Automating Quality Assurance In Neurology Trials Using Speech Analytics
By Celia Fidalgo, Ph.D., head of product, Cambridge Cognition, and John Harrison, chief scientific officer, Scottish Brain Sciences
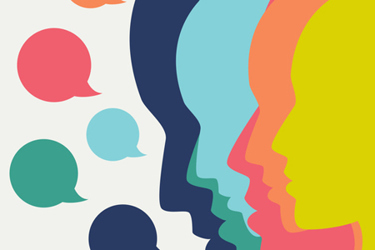
Clinical assessments in neurology play a pivotal role in tracking a condition’s progression and providing valuable insights into the efficacy of potential treatments. These assessments demand specialized expertise to ensure accurate data collection and analysis, often necessitating extensive training for the raters responsible. The evaluations of these assessments are scrutinized to identify any discrepancies in administration and scoring and, while they yield vital insights, they are costly due to their manual nature and are challenging to scale. As a result, many assessments go unexamined. This challenge can be addressed with the automation of quality assurance (QA) within clinical trials by using speech analysis, flagging assessments with the highest likelihood of quality issues for further review.
Challenges With Clinical Trial Outcome Measures
One challenge with clinical trial outcome measures is their divergence from the standards typically used in clinical practice. A good illustration of the divergence is the measurement on constructional praxis. For example, it is commonly the case that the Alzheimer’s Disease Assessment Scale-Cognitive Subscale (ADAS-cog) Constructional Praxis copied figures are photographed and sent for central rating. However, clinical assessment of constructional praxis (e.g., of the Rey-Osterreith figure) requires evaluation not just of the final copy, but also of the process by which the copy was made.
A talent pool is scarce for conducting these trial-specific assessments, necessitating additional effort from the clinical research professionals involved. This added workload comes at a cost, with many sites reporting that raters spend a significant amount of time preparing to administer outcome measures that hold little applicability outside the realm of clinical trials.
Another challenge is that while research programs typically commence with a strong emphasis on quality and seek experienced raters at reputable sites, the initial rater assigned to a study may not be the same as the one who concludes it. A high turnover rate among site raters adds to this challenge. This issue, exemplified by the continuity issues, can, for example, significantly impact an 18-month study on Alzheimer’s disease, demanding careful management.
Where Can Automation Add Value?
Novice raters often encounter challenges with specific aspects of assessment scales, such as the Alzheimer’s Disease Cooperative Study – Clinical Global Impression of Change (ADCS-CGIC), Clinical Dementia Rating (CDR) scale, and ADAS-Cog, especially in areas involving language skills like speech comprehension, speech production, and word-finding difficulties. Administering these assessments typically demands specialized healthcare training. And so, automating assessments presents an encouraging solution to address these hurdles, offering the potential to ease raters’ burdens.
Consider this scenario: Assessment scales frequently emphasize inquiring whether a study participant can perform specific tasks, such as recognizing previously shown words with simple “yes” or “no” responses or even something as fundamental as making a fist. Clinically, there is an understanding that individuals in the earliest stages of Alzheimer’s disease and other related conditions can often complete these kinds of tasks. However, it is noteworthy that if you ask whether the task now takes them more time than it used to, patients frequently acknowledge that it now takes two or three times longer.
Conventional scales have failed to capture this aspect. When engaging individuals deemed functionally intact, a brief conversation quickly reveals that their functional abilities may not align with the initial assessment under a different line of questioning or evaluation. Although they ultimately complete tasks, it becomes evident that they require significantly more time. Technology presents a promising avenue to begin capturing these features.
We now find ourselves in an era where novel therapies for people living with Alzheimer’s disease are receiving FDA approval or are on the verge of it, with numerous potential treatments progressing through the development pipeline. One persistent challenge is determining clinical significance. Data must frequently be revisited to uncover valuable insights from the conversations between raters, study participants, and caregivers. This involves delving into paper case report forms (CRFs) for specific assessments. If this data were to be seamlessly captured and automatically extracted, it would enable clinically meaningful information to be identified as an integral part of the automated process.
Expanding on the theme of accuracy, specific aspects of the Montreal Cognitive Assessment (MoCA), as an illustration, warrant thorough capture and analysis. Let’s consider its verbal fluency component, which is scored using a binary scheme — 11 or more earns a score of one, while less than that results in a score of zero. This measure can extract extra value by analyzing the raw score data. Verbal fluency paradigms provide robust, reliable, and valid assay-sensitive assessments with a strong track record of detecting treatment effects. The prospect of automated data capture in this context opens an additional avenue for delving deeper into potentially highly valuable data.
Finally, ensuring consistency between objective and subjective assessments can be a challenge. What stands out is the need to reconcile a participant’s and caregiver’s impressions with formal assessments. Typically, this kind of data is most often considered retrospectively. However, a rater can take only so many notes during an ADAS-Cog or CDR assessment. Automation can support raters in conducting assessments, delivering quality improvements and the chance to capture more insightful data.
Integrating Automated Quality Assurance (AQUA)
Automated Quality Assurance (AQUA) begins with recording assessments, including the ADAS-Cog, CDR scale, and ADCS-CGIC. Recordings can be obtained by importing externally collected recordings from smartphones or tablets or by integrating speech collection into an electronic clinical outcome assessment (eCOA) platform. Advanced eCOA platforms may already incorporate integrated delivery of these cognitive scales. Once a recording has been collected, each speaker is isolated, and their speech is transcribed. This identifies the rater and the participant, as well as denoting any other speakers present in the recording.
