2 Key Areas To Leverage AI/ML For More Successful Clinical Trials
By Tina Hu-Rodgers, Buchanan Ingersoll & Rooney
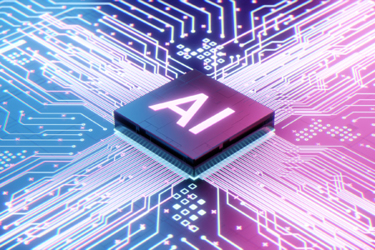
The adoption of artificial intelligence (AI) and machine learning (ML) has been one of the fastest growing trends across industries over the past decade. With the continuous advancements in technology, access to ever more powerful computers, increased availability of clinical and research data, and rapid development of novel algorithms that analyze and utilize that data, interest in applying AI and ML to trial design and clinical trials to improve high failure rates is increasing.
Among its many potential practical applications, AI and ML can be used to minimize errors in clinical trial participant management (e.g., cohort selection, patient identification and recruiting, participant retention) and streamline data management (e.g., automate data collection, monitor data quality, analyze large data sets).1 However, realizing the potential of this technology will require overcoming a range of different issues, including problems with data quality and access, transparency of underlying development and validation processes, potential bias inherent in the source data as well as the algorithm’s implementation, and the lack of definitive regulatory guidance from the relevant government agencies.
1. Patient Identification, Recruitment, And Retention
Selecting and recruiting patients for clinical trials is complicated and, despite the extensive time and effort companies put into clinical trial participant management, one of the biggest factors that causes a clinical trial to fail is failure to select and recruit the most suitable subjects for a trial.2 Participant dropout and non-adherence can also frequently cause studies to be delayed, go over budget, or fail to produce useable data.1 It is estimated that 86% of all trials do not meet enrollment timelines and nearly one-third of all Phase 3 trials fail due to enrollment problems.3 Addressing these issues early on can potentially shorten the clinical trial phase and help to reduce the risk of clinical trial failures that are not attributable to the drug being studied. ML can help researchers minimize errors in these areas by:
- Improving the selection of specific patient populations for trials by analyzing patient population data and identifying characteristics of patients that are most likely to benefit from the proposed drug or intervention (often informally called “responders”).1
- Improving individual participant identification and recruitment, such as using electronic health record (EHR) data to match patients to trials.1
- Identifying participants that are at high risk of study non-compliance1 and allowing researchers to intervene to ensure protocol adherence.
- Increasing trial participant adherence via real-time remote patient monitoring. Voice assistants powered by AI have already been adopted in many clinical trials for various routine monitoring tasks, such as sending patients appointment reminders, recording patient diaries, enabling more efficient collaboration between site investigators and sponsors, and increasing physician awareness.6
Unfettered by the limits of the human mind, digital tools can process many different kinds of available data to streamline and optimize the patient management process. Simulation studies have shown that adoption of ML methods resulted in more personalized treatment assignments and higher overall response rates among trial participants.4
However, using ML to identify more targeted patient populations does have its challenges, primarily with bias, which can skew and limit machine learning outputs. For example, selective identification of ideal patients based on EHRs and other available data may unfairly skew enrollment toward patient populations that have better access to healthcare and, therefore, have a greater opportunity to be selected based on their healthcare data. Patients located in rural, remote, or underserved areas who have more limited healthcare system access and interactions (and therefore less available data) are less likely to be selected for inclusion in these studies. The results of a study with such skewed enrollment, though likely to be more successful in the context of the clinical trial, will in fact be less accurate and less representative of the overall patient population. Theoretically, this could result in approval for drug products that work well in only a very small proportion of the intended treatment population for a particular disease or medical condition.
Similarly, in the context of using ML to ensure participant retention, monitoring, and protocol adherence (e.g., facial recognition software), there may be perception by participants that their privacy is being invaded, such that they do not wish to continue participation in the trial.1 Selecting patients who have the ability to access and feel comfortable with the necessary ML monitoring technology may also introduce bias.
Clinical trials have historically been plagued by issues regarding lack of diversity, with women, minorities, and patients from certain socioeconomic populations being consistently underrepresented in studies. In order to avoid having AI/ML further exacerbate these issues, companies will need to build health equity into their algorithms. Some have suggested a “domain-forward approach,” wherein domain experts” (e.g., healthcare professionals) are incorporated into the algorithm development process so that they can provide important context to address algorithms’ decision-making inaccuracies.5 Involving patients and caregivers in the development process can also help to ensure the real-world implications of the algorithms’ decisions are better understood. Companies looking to develop AI/ML algorithms to aid in clinical trials should also develop specific processes for routinely evaluating and addressing bias in their algorithms.
