How To Avoid Common AI & ML Mistakes In Clinical Trial Development & Recruitment
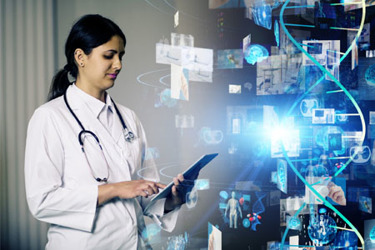
Over the last decade, bringing new drugs and compounds to market has become increasingly expensive. Considering that up to 90% of drug candidates never make it to market, the biotech and pharma industries have made significant investments in new technologies to increase the chances of approval.
Enter artificial intelligence (AI) and machine learning (ML). Though often associated with number crunching and broad-scope data analysis, many life science decision-makers are using artificial intelligence and machine learning to select trial sites and/or recruit patients. Using these technologies can reduce the number of trial sites and help you enroll the required sample size. But these results aren’t guaranteed. To truly reap the rewards, it’s crucial to avoid common pitfalls.
Take a closer look at four of the most common mistakes sponsors make when implementing AI or ML into the clinical trial development process.
Get unlimited access to:
Enter your credentials below to log in. Not yet a member of Clinical Leader? Subscribe today.