Bayesian Adaptive Designs in Clinical Trials: Using Data Science To Optimize Investigational Product Supply
By Christopher Ohms, Ohms Consulting
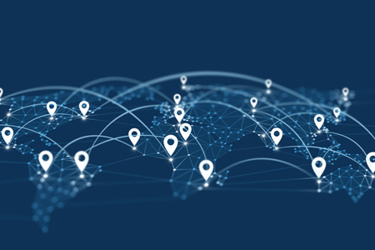
A crucial aspect of efficiently managing a clinical trial involves having a deep understanding of the trial's supply requirements and integrating flexibility into its supply and resupply processes. While it is challenging to make precise predictions for clinical trial supplies, there are often certain aligned assumptions that can serve as a foundation for estimating these requirements. In the last three decades, there has been a significant expansion in the use of statistical, mathematical, and technical analyses, with one notable approach being the application of Bayesian statistics.
Bayesian statistics is distinguished by its use of probability to represent uncertainty and its focus on updating beliefs based on new evidence. This methodology offers a systematic and principled framework for statistical inference and decision-making.
Consider a scenario involving a double-blind clinical trial for a rare disease, where two experimental treatments, referred to as Treatment A and Treatment B, are under evaluation. In this trial, a Bayesian adaptive design is employed to efficiently allocate resources, with a focus on incorporating ethical considerations and real-time patient data into treatment decisions. It is crucial to emphasize that preserving the double-blind nature of the trial while implementing a Bayesian adaptive design demands rigorous adherence to regulatory guidelines.
Planning Your Bayesian Approach
Prior to initiating this approach, it is crucial to ensure a shared understanding and a well-defined plan are in place. Without such alignment, supply planning and production requirements may become perplexing and add unnecessary complexity to the process. Here are a few key things to consider:
- Plan adaptations beforehand to avoid influencing the trial outcomes based on interim unblinded data analysis.
- Create two committees — a data monitoring committee (DMC) and a decision committee (DC). Separate these committees to ensure the unblinded data analysis remains isolated from the decision-making process. The DMC reviews unblinded data and provides recommendations to the DC.
- Develop blinded Bayesian methods for data analysis. These methods ensure that interim analysis and adaptations are conducted without compromising the blinding of treatment information.
- Implement stringent access controls to prevent unblinded individuals from accessing interim data or analysis results.
- Conduct simulations using historical data to demonstrate the impact of adaptations without unblinding.
- Define escalation and de-escalation rules based on observed outcomes, without disclosing treatment assignments.
At first glance, it seems like this approach demands a significant amount of effort. Is it truly justified? While it might appear overly complex in terms of supply management, it offers substantial benefits for the sponsoring entity, the receiving site, and, most importantly, the patient(s).
Reducing Waste and Preventing Shortages
The Bayesian approach offers the ability to implement dynamic treatment allocations, resulting in the reduction of investigational medicinal product (IMP) wastage and the efficient use of limited supplies. Frequently, there is a temptation to request the CMC and supply chain departments to produce, package, and label IMP in excess for the sake of convenience. However, instead of resorting to overproduction or imprecise allocation of costly pharmaceutical products, this approach facilitates optimized IMP allocation. This, in turn, helps curb the increased costs associated with overproduction and unused supplies.
By basing estimated demand on a systematic approach and allowing for adaptable adjustments, the supply chain can align itself with real-time patient data. This proactive strategy substantially reduces the risk of experiencing shortages and prevents the accumulation of excessive inventory. Moreover, beyond the primary tasks of manufacturing, packaging, and labeling, this approach yields advantages in downstream logistics. The continuous monitoring of IMP inventory and its consumption guarantees timely delivery to patients, thereby minimizing delays and enhancing the quality of patient care.
It is important to note there is a tendency to initially provide sites with excessive volumes of IMP and continue to flood them with an abundant supply. However, this practice not only drives up costs related to IMP storage and shipping charges but also exacerbates the problem of surplus inventory at sites responsible for dispensing IMP to patients. Many pharmacies prefer to keep their inventory in check due to the premium placed on space in most cases.
The Bayesian approach employs mathematical calculations to optimize the allocation of IMPs. This process involves calculating treatment probabilities using both prior information and real-time trial data, which requires collaborative effort from clinical operations and the supply chain, working with a carefully designed planning model.
AI and ML resources are being harnessed to support these adaptive approaches, including strategies to safeguard blinded information.
Conclusion
The use of optimization algorithms to dynamically allocate treatments, while simultaneously minimizing resource wastage and adhering to trial constraints, offers substantial benefits to sponsors, trial sites, and, most importantly, the patients involved in the study. From the sponsor's perspective, implementing an adaptive design can result in cost savings, expedited time to market, and improved odds of success. For clinical sites and hospitals, this approach streamlines procedures and optimizes the use of resources, facilitating increased participation in trials without compromising patient care. As for the patients, adaptive designs can offer greater opportunities to receive effective treatments, instilling a sense of hope and motivation to participate in clinical trials.
The convergence of Bayesian adaptive designs, AI, and the stringent requirements of double-blind trial designs represents an exciting frontier in medical research. By optimizing resources and upholding blinding principles, the industry is poised to revolutionize the evolution of clinical trials. These innovations span across various disease areas, from oncology to infectious diseases, ensuring that real-time data shapes treatment allocation while preserving the essential double-blind framework.
About The Author:
Christopher Ohms is a consultant at Ohms Consulting and is a San Francisco Bay Area native. He has held positions at Rigel Pharmaceuticals, Gilead Sciences, Patheon, Stanford School of Medicine, Pain Therapeutics, and ALZA.