Building And Applying A Configurable Clinical Study Model
By Jeffrey S. Handen, Ph.D., Yesh Ajgaonkar, and Neil Conroy, Grant Thornton LLP
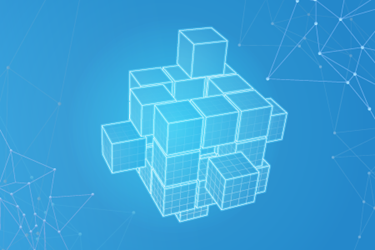
Last month we introduced an approach for the rapid assessment and prototyping of digital and other clinical trial technologies.1 Advances in technologies and digital innovations targeting the clinical research environment are evolving at dizzying rates, and the need to efficiently assess and implement opportunities is greater than ever. The challenge, then, becomes how to select those solutions that are not only the most efficacious but provide the largest ROI, i.e., separating the proverbial wheat from the chaff. The answer, as outlined previously,1 is rapid prototyping involving a three-step adaptive approach: building a configurable model, conducting virtual/conference room pilots, and interim data analysis. In this article, the first of a series of three, we will more fully explore the first step in the process – building a configurable clinical study model – or, process modeling – against which to measure potential efficiencies. Future articles will examine how to leverage virtual pilots and artificial intelligence (AI) in testing the model and evaluation and change management considerations in pilot implementations of ongoing GCP-compliant studies.
Lessons From Other Industries
Process modeling to drive performance improvement (PI) is the mainstay of many operational excellence (OE) activities in a range of industries. PI models are used to calibrate the output of a particular business process by comparing before and after modifications (in this case, applying a digital technology) that result in increased output, efficiency, effectiveness, and/or cost reduction.
This approach has been used quite successfully, and routinely, in the finance industry, for example, to represent the effect of a financial asset on a portfolio or an investment initiative on a business, in order to forecast cash flows and to make decisions on raising capital, acquisitions, risk aggregation, budgeting, forecasting, capacity planning, and allocations. Lloyd’s Banking Group, the largest retail and commercial bank in the U.K., is a prime example of a firm using process modeling, combining it with robotic process automation (RPA) to identify the steps in its secured lending business amenable to digitization – an exercise that resulted in significant critical path time savings.2
In the insurance world, PI models are used both for risk analysis and predictions based on risk profiles and factors. This is evident in the many vendors offering process and service modeling for the insurance industry, i.e., business process management (BPM), of which business process modeling and analysis are hallmarks. In the consumer goods industry, these models are used to optimize supply chains and improve performance in manufacturing operations.
In the aeronautics industry, the Boeing 777 was the first jetliner 100 percent digitally designed using the concepts of (virtual) process modeling.3 Boeing engineers were able to identify potential bottlenecks, establish lead times, and use modeling and simulation of modification of key steps to determine not only optimal product characteristics, but production schedules. This approach eliminated more than 3,000 assembly interfaces; reduced engineering change requests by 90 percent (6,000 to 600), cycle time for engineering change requests by 50 percent, and material rework by 90 percent; and improved assembly tolerances for fuselages by 50 times.
What all these seemingly disparate examples have in common is the use of process modeling to drive measurable improvements in time, cost, and/or quality of project execution in highly regulated environments with complex supply chains, where safety and quality are paramount.
A Modeled Clinical Study
Similarly, in the clinical trials industry, there is an increasing focus on improving time, cost, and quality when executing clinical studies. Additionally, with the advent of novel technologies and methodologies, such as wearables, AI, risk-based approaches, and more, the pharma industry is in a constant state of evaluating and implementing these approaches. Here, we present an activity-based approach based on best practices to evaluate such proposed improvement opportunities and solutions that looks at the individual activities that make up a clinical trial and the levers associated with those activities as the basis of the model. This allows for the differentiation of critical path levers that directly affect time – and thus, time to market (TTM) and non-critical path but value-added activities that directly affect out-of-pocket costs. The model defines the approximately 70 Level 2 process steps that make up a “typical” clinical study, from clinical development plan (CDP) planning to clinical study report (CSR) writing.
The model, which can be modified for nontypical studies or customized to add further levels of granularity as desired, assumes industry-typical ranges on all activities’ duration and work efforts, yet the configurability of the model allows a user to input company, phase, TA, or any such study-specific parameters, such as average study team FTE cost, number of sites, number of subjects, etc. It also accounts for potential sales of the product under development in order to capture revenue enhancement opportunities driven by impacting the levers of identified critical path activities.
