Improve Site Selection And Identify Your Ideal Patient With AI Insights
By Life Science Connect Editorial Staff
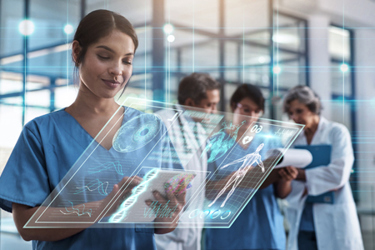
The question of using artificial intelligence (AI) and machine learning (ML) in clinical trials is no longer one of if we or should we, it’s how and when can we? The answer to that varies from pharma company to pharma company and depends on the needs and goals of a given trial. While data analysis is perhaps the most obvious way to implement AI and ML into a trial, that’s just the tip of the iceberg in terms of how this technology can help clinicians design effective trials and produce more successful outcomes.
In a recent Clinical Leader Live event, Chief Editor Dan Schell sat down with Head of Reporting Analytics and Insights at Astellas Pharma Mo Ali; CEO of IGC Pharma Ram Mukunda; and Assistant Professor for Operations Research, Machine Learning, and AI in Healthcare at Harvard Business School Michael Lingzhi Li. This expert panel dug into the data-backed benefits of using AI to help with site and patient selection while sharing their thoughts on how to expand AI offerings in pharma even further.
How Is AI Being Used In Clinical Trials?
According to a viewer poll during the live event, data analysis is still the primary way many pharma companies are using AI in their trials, if they’re using it at all. The key to implementing this technology to your trial’s advantage is recognizing where it can be injected to reduce the load on key staff resources, allowing them to use their time more tactically. Ali provides an example: “A company like Pfizer might have 1.5 million files to archive each year, maybe more. Each of those files may take 15 minutes [to archive manually]. With AI technology, you cut that time down to seconds. For the sponsor, that’s a significant plus because resources [typically] spending that time and effort can be redirected to activities that require more strategic thinking and application.”
Beyond reducing the manual workload, AI can also be used to help diagnose patterns and respond to them. That might include training AI to recognize repeated words in site searches and, from there, tasking a trial training team with reaching out directly to the site to better address their questions and concerns. In Mukunda’s case, his team leveraged AI to track data patterns for their Phase 2b trial: “We created an internal software within a relational database to put our data points in. We took this data and trained a transformer model to cluster patients and determine those that responded best to the intervention as well as which input variables are important. Phase 1 data helped us with selections in Phase 2. The roughly 3,000 data points helped us cluster and stratify patients and look for important variables and biomarkers.”
Mukunda’s trial is seeking to treat agitation in Alzheimer’s patients, a neuropsychiatric symptom of the disease. To help interpret the vast amount of data gathered, they partnered with The Center for Research and Formation in Artificial Intelligence at the University of the Andes, led by AI expert and academic Pablo Arbeláez. This partnership has provided IGC with access to the university’s existing hardware, rather than forcing them to build their own, an expensive and time-consuming task. The academic team also has helped to strategically design models and teach clinicians how to interpret them.
Beyond the possibilities for data analysis, AI also can be leveraged to speed up the initial design and development phase, which is typically an expensive and time-consuming process. Li, who worked with Janssen to use AI in a COVID vaccine trial, details the potential impact: “Historically, it may take an organization 35 weeks for startup activities to begin. If pharma companies focus on developing AI tools that [can inform] patient population, site selection, and probability of success, they'll cut this time significantly. You’re not only starting up faster with better insight and intelligence, but you’re also likely going to finish faster because the intelligence [helps ensure] a high likelihood of success.”
What Does Successfully Using AI In ClinOps Look Like?
According to Li, when he was working on the Janssen COVID trial, “There was some skepticism when we recommended Brazil, Peru, Russia, and South Africa [as trial sites]. However, our model showed that if we did clinical trials there, we'd be able to get a representative sample faster because of how the pandemic was projected to spread.”
Ultimately, Li believes they were able to reduce skepticism due to the transparency of their AI model, i.e., it’s not a black box like others. His team could share why specific parameters existed and how the model could respond to government interventions or virus variants, helping to build confidence for their collaborators. Beyond that, there was open communication between stakeholders. Li and his team asked Janssen, “What are your concerns with the model, and how can we solve them?”
The results speak for themselves. Thanks to the assistance of AI, Janssen was able to conduct the trial with 15,000 fewer patients and accelerate the timeline by eight weeks. Furthermore, they were able to deliver the vaccines to those in developing countries much faster and save a significant amount of money — and likely lives. “It is one of my proudest projects. We were able to demonstrate that AI has a benefit to patient care and cost through accelerating the trial process.”
How Do We Improve AI Accessibility In Drug Development?
Despite the compelling possibilities of AI for trial design and execution, it has only recently started to become a more accessible technology for those who don’t have Ph.D.s in the AI or ML space. To ensure the technology becomes both useful and usable for clinicians, they’ll need a baseline understanding of how GenAI and ML work as well as strong partnerships with AI academics and consultants. Li recommends that those interested in improving their AI literacy build their knowledge of statistical theory: “They need to understand how the garbage is being processed to know if its garbage or not.”
Beyond that, AI is expensive to implement, both in terms of hardware and the relatively scarce experts who help design AI models. To help overcome these obstacles, pharma companies should consider forming partnerships with academic cohorts that have access to and expertise around existing AI hardware. These collaborations are beneficial to both clinical trial teams and experts building their AI portfolio. They help to make great AI research more tangible and usable throughout the industry. Per Li, “Academics want to work on novel, cutting-edge [opportunities], like expanding an algorithm or using it in a special way. To the extent that you can interest them in that, I think teaming up with academicians is probably the best thing you can do.”
With software like ChatGPT and the increasing inclusion of AI in bachelor’s degree curriculums, we are potentially moving toward what Li calls the “democratization of AI knowledge.” By making AI more accessible to nonexperts, costs will be lowered and AI introduction into clinical trials will run more smoothly.
Where Do We Go From Here?
AI is sprouting seemingly everywhere across the clinical space, and the hype is not unwarranted. That said, this growing alliance between tech and biopharma requires extensive collaboration, ample testing, and greater understanding across the board. As the technology spreads further into clinical trials, the goal of AI experts is to develop easy-to-understand technical solutions that encourage pharma companies to improve their process and allow patients to reap the benefits.