Data Overload: Determining Which Trial Data To Collect
By Rashida Rampurawala, study data lead, Sanofi
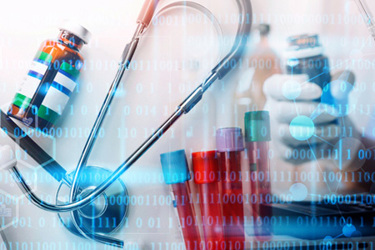
Clinical trial participants provide a variety of routinely collected clinical data. By analyzing data regarding the consequences of the treatment under investigation, researchers are better able to determine if it is safe and/or effective. However, a substantial amount of participant data is available within a clinical trial — and not all of it is required for trial analysis.
Let us look at recent statistics pertaining to clinical trials data collection. The amount of data collected has expanded due to growing regulations and requests from sponsor companies. On top of mounting clinical data, more than half of the outcome data gathered for Phase 2 and 3 studies is secondary, exploratory, or auxiliary; the remaining, of course, is primary. In fact, based on research by KA Getz et al, in just one drug/dietary supplement trial, only 18,124 of the 137,008 data items obtained were deemed essential for targeted risk-based monitoring (RBM) — barely 13% of the total data collected.1
The abundance of data gathered from clinical sources to patient-worn sensors can assist researchers in selecting suitable trial participants, identifying potential side effects, and even giving regulators information to support the approval of new indications. However, the methods for data collection, processing, and quality control take time and effort for each data point. More data typically means more work for trial staff and participants, as well as rising costs. To ensure limited trial resources and participant goodwill are used appropriately, it is helpful to know what kind and how much data is being collected. Therefore, we need to identify and define necessary data, supportive data, and “nice to have” data.
What Data Must Be Collected?
Making sure the data obtained are adequate to address the trial's purpose while not being too cumbersome to jeopardize the trial's viability is an underappreciated problem in conducting clinical trials. It’s reasonable to anticipate that the primary outcome of a clinical trial will receive a sizable portion of the data collection because it is the measurement used to assess the effectiveness and safety of the intervention.
Yet, instream data collection is only one part of data collection process; it is equally imperative to understand why and how the data is reported for analysis. Case report forms (CRFs) support recorded data from other sources, such as the electronic health record (EHR) and paper records, as well as primary (real-time) data collection. The difference between using EHRs and gathering research data is that the latter meticulously and maximally structures a subset of patient parameters — the variables of the research protocol — while narrative text is de-emphasized except to record unexpected information.
Conventions and some best practices exist, but there are currently no global standards for CRF design, though Clinical Data Interchange Guidelines Consortium (CDISC) has introduced guidelines that primarily focus on regulated trials. These suggestions, while helpful in general areas like drug safety, do not, however, deal with more extensive problems in clinical research, such as observational research, genetic studies, and studies that use patient-reported experience as a primary study endpoint.
Hence, we see requests from study teams to include data types/fields that may not need to be captured at the EDC level and instead can be managed at the study data tabulation model (SDTM) or biostats programming level by combining a set of data captured with the EDC. The essence of data collection within EDC should categorically focus on necessary endpoints as per protocol and for certain maneuvering within the EDC in order to collect specific form within specific cohorts or arms within the study. The way a data item pertinent to a research procedure is divided into distinct CRFs might vary depending on the study design and is frequently based on factors other than logical grouping. For instance, if the items are not too numerous, one may choose to designate a single CRF to capture all of them in a one-time data collection. However, in a longitudinal study, things that are only recorded once at the beginning of the study are kept apart from items that are sampled repeatedly throughout several visits in a CRF.
For a data manager (DM), liaising with the cross-functional teams like clinical and biostatistics in the nascent stages of protocol development helps in understanding the schema and significance of data to be collected within a trial. From the conception of the protocol, it is imperative to collect data which impacts analysis and to avoid “nice to have’s, as ultimately the trial design has to be patient centric. While designing the eCRF, there is a tendency to copy eCRF design from an array of an existing trial. This is mainly done to optimize efforts and time but may lead to unaccounted risks at a later stage of the trial. Therefore, understanding the science behind the data being collected helps with an efficient eCRF design and data collection tool that only collects the right kind and amount of data.
Questions To Ask Before Introducing Data Standardization
When a data standardization approach is taken to collect data within an eCRF, it can also inspire other cross-functional teams to collect what is required and categorizing the data as primary, secondary, or auxiliary. Businesses rely on operations that are effective, and one of the most crucial components is preserving structured data across several systems. It might be difficult to standardize data across departments in or across an entire organization. The truth is that every department has access to the information they require to complete their tasks without having to worry about learning new formats, and data integrity problems can be avoided when there are clear, consistent data standards in place.
When assessing data fields throughout the data standardization process, many things need to be determined. It is beneficial to initially identify all potential data entry sites and assess their viability in order to streamline the procedure.
When evaluating data collection schema within a trial, some things to keep in mind are:
- What is the data source, and is s the information trustworthy and correct?
- How readily can the data be transformed into the necessary format?
- How much data is there, and is it manageable?
- Are the data entry fields and form simple to use and well defined?
Eliminating any duplicate data points is one of the first steps to identify what data is needed . Data that is identical to another data point in the same data set is referred to as duplicate data. Data standardization can start when your data has been cleaned.
Standardization Can Keep Out Unwanted Data
Data capture standards can help with interoperability, data quality and consistency, efficient creation and implementation of new studies, and element reuse. Clinical research is protocol-centric, so chances for shared standards at levels above individual items are more constrained than for standards at the item level. However, disease-specific CRF standardization initiatives have helped identify standard data item pools within specialized research and professional groups and, as a result, have secured research efficiencies within respective application domains.
Standardization efforts to create good procedures and workflow for CRF and CRF section development, as well as data collection and validation, are of more urgent and widespread value. In such development, terminology usage for facilitating semantic interoperability should be emphasized. The structure and content of individual CRFs/sections can be left flexible to facilitate adaptation to specific protocol requirements as good CRF design principles and community participation become standard practices in clinical research. More and more sponsor companies are working toward developing data standards within the organization to achieve harmonization in terms of data collection as this has a direct impact on eCRF design, SDTM programming, and then how biostatisticians handle and analyze the collected data. At a functional level, standardization also helps achieve the milestone targets and align the cross-functional teams with the same goal.
References:
- Getz KA, Stergiopoulos S, Marlborough M, Whitehill J, Curran M, Kaitin KI. Am J Ther. 2015;22:117–24), (Fougerou-Leurent C, Laviolle B, Tual C, et al. Br J Clin Pharmacol. 2019;85:2784–92 10.1111/bcp.14108)
About The Author:
Rashida Rampurawala has 15-plus years of CDM experience and is currently working as a study data lead at Sanofi, where she is responsible for oversight of projects/portfolios and people management. She started her career in 2010 in UK and then continued in India since 2012 and has worked across CROs and pharma companies. She pursued a master’s in biomedical sciences from UEL in U.K. and has an executive MBA from XLRI in India. She has presented at various conferences held by SCDM, DIA, ISCR, PHUSE, and conducted RBM workshops at DIA and ISCR. She is a data visualization enthusiast. She is a part of the SCDM author group, which is updating the GCDMP chapters for CCDM certification.