The model allows the user to specify which activities are affected by the proposed (technology) change, i.e., the levers, the magnitude of the effect, and the “type” of effect. The type of effect can either be to the work effort expended for the activity (direct cost savings) or to the duration of that activity. For duration effects that are critical path activities as defined by the model or configured by the user, the corresponding increase in TTM translates directly to enhanced revenue, based on user-defined or industry average market projections. Once all the effects for each relevant activity have been entered, the model calculates individual benefits for the activity in question, as well as its contribution to efficiencies for study processes (e.g., study planning, study startup, enrollment, data management, statistical analysis, report writing, etc.) and the entire study as a whole.
Model Use And Output
This process modeling approach allows for quantifying the proposed effect of multiple technology (or other) improvements, both individually and according to their cumulative effect on the given study, on measurable value-added outcomes. Given the complex nature of many technology improvements and of clinical trials themselves, this approach is best implemented through a facilitated workshop or in a small-group setting with the appropriate subject-matter experts around the table to tailor the model for specificity.
Steps include the following:
- Provide study-specific inputs and assumptions where available, or default to industry-typical averages supplied as baseline assumptions in the model.
- In its simplest form, the user first identifies from a list of all process steps comprising a typical clinical study those with the potential to be impacted by the proposed digital (or other) change and those that are critical path activities, i.e., rate-limiting.
- Then, the user assigns work duration (in days) and work effort (in hours) to those process steps or accepts the model-assigned default values – the before values.
- Link vendor claims and/or hypothesized efficiencies to defined process steps and confirm impact on value-added activities.
- The user then assigns reductions in duration or effort due to direct postulated effects of the beneficial technology or to indirect positive effects on quality – the after values.
- Various scenarios can be modeled.
- Through the model output, the working group identifies the proposed technology improvements with the greatest potential ROI, either on direct out-of-pocket cost savings through reduced work effort or improved quality, or enhanced revenue opportunities through decreasing TTM.
The model can also be used at the portfolio level by configuring multiple studies that make up the portfolio, across phases and therapeutic areas, running the same improvement scenarios and quantifying the cumulative output.
Leveraging The Output
In addition to using the model outputs to perform a sensitivity analysis to identify process steps that would return the most bang for the buck, teams can conduct virtual conference room pilots using pragmatic AI approaches. Using available data and defined KPIs, it’s possible to analyze multiple digital interventions/scenarios, or digital clinical trial arms, for the optimal combination in a minimal viable product (MVP) development technique in 30 days. We’ll explore these scenarios in next month’s article in this series.
References:
- “Assessing And Implementing Clinical Trial Technologies: A 3-Step, Adaptive Approach”, J.S. Handen, Clinical Leader, March 5, 2019
- “Before Automating Your Company’s Processes, Find Ways to Improve Them”, T.H. Davenport and D. Brain, Harvard Business review, June 13, 2018
- Boeing Frontiers Magazine, July 2009
About The Authors:
Jeffrey S. Handen, Ph.D., is a director in the Life Sciences practice at Grant Thornton LLP and leader in R&D Transformation. He has published in multiple peer-reviewed and business journals, presented at numerous industry conferences and scientific meetings as an invited speaker, and served as past editor in chief of the Industrialization of Drug Discovery and Re-inventing Clinical Development compendiums. Handen has more than 20 years’ experience in pharmaceutical and biotechnology R&D, process re-engineering, and systems and process implementation. He is responsible for overseeing business process improvement and solution architecting for optimizing both clinical development and discovery.
Yesh Ajgaonkar is a seasoned program management practitioner and a strategic thinker with tactical execution capabilities who brings critical thinking and intellectual rigor to help clients successfully deliver on large complex business transformations. He is well-versed in program management, PMO stand-up, portfolio and program health assessments, delivery of innovative business solutions, ERP implementations, post-M&A integrations, divestitures, process improvement, and business-led transformations using Agile and Waterfall methodologies across the pharma, finance, insurance, consumer, media, technology and manufacturing sectors.
Neil Conroy is a senior process improvement leader with more than 20 years of cross-industry operational excellence experience. He has designed and led several organization-wide improvement programs in complex and challenging manufacturing and R&D environments and has deep experience in Lean thinking, Six Sigma and organizational change management.