Combining Data Science & RWE For Better Clinical Outcomes For Immunological Diseases
By Hemanth Kanakamedala, senior director, The Janssen Pharmaceutical Companies of Johnson & Johnson
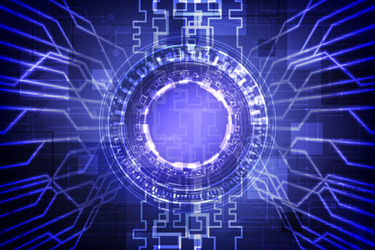
As we continue to push ourselves to better understand immune-mediated inflammatory diseases (IMIDs) and develop new solutions for patients’ unmet treatment needs – particularly for patients with rare disease or those with prevalent but difficult-to-treat diseases like psoriatic arthritis (PsA), rheumatoid arthritis (RA), and inflammatory bowel disease (IBD), which includes Crohn’s disease (CD) and ulcerative colitis (UC) – Janssen’s Immunology and R&D Data Science teams are utilizing real-world data (RWD) to drive impact across the drug development life cycle.
We apply machine learning and artificial intelligence (AI) on real-world data (RWD) – including administrative claims, electronic health records, laboratory data, disease registries – to generate evidence on diagnosis, prognosis, and etiology. This is critical for providing relevant context for the appropriate use of new and existing therapies. Such real-world evidence (RWE) gives us a deeper awareness of patients’ medical needs, their journeys, gaps in current treatment options, and a vision toward new areas of research. Some specific areas of impact are highlighted below.
RWD Is Proving Invaluable For The Identification And Development Of Biomarkers
RWD – powered by data science – helps us better understand the diseases we’re tackling, and the patients impacted by them. At Janssen, we are using RWD to create detailed phenotypic profiles, which provide a comprehensive analysis of clinical characteristics of patients and of their immunological diseases. Not only is this an evaluation of what is documented in patients’ medical charts, but also their labs and/or imaging. This information is helping us develop more precise disease classification systems using AI and NLP, which is bringing us a step closer in the path toward precision medicine.1 This is helping us identify novel disease biomarkers, find and clinically advance promising compounds that can target them.
Use Of Data Science And RWD To Design Smarter, More Efficient, And More Representative Clinical Trials
Knowledge of a disease’s natural history is critical for drug development. We utilize RWD to inform the design of our interventional studies, including inclusion/exclusion criteria, diagnostic criteria, adequate follow-up, assumptions to power the study, and other design components.
RWD is especially critical to drug development in ultra-rare immunological diseases such as hemolytic disease of the fetus and newborn (HDFN), a condition that occurs when maternal red blood cells or blood group antibodies cross the placenta during pregnancy and cause fetal red cell destruction. Randomized controlled trials are often infeasible and unethical in such populations. Consequently, patient populations like these have been underserved historically by traditional clinical development programs. We enroll such patients in single arm studies with real-world external control arms using rigorous methods to control for confounding.
Fundamental to the successful treatment of immunologic disease is recruiting patients who reflect the same characteristics as those in the real-world for our studies. Diversity, equity, and inclusion embedded into study inclusion/exclusion criteria and active patient recruitment is critically important to serving the needs of all patients and enables our ability to improve access to innovative therapies. Without inclusion of all patient subpopulations with immunologic disease, it is difficult for researchers to have a thorough understanding of disease progression and response to therapy in important patient subgroups.
This is particularly important in under-represented and understudied populations. We continually ask ourselves if our clinical trial sites are in the right places and if we are making our clinical trials accessible to all populations of patients with immunologic disease. With this in mind, Janssen is applying AI and machine learning to RWD to help identify where pockets of patients with rare or difficult to diagnose diseases are and help inform the placement of study sites – with the goal of enabling communities of patients who may not have participated in a clinical trial in the past to enroll in a study.
We know that diseases and drugs may impact people differently based on their race and ethnicity, so the alignment of clinical trial enrollment with patient population demographics is key. Simple yet impactful decisions, such as making sure clinical trial sites are located in accessible places within historically underserved communities, make a big difference in our ability to reach a representative population to ensure we are learning everything about how our new therapies are addressing the unmet medical need across all races, ethnicities, and genders.
Integrating Digital, Real-World Endpoints Into Trials
Understanding how improvement would be seen and measured in a real-world clinical setting is key to advancing outcomes among patients with diseases like CD and other IMIDs. Important RWD such as endoscopy videos and histology slides – using computer vision algorithms to measure disease severity – are built into our CD clinical trials and create a bridge between standard clinical trial outcomes and measures valued in a real-world clinical setting.
An RWE approach enables collection of more comprehensive data so that we may contextualize randomized controlled trial (RCT) outcomes against questions about diagnosis, prognosis, and disease etiology. Answers to these questions are also critical for articulating the value of changing a health outcome.
Comparative Effectiveness Research After Product Launch
Tokenized RWE is also helping us generate evidence on healthcare resource utilization and other real-world outcomes during and after the conclusion of our trials. After launch, we monitor the efficacy and safety of our products through the analysis of RWD. To mitigate the limitations of traditional case-control designs, we emulate pragmatic RCTs of our approved treatments2. This type of comparative evidence generation is critical for informing real-world effectiveness of our therapies.
RWE is increasingly playing a critical role across the product lifecycle in our immunology trials. To learn more about our work, visit our Immunology and R&D Data Science sites.
References
- Weng, C., et al. Deep phenotyping: Embracing complexity and temporality-Towards scalability, portability, and interoperability. J Biomed Inform. 2020;105:103433. https://doi.org/10.1016/j.jbi.2020.103433
- Miguel A. Hernán, James M. Robins. Using Big Data to Emulate a Target Trial When a Randomized Trial Is Not Available, American Journal of Epidemiology, Volume 183, Issue 8, 15 April 2016, Pages 758–764, https://doi.org/10.1093/aje/kwv254
About The Author:
Hemanth Kanakamedala is senior director, immunology within Janssen R&D Data Sciences. His expertise lies in drawing causal inference using observational, non-randomized data. His work is focused on externally controlled interventional trials, emulating randomized experiments using observational data, and integrating patient-centric digital health endpoints in trials. Prior to joining Janssen, Kanakamedala spent 10 years supporting the design and execution of phase 1–3 randomized controlled trials and non-interventional studies. He holds a degree in mathematics and statistics from the University of Massachusetts, Amherst.