2. Data Collection And Management
The most obvious benefit of AI/ML in clinical research is data collection and management. ML can help researchers more easily collect, process, and manage data, by:
- Allowing for remote patient monitoring using digital health technologies, such as wearables, voice technologies, and computer vision. The ability of these technologies to collect longitudinal and real-time biometric data can provide insights into the long-term, real-world impact of therapies and treatment protocols. 6
- Offering different techniques for data collection, for example, patient-generated health data from wearable devices can replace the need for participants to travel for study visits and have their data collected using traditional methods.1
- Enabling use of new patient-centered digital biomarkers, which can facilitate efficient conduct and patient-centeredness of clinical trials.1
- Automating data collection, verification, and surveillance, thereby decreasing the time, expense, and potential for human error.1
- Maximizing the success of trials by analyzing data from prior trials to optimize clinical trial protocol development.7
- Optimizing analysis of data collected in trials, registries, and clinical practices.1
However, as with the previous discussions, use of ML in these ways is not without issues. For example, in the case of patient-centered digital biomarkers based on wearable data, ML processing of that data to derive research endpoints may lead to corrupt results if the ML model is intentionally or unintentionally disrupted by modified sensor data.
Before ML processing of device data can be broadly implemented, FDA approval of novel biomarkers will be required, and there needs to be better understanding of how previously validated clinical endpoints and patient-centered digital biomarkers overlap. There also needs to be a clear understanding of how the device wearers feel about the sharing and use of their device data (comprehensive informed consent is key). Researchers interested in taking advantage of the power of AI/ML-enabled devices need to clearly explain to patients the risks and benefits of their data collection, for both ethical and privacy reasons. Failure to address participants’ concerns regarding the technology being used has the potential to worsen participant recruitment and retention.
Conclusion
There is a lot of potential for ML to improve the efficiency and quality of clinical research, but as discussed above, a number of fairly significant challenges still remain. In addition to the outstanding issues of inherent bias, informed consent, etc., that companies must address with their algorithms, additional guidance and standard-setting by FDA and other regulatory agencies regarding transparency, oversight, and data quality in AI-ML processes will be necessary to reduce risks and discriminatory outcomes in clinical trials. Until then, the excitement for the potential applications of ML to clinical trials will likely continue to outpace its actual use.
References
- E. Hope Weissler, et al., The role of machine learning in clinical research: transforming the future of evidence generation, Trials, 22:537 (2021).
- David Wallace and Roger Kuan, Introduction, A practical cross-border insight into digital health law, Digital Health 2021, available at https://www.acc.com/sites/default/files/resources/upload/DH21_E-edition.pdf.
- Stefan Harrer, et al., Artificial Intelligence for Clinical Trial Design, Trends in Pharmacological Sciences, Aug. 2019, Vol. 40, No. 8., available at https://reader.elsevier.com/reader/sd/pii/S0165614719301300?token=768294224C0881D7D320F611B90096FC26D5201AC21ACB7BE0EA2661819EAB23BB06
493863C9A47D053DABFC62304D21&originRegion=us-east-1&originCreation=20220909193909. - Yizuo Wang, et al., Application of machine learning methods in clinical trials for precision medicine, JAMIA Open, 5(1), 2022, 1-10.
- Lucas Glass, Machine Learning-based Predictive Modeling: Notable Uses in Clinical Trials (Feb. 11, 2022), available at https://www.contractpharma.com/contents/view_online-exclusives/2022-02-11/machine-learning-based-predictive-modeling-notable-uses-in-clinical-trials/.
- Zhichao Liu, et al., AI-based language models powering drug discovery and development, Drug Discovery Today, Nov. 2021, Vol. 26, No. 11., available at https://reader.elsevier.com/reader/sd/pii/S1359644621002816?token=5F2B4D06283D7B0F5E3297AA94B7A78B8B75F651B7F1B4150C2795664B0B130B188
2B82819E20A78C729736A0C450816&originRegion=us-east-1&originCreation=20220921205958. - Brian Wilder & Maia Majumder, “A Domain-Forward Approach to Algorithmic Decision-Making: Lessons Learned from the COVID-19 Pandemic”
About The Author:
Tina Hu-Rodgers is counsel in Buchanan Ingersoll & Rooney’s Life Sciences industry group. She focuses her practice on issues related to the approval, regulation, promotion, sale, and reimbursement of drugs, medical devices, biologics, dietary supplements, foods, and cannabis-related products.