Artificial Intelligence And Machine Learning/Translation In Pharma Language Translation
By Mark Wade, TransPerfect Life Sciences, Global Practice Leader
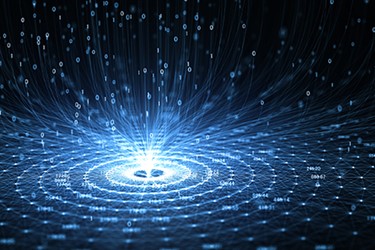
AI, in practice, has a lot of potential and also a lot of limitations. AI is being used to model, extrapolate, and automate tasks throughout the lifecycle of a drug (as it should be). The potential benefits are substantial, both in terms of clinical efficacy and financial savings. The low-hanging fruit was big data analysis for drug interactions, as the data is easily consumable and analyzed by today’s AI. Amassed medical data on drugs can be easily compared against patient medications to alert study teams to potential risks. Patient recruitment, monitoring, and retention by way of analyzing large EMR data sets, both structured and unstructured, is a point of focus and is a similarly accessible and important use case for AI. Pinpointing patient heterogeneity, securing patients who are more likely to match the ever-increasing inclusion/exclusion criteria and also have the capacity to respond to certain treatments.
AI, and in this case, neural language processing (NLP) could vastly speed up electronic phenotyping, which is a complex task of reviewing EMRs to find that suitable heterogeneous target patient population.
With all of the supposed power and promise of this advanced technology, what are the real, practical implications for clinical outcome assessments?
Get unlimited access to:
Enter your credentials below to log in. Not yet a member of Clinical Leader? Subscribe today.