Leveraging AI For Data Analysis In Pharmacy-Based Trials
By Sandy Kim, Pharm.D.
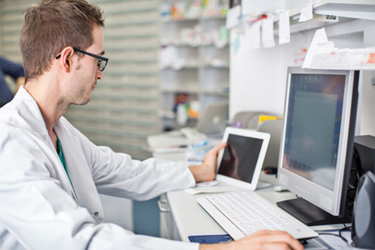
Independent pharmacies, estimated at over 260,000 worldwide, play a unique role in managing diverse patient populations and chronic conditions, making them valuable partners for clinical research.1 Their vast collection of real-world data — over 800 petabytes annually according to a 2022 IQVIA report2 — paints a comprehensive picture of patient medication use, adherence patterns, and feedback. Unfortunately, traditional clinical trials often face limitations in recruiting diverse participants and capturing real-world data effectively. Harnessing this wealth of data effectively requires innovative solutions beyond traditional methods. AI steps in as a transformative tool, offering powerful avenues for data analysis and unlocking the true potential of pharmacy-based trials.
Data Challenges And AI Solutions
Consider the following topical areas where clinical trials experience data challenges and how AI can help address them.
Data Volume And Variety
Pharmacies collect massive amounts of structured and unstructured data (prescription records, dispensing patterns, patient feedback, etc. ). Analyzing this intricate tapestry can be overwhelming for traditional techniques. However, AI's ability to handle complex data sets can be beneficial.
- Natural Language Processing (NLP): This AI technique deciphers unstructured data like clinical notes and patient experience feedback, extracting valuable insights hidden within.3,4 For example, NLP can identify patient sentiment and medication concerns from pharmacy feedback, potentially informing future trial designs.5
- Machine Learning: These algorithms identify patterns in prescription records, uncovering medication adherence trends, potential drug-drug interactions, or risks for individual participants in clinical trials, even those previously unknown.6
Data Integration And Standardization
Inconsistent data formats and lack of standardization across pharmacies create integration hurdles, limiting comprehensive analysis. Federated learning emerges as a promising solution. These AI techniques allow distributed analysis of data across different pharmacies without compromising privacy, enabling researchers to gain valuable insights from a collective wealth of information. Each pharmacy stores its data locally, but AI algorithms can access and analyze it without transferring raw data, preserving privacy. This collaborative approach allows researchers to identify broader trends and patterns across diverse patient populations. 7,8
Identifying Eligible Participants
Selecting appropriate participants for trials is crucial, considering medical history, medication adherence, and potential risk factors. Traditional methods can be time-consuming, subjective, and prone to bias from relying on self-reported data. AI-powered algorithms analyze patient data with remarkable speed and accuracy, identifying ideal candidates who meet specific criteria more objectively.
Real-World Evidence (RWE) Generation
Real-world data from pharmacies paints a realistic picture of drug effectiveness and safety in everyday settings, complementing the controlled environment of traditional clinical trials. AI analyzes this data to generate robust RWE, identifying trends and patterns that might not be evident through traditional statistical methods. 9
Predictive Modeling And Risk Assessment
By analyzing historical data and identifying patterns, AI can predict potential risks and adverse events during trials. This proactive approach allows researchers to implement safety measures beforehand, minimizing risks for participants and ensuring ethical conduct. 10
Benefits For Stakeholders
Clinical trials in the pharmacy setting present unique benefits to pharmaceutical sponsors, pharmacies, and patients. Here are a few ways each can reap their rewards:
Sponsors
- Faster and more efficient trial design and participant selection: AI streamlines processes, significantly reducing timelines and costs associated with participant recruitment and data analysis.
- Deeper insights from real-world data: AI-powered RWE generation provides a more holistic understanding of drug effectiveness and safety, leading to more informed decisions and improved drug development strategies.
- Reduced costs and improved trial feasibility: By optimizing processes and utilizing RWE, AI can significantly reduce the costs and complexity of conducting clinical trials, making them more accessible and feasible.9
Pharmacies
- Enhanced patient care through involvement in cutting-edge research: Participation in AI-powered trials allows pharmacies to contribute to advancements in healthcare and offer their patients access to innovative treatments closer to home.
- Diversified revenue streams through participation fees and data analysis services: Pharmacies can leverage their data and AI expertise to participate in trials and offer data analysis services to other stakeholders, generating additional revenue streams.
- Improved operational efficiency through AI-powered tools: AI can automate tasks like medication reconciliation and adherence monitoring, freeing up pharmacist time and improving overall operational efficiency.
Patients
- Access to innovative treatments closer to home: Pharmacy-based trials increase accessibility to cutting-edge treatments for patients who might not be able to participate in traditional hospital-based trials.
- Potential for personalized medicine approaches based on AI-driven analysis: AI can analyze individual patient data from pharmacies to tailor treatment plans and dosages for optimal patient outcomes.
Ethical Considerations And Regulatory Landscape
However, introducing clinical trials into the pharmacy setting with the assistance of AI must be done with careful consideration of data privacy and security issues as well as regulatory compliance. Robust data security measures and clear patient consent procedures are crucial when using AI in healthcare. Encryption, adherence to regulations like Good Machine Learning Practice (GMLP), General Data Protection Regulation (GDPR), HIPAA, and anonymization techniques are essential to protect patient privacy and build trust.11, 12 Ensuring adherence to data privacy regulations and ethical guidelines for clinical research is crucial. Regulatory bodies are actively developing frameworks for AI in healthcare, and navigating this evolving landscape requires collaboration between researchers, industry professionals, and regulators.
Conclusion
Leveraging AI for data analysis in pharmacy-based trials holds immense potential for revolutionizing drug development and healthcare delivery. By unlocking the vast insights hidden within real-world pharmacy data, AI can accelerate trial design, personalize treatment plans, and ultimately improve patient outcomes. As we move forward, addressing ethical considerations, ensuring data privacy, and fostering collaboration among stakeholders will be crucial for realizing the full potential of this transformative technology.
References:
- National Community Pharmacists Association. (2023). NCPA Digest: Key statistics about independent pharmacies. https://ncpa.org/annual-report
- IQVIA Institute for Human Data Science. (2022). The 2023 IQVIA Institute for Human Data Science Trends Report. https://www.iqvia.com/blogs/2023/02/iqvia-institute-research-highlights-2022
- Harrison, C.J., Sidey-Gibbons, C.J. Machine learning in medicine: a practical introduction to natural language processing. BMC Med Res Methodol 21, 158 (2021). https://doi.org/10.1186/s12874-021-01347-1
- Khanbhai M, Anyadi P, Symons J, Flott K, Darzi A, Mayer E. Applying natural language processing and machine learning techniques to patient experience feedback: a systematic review. BMJ Health Care Inform. 2021 Mar;28(1):e100262. doi: 10.1136/bmjhci-2020-100262. PMID: 33653690; PMCID: PMC7929894.
- Reed, J. Z. (2022, July 6). NLP Analyzes the Past to Inform the Future of Clinical Trial Design. Applied Clinical Trials Online.
- Shinozaki, A. (2020). Electronic Medical Records and Machine Learning in Approaches to Drug Development. IