Overlooking whether a therapy option is “meaningful”. Whether a therapeutic will make a significant impact in practice or material effect on clinical outcomes cannot be derived from a p-value alone. In a large data set originating from administrative data, even very small differences will be significant, but not necessarily meaningful enough to drive adoption in the real world. For example, a pharmaceutical company’s clinical study of 2,200 patients found its topical healing solution improved outcomes in 4.8 versus 5.5 days, with pain diminished from 4.1 days to 3.5, both of which equate to p<0.001. However, the treatment option would cost several hundred dollars out of pocket, and government payers already covered the standard of care. So, while the p-values were compelling, the costs far outweighed the clinical benefits and thus were a critical adoption barrier.
Figure 1
- Publication bias. P-values fail to address issues with the data itself, meaning, a study’s results – good, bad, or neutral – might be misleading in any case. Sometimes, deeper analysis will reveal missing data, publication bias (which can encompass an entire field of inaccurate literature), or other issues. For example, in a recent market-sizing project, Navigant, a Guidehouse company, analysts conducted a comprehensive data review of oncology progression studies and found clear publication bias (see Figure 2). The asymmetrical funnel plot indicates a series of studies were missing, and further independent analysis revealed an extremely low progression rate for the condition being investigated. If this bias had gone undetected, the medtech company likely would have grossly overestimated the market size of its new product and targeted a launch at a patient population that does not exist.
Figure 2
Get It (Closer To) Right
These are just a few of the common ramifications of focusing too narrowly on one statistical result, rather than looking at the full context. Of course, when it comes to the life sciences market — or any market — there are no guarantees. But you can shift the odds in your business’ favor:
- Choose a meaningful value proposition and prove it works. For example, proactively design your clinical trials to use the best available evidence or clinical judgment to intentionally focus on the subpopulation in which the therapeutic likely will be most efficacious. While this will not guarantee positive trial results, it will help garner more relevant and actionable data to inform further research and development activities. You can always invest to expand indications later, after you’ve demonstrated the best possible clinical impact on those “perfect patients.”
- Invest the time and resources in conducting a comprehensive review of all relevant data, and synthesize those data using best-practice methodology to uncover latent biases, such as publication bias.
- Apply the appropriate statistical expertise to interpret your research in full context. As we discussed, while p=.05 is useful as a rule of thumb, it’s not alone sufficient to bet your business on it. When the stakes are high, a very deep dive is essential.
Those life sciences companies that understand how to apply and interpret p-values as just one factor in informing critical decisions will be better positioned to achieve significant market success.
About the Authors
Ross Meisner is a partner in the Life Sciences practice at Navigant, a Guidehouse company, and has 30 years of experience building high technology companies. In 2007 he cofounded Dymedex Consulting to become the thought leader for understanding medical market dynamics. Additionally, he cofounded and held leadership positions at four other start-ups and two international joint ventures. His experience spans medical technology, management consulting, internet financial services, and the semiconductor industry. Prior to Dymedex, Meisner was director of Global Market Development at Medtronic, implementing new methods to identify untapped growth opportunities across geographies.
Bill Woywod, MPH, MBA, is a managing consultant in the Life Sciences practice at Navigant, a Guidehouse company, where he leads big data analytics and machine learning projects. He has played a central role in expanding Navigant’s deep data analytics capabilities, including the development and execution of sophisticated claims data analyses and machine learning initiatives for clients in the medtech and biopharma industries. Previously, Woywod worked as a data scientist at a large regional health plan, where he led the development and successful execution of the organization’s first machine learning program.