The subcomponents of the assessments are then labelled. This labelling process is carried out with expert clinicians on a scale-by-scale basis. To illustrate, in the case of the ADAS-Cog, distinct subcomponents, such as Word Recall and Commands, have clearly defined segments, each accompanied by its own set of scripted questions and scoring criteria. These subcomponents also have unique statistical characteristics, such as expected duration and guidelines regarding the presence of specific individuals in the assessment room. Hence, this comprehensive separation becomes essential to maintain clarity and accuracy throughout the assessment process.
Once the distinct elements have been isolated, each undergoes analysis through a processing pipeline. Features can be extracted from the transcript and audio recording of each subcomponent. These features encompass a wide range of aspects, including audio duration and the frequency of pauses, which help determine if a subtask adhered to its expected time frame and whether the rater was adequately prepared. Furthermore, this analysis can identify specific criteria, such as whether the rater’s transcript contained the required words per that scale's established guidelines. This way, disparities between the transcript and audio compared to expected standards can be picked up. These identifiers encompass variations at the scale level, the study level, and the subtask level.
At the study level, factors such as rater consistency over time can be identified. Likewise, participant duplications can be investigated to uncover instances where individuals have enrolled in a trial more than once, possibly at different sites. On the scale level, there is the capability to detect nuances such as the clarity of the rater's speech and their level of preparedness. Potential distractions from background noise or unexpected speakers in the room also can be identified. At the subtask level, it is possible to scrutinize whether the rater adhered to the prescribed administration guidelines. This involves assessing if they followed the subtasks in the intended sequence, whether they altered the meaning of a question, and if they accurately scored the responses.
Once complete, a comprehensive report can be created on the identified quality tags, revealing the number of concerns associated with each scale. They are categorized into major and minor issues and can be quantified per scale and per trial. A major issue could entail a significant deviation from the guidelines by the rater, resulting in the intended meaning of a question being significantly altered. A minor issue might involve occasional distracting background noise during the assessment process. Sponsors are actively involved in determining the classification of issues as major or minor within their respective trials, allowing for a tailored approach that aligns with their specific requirements and priorities.
Data Validation With AQUA
As previously mentioned, automated approaches can detect rater changes and identify duplicate participants. Advanced machine learning speaker verification models analyze the acoustic properties of multiple voices to ascertain whether they belong to the same or different individuals. Across different data sets and languages, voice detection models demonstrate remarkable accuracy rates. In the case of English-language models, a demonstrated accuracy rate of 90% has been achieved in detecting rater changes and participant duplications.1
Quality monitoring platforms facilitate efficient review of recordings and labelling quality issues. Additionally, they serve as a valuable tool for annotating data sets and training machine learning algorithms geared toward quality assessment. Testing has demonstrated that individuals without clinical expertise can proficiently identify quality concerns, and their reviews align closely with those of expert clinicians. This approach offers a cost-effective alternative to relying solely on clinical experts.
AQUA can be applied to a diverse range of scales, enabling the identification of various quality indicators and adherence to administration guidelines. Summaries of these indicators are provided to clinicians, expediting the review process and ensuring a focus on the most critical issues.
A Future With AQUA
Ultimately, the goal is to maintain objectivity, consistency, and the highest quality standards in disease measurement. The provision of real-time quality indicators serves as a crucial tool in achieving the timely identification and resolution of deviations, minimizing the potential impact of errors on disease measurement. This, in turn, expedites the detection of effective treatments.
It is anticipated that in the years to come, most rater-administered scales will incorporate immediate, automated feedback that will help raters administer scales in a highly consistent manner. While establishing rapport will always remain critical in clinical assessments, automated feedback is poised to become the standard method for ensuring raters adhere to guidelines. Anticipated to become the gold standard for complex scales in global CNS trials, this approach marks a significant advancement in precision and reliability in clinical development.
References:
- Malikeh Ehghaghi, Marija Stanojevic, Ali Akram, and Jekaterina Novikova. 2023. Factors Affecting the Performance of Automated Speaker Verification in Alzheimer’s Disease Clinical Trials. In Proceedings of the 5th Clinical Natural Language Processing Workshop, ACL.
About The Authors:
Celia Fidalgo leads product development at Cambridge Cognition. She shapes how speech analysis tools are used in novel applications within healthcare and clinical trials, working cross-functionally with R&D and machine learning teams to develop innovative solutions. Celia obtained her Ph.D. in cognitive neuroscience from the University of Toronto, studying aging and memory loss in Alzheimer's disease.
Professor John Harrison is a cognition expert whose principal professional interest is helping people understand, maintain, and enhance their cognitive skills. John is chief scientific officer at Scottish Brain Sciences, a clinical trial and neuroscientific research company. In the past 25 years, John has assisted more than 80 CNS drug development organizations with the selection and integration of cognitive testing into therapeutic development programs. His work in Alzheimer’s disease dates back to his participation in the studies of compound AN1792, for which he developed the Neuropsychological Test Battery (NTB). Variants of the NTB have since been a common feature of CNS focused treatment development programs. His work in psychiatric indications includes the development and registration of Brintellix. John is also an associate professor with the AUmc Alzheimer Center and visiting professor at King’s College London. He holds Chartered Psychologist status and has authored/co-authored more than 100 books and scientific articles, including a popular neuroscience book, “Synaesthesia: The Strangest Thing.”