Evidence Synthesis: Reducing The Guesswork Of Clinical Trial Design
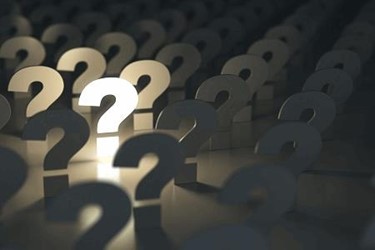
By Ross Maclean, M.D., and Jeroen P. Jansen, Ph.D., Precision Health Economics
One of the biggest challenges to the success of new drugs is that approval and uptake rely heavily on the evidence collected during development, yet decisions on the design of clinical trials occur long before much is known about a drug or its effects. The available evidence for existing and potential competing interventions is expanding constantly, raising the stakes for efficient and appropriate study design even further.
In the past decade, drug manufacturers have faced evidentiary demands from health technology assessment (HTA) agencies, and the convention has been to “do a meta-analysis” just before launch — to generate insights into how the new drug compares to the available alternatives. Given this context, meta-analysis methods have developed rapidly, and the scientific discipline of evidence synthesis has blossomed. Evidence synthesis relies on the principle that when we consider the findings of multiple studies simultaneously, we have a lot more information to inform decisions than when we interpret the findings of each study individually.
One specific and powerful evidence synthesis technique is network meta-analysis (NMA). A collection of randomized controlled trials informing on several treatments constitutes a network of evidence, in which each trial directly compares a subset of — though not necessarily all — treatments of interest. Such a collection of trials can be synthesized by means of an NMA, thereby providing relative treatment effect estimates between all treatments of interest, whether they were part of a direct head-to-head comparison or not.
The question arises: How can manufacturers take advantage of the great potential of evidence synthesis, and NMA in particular, for drug development decisions? Or, to put it more bluntly, how do you know where you’re going if you don’t know where you are (see Figure 1)?
How The Evidence Synthesis Process Works
Suppose your new “developmental asset” has promising Phase 2 data, and the pressure is on to move forward with Phase 3. The current standard of care is a novel branded agent in a different class than your drug, and it recently usurped that position from another brand from a third class. Trailing in third place is a long-standing drug that is now generic. With finite resources and the need to prioritize your Phase 3 trials, which drug is the best comparator, and what should be your primary trial endpoint?
A systematic literature review (SLR) will identify all the relevant evidence regarding the treatment effects of current and potential future competing interventions relevant to your development compound. This process involves searching through publicly available databases for peer-reviewed scientific papers describing the relevant studies, and selecting studies based on predefined criteria. Then, two or more researchers should independently read each paper and abstract for information regarding study design, population characteristics, and efficacy and safety. Potential endpoints of interest can range from laboratory “surrogates” to clinical outcomes, and can include patient-reported (quality-of-life) outcomes, safety data, and the increasingly popular measures of caregiver burden and workplace productivity. A database is necessary to store and validate the abstracted data and supplement it with relevant in-house sources (e.g., Phase 2 results).
Next, with the repository of relevant data in place, a robust synthesis of the data using NMA techniques and other advanced statistical models allows you to answer a variety of questions. For example:
- What are the relative treatment effects between all the available treatments regarding outcomes of interest?
- Do these treatment effects differ by follow-up time?
- Do treatment effects differ according to differences in study inclusion criteria?
- What is the relationship between treatment effects in terms of surrogate endpoints and hard endpoints?
This knowledge informs insights on Phase 3 decision-making, including endpoint choices, choice of comparator, drug-effect sizes and confidence intervals, sample-size calculations, and even safety signals.
But why perform the SLR-NMA just once when the data is changing with every scientific conference and competitor data release? A dynamic or cumulative NMA allows you to update your comparison of the different established and emerging treatments as often as you wish, such as by following the release of pivotal trial data from a competitor or the results of an interim analysis from your own trial.
Depending on the available data, a predictive NMA — where the anticipated endpoints (and other trial characteristics) for the yet-to-be-conducted study are modeled — can also be performed. Predictive NMAs can reassure you that, if successful, your trial will answer the question, “Of all the drugs, which one is the best?” — or inform a shift in your Phase 3 priorities and plans.
Wide-Ranging Benefits
Following are some of the benefits of evidence synthesis for a variety of stakeholders:
- As discussed, an NMA can help clinical development with trial design.
- For your pharmacoepidemiology and pharmacovigilance, a meta-analysis of safety data across all drugs in the class will inform the benchmark rates for safety signals that arise from spontaneous reporting, risk evaluation and mitigation strategies (REMS), and other safety monitoring efforts.
- For access, pricing, and reimbursement, authorities will demand to know the relative efficacy across all treatments available, not just the ones studied in the registrational program — an NMA will provide this critical evidence.
- For local market affiliates, the NMA can be adapted to the drugs available in each market, providing the relevant information for reimbursement decisions.
- In a dynamic and competitive therapeutic area with multiple competing products, the NMA will indicate how each drug compares on efficacy, safety, and patient-reported and other outcomes — and can be updated each time a competitor produces new data.
- For health economics and outcomes research (HEOR), an NMA is the starting point for understanding the comparative effectiveness of competing treatments; it also serves as the foundation for cost-effectiveness modeling.
- For the C-suite, an NMA will help them determine the potential meaning and impact of new data from competitors.
Like it or not, you will be meta-analyzed! Rather than taking a wait-and-see approach, or perform only an evidence synthesis to support HTA submissions once your Phase 3 program is completed, it makes sound business sense to integrate these kinds of studies into the early stages of drug development. Given the great potential for reward, combined with the high risk of drug development, it makes sense to base decisions on evidence as much as possible. NMAs can enable you optimize the design of your Phase 3 trial for regulatory purposes, as well as for market access.
About The Authors:
Ross Maclean, M.D., is SVP and head of medical at Precision Health Economics (PHE). He has more than 20 years of progressive experience in health services and outcomes research, health economics, health system design, health policy, and market access. With over a decade spent in the biopharmaceutical industry, Maclean gained wide exposure to value demonstration as head of U.S. HEOR for Bristol-Myers Squibb (BMS).
Jeroen P. Jansen, Ph.D., is VP and chief scientist for evidence synthesis and decision modeling at Precision Health Economics. He was a founding partner of Redwood Outcomes, which is now part of PHE. Jansen has 15 years of experience with meta-analysis, network meta-analysis, cost-effectiveness modeling, and health technology assessment submissions. He is trained as an epidemiologist. Jansen helped lead the development of network meta-analysis methods and guidelines. He has published many papers related to epidemiology, evidence synthesis, and economic evaluations, and was an associate editor of the Research Synthesis Methods journal.