How To Balance Data Privacy And Sharing: A New Methodology For Clinical Data Reuse
By Boris Grimm and Jeppe G. Manuel, TransCelerate BioPharma Inc.
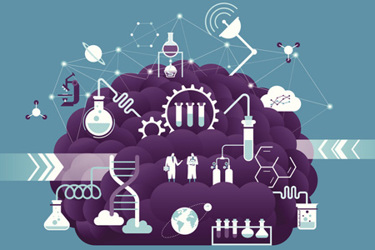
Clinical research has previously struggled to develop data privacy and data sharing operations. On one side are the laws, regulations, and ethical considerations that necessitate protecting personal information about individual research participants. On the other side is a desire to reuse high-quality participant-level clinical trial data for further research to improve healthcare, thus potentially minimizing future participant burdens.
Until now, most pharmaceutical companies have had to build their own approaches to address both data privacy and data utility. Available principles frameworks1 offer a conceptual understanding of how to proceed but lack concrete methodologies.
The TransCelerate consortium and its preclinical subsidiary, BioCelerate, aim to address that gap with a new methodology titled Clinical Data Sharing: A Proposed Methodology to Enable Data Privacy while Improving Secondary Use.2 The result of a three-year collaboration involving members from more than 20 global sponsors and substantive comments from industry stakeholders, this “cookbook” describes how sponsors can improve the sharing and reuse of clinical data for secondary research. The best practices it contains are intended to help speed scientific insights and reduce burdens on research participants by enabling the industry to:
- increase transparency around the privacy safeguards used on clinical trial data,
- increase data utility for the reuse of existing clinical trial data, and
- protect patient privacy.
Recommendation Snapshots
In balancing data privacy and utility, researchers focus intently on protecting their clinical trial participants and the data. For optimal data sharing, it is essential to understand what steps have been applied to participants’ data to anonymize it. Methodology and documentation are necessary to secure participants’ continued privacy while ensuring that the anonymized data are appropriate for secondary research purposes.
Therefore, the new methodology outlines concrete steps designed to improve transparency and reduce data privacy variability to better support the reuse of participant-level clinical trial data. Because it is intended to augment current strategies, it delivers “recommended” and “compatible” approaches for modifying 12 common clinical data set variables and provides key considerations regarding data utility and data privacy for each variable.
Here is a brief synopsis of the recommendations for the 12 variables:
- Unique identifiers. Unique identifiers include data values that could be used to identify a study participant alone (direct identifiers) or in combination (indirect identifiers). The methodology recommends replacing the original data values with scrambled values of the same length, type, and format for direct identifiers. For indirect identifiers, the methodology recommends that researchers should assess on a study-by-study basis whether transformation is needed. If possible, an indirect identifier should be retained or scrambled rather than redacted or suppressed.
- Dates. If a data set includes actual dates related to individuals, the methodology recommends transforming them using the Date Offset method. This involves adding or subtracting a defined number of days from the original date. The methodology also suggests applying a randomly generated number of days within parameters appropriate to the study.
- Verbatim text/free text. Free text data fields may contain valuable insights but may be difficult to analyze. The methodology recommends redacting most free-text fields, with non-blank values used to clarify that the variable field was not originally blank. The string “—redacted—" should replace any fields that require modification.
- Variable banding. Many indirect identifiers — such as age, height, and weight — have high scientific utility but also present significant re-identification risks. Banding techniques allow researchers to aggregate continuous variables (e.g., age) into groups that reduce the likelihood of re-identification. The methodology recommends generating independent bands on each relevant variable within a data set (single-dimensional, flexible banding) and keeping bands as small as possible to maximize data utility.
- Patient demographics. Demographic data that describe a study’s population — such as sex, race, and ethnicity — are crucial to the quality of research analysis. Consequently, the methodology recommends preserving as much information as possible about sex, race, and ethnicity. However, it cautions that risk must be assessed in conjunction with other identifiers. When demographic data must be removed, the methodology suggests including a rationale for the removal in data-sharing documentation. 3
- Low-frequency data. This refers to data that applies to a very small cell or group size and thus increases the risk of participant re-identification. The methodology recommends assessing variables with low frequencies and redacting those values that pose a significant risk for re-identification, using PHUSE De-identification Standard for SDTM 3.2 as a guide for making data adjustments.4 It also notes that supporting documentation should explain the removal or redaction of low-frequency events.
- Sensitive information. Sensitive information is highly personal information that could harm a participant if disclosed. Examples include data about alcohol or drug use, mental health conditions, or conditions such as HIV/AIDS. However, it is essential to know the context of the clinical study to determine whether data in a specific data set is considered sensitive information. As such, the methodology recommends that 1) pharmaceutical companies have a policy to determine which variables should be classified as sensitive information, and 2) sensitive information should be redacted across all variables, with the redaction explained in supporting documentation.
- Adverse events & medical history. Data about medical history and adverse events are pivotal to therapeutic safety and efficacy assessments. However, the methodology recommends redacting any verbatim adverse event terms and replacing them with the appropriate MedDRA-coded term. It further recommends using all five levels of the MedDRA dictionary and sharing information on the version being used in the supporting documentation. Any adverse events or rare medical history data that pose a privacy risk should be redacted, with a description of the redaction steps included in supporting documentation.
- Concomitant medications. Clearly and consistently identifying the medications taken by study participants is essential to data utility. The World Health Organization (WHO) has created a medication classification structure known as the Anatomical Therapeutic Chemical (ATC) Classification to help enable consistency in medication communication. Therefore, the methodology recommends that verbatim medication terms be removed and replaced with the corresponding WHO ATC drug code. It further suggests using the complete WHO ATC code (all five levels of detail) for the data set and sharing the version of the WHO ATC classification being used.
- Geographic location. The methodology recommends keeping original country information whenever possible or remaining as close as possible to the data collected. Aggregating data with other countries on the same continent is only advised for studies involving single-site countries and/or countries with very few participants. It further suggests using the United Nations Statistics Division’s (UNSD) proposed methodology, the ISO-alpha3, and M49 (UNSD — Methodology) standards for generalizing geographic information.5
- Records of deceased participants. Data privacy regulations may still apply if a research participant dies during a clinical trial or the post-treatment phase. So, the methodology recommends treating the data of deceased participants in the same manner as the data of living participants. Specifically, it states, “A participant’s death should have no special impact on the level of anonymization needed for that individual…”.
- Information collected under copyright licenses. There are many variables involved in clinical trial data collected under copyright. However, to maximize data utility, the methodology recommends sharing as many variables or data as possible within the limits of applicable licenses. Supporting documentation should include applicable license versions and issue numbers, any license limitations relevant to secondary research teams, and the version/issue number of any questionnaires used.
Collaborative Solutions To Common Challenges
In addition to offering details about each variable’s recommended approach, the new methodology provides insights into important considerations and compatible approaches. Insights into evolving areas of data privacy — including data derived from genomic information — are also touched upon.
As part of the recommendation for supporting documentation, a Data Transparency Checklist is introduced via the methodology, which will prove equally valuable for many organizations. Those focused on collecting and analyzing clinical data may not always consider metadata regarding data transformation, but appropriate reuse depends highly on it. Thus, the transparency checklist is intended to provide data recipients with a great level of insight into how the clinical data has been transformed and thus increase data utility.
Throughout our work on the Privacy Methodology for Data Sharing Initiative,6 it has been gratifying to collaborate with colleagues from many companies and disciplines on solutions to common challenges. We’ve come to realize that drug development organizations across the globe are dealing with the same problems and issues in many ways. Companies may look to the new methodology to better balance increasing data reuse and maintaining data privacy; we hope it will benefit the wider data-sharing community.
References:
- Manuel, JG. 6 Key Principles To Guide The Compatible Reuse Of Clinical Data. Clinical Leader. 21 December 2021. https://www.clinicalleader.com/doc/key-principles-to-guide-the-compatible-reuse-of-clinical-data-0001
- TransCelerate BioPharma Inc. Clinical Data Sharing: A Proposed Methodology to Enable Data Privacy while Improving Secondary Use. Accessed 30 August 2023. https://www.transceleratebiopharmainc.com/wp-content/uploads/2023/08/FINAL-Privacy-Methodology-Revision-August-25.pdf
- TransCelerate BioPharma Inc. Transparency Checklist Template. Accessed 30 August 2023. Standalone-Transparency-Checklist-August-2023.docx (live.com)
- PHUSE Working Group. De-Identification Standard for SDTM 3.2 Version 1.0. May 20, 2015. Accessed 30 August 2023. https://phuse.s3.eu-central-1.amazonaws.com/Deliverables/Data+Transparency/De-identification+Standard+for+SDTM+3.2+Version+1.0.xls
- United Nations. Department of Economic and Social Affairs. Statistics Division. Methodology. Standard Country or Area Codes for Statistical Use (M49). Accessed 30 August 2023. https://unstats.un.org/unsd/methodology/m49/
- TransCelerate BioPharma Inc. Privacy Methodology for Data Sharing. Accessed 30 August 2023. https://www.transceleratebiopharmainc.com/initiatives/privacy-methodology-for-data-sharing-2/
About The Authors:
Boris Grimm is the head of (chapter) biostatistics & data science operations at Boehringer Ingelheim. Boris joined Boehringer Ingelheim’s department for Biostatistics and Data Science in 2013, where he took an active role in developing first-wave de-identification processes for BI’s Data Transparency Initiative and putting them into production. As a senior statistical programmer, he has provided many contributions to critical trial and project activities across various therapeutic areas and submissions.
In 2020, Boris took over the role as head of global clinical trial disclosure & data transparency. At the beginning of 2022, he stepped into the role of a chapter head and capability owner of clinical trial transparency and data sharing. He also acts as a co-chair for the PHUSE SDE in the Germany/EMEA region.
Jeppe G. Manuel is principal R&D data privacy specialist at Novo Nordisk. In his role, he is responsible for driving and maturing the global research organization’s data protection framework. He focuses on development, clinical- and health-related data, and ensuring legitimate compatible use of research participants’ data while maintaining high data ethics and a solid legal foundation.
Boris and Jeppe presented the approach outlined in TransCelerate’s Privacy Methodology for Cross-Industry Clinical Data Reuse at the PHUSE Data Transparency Summer Event 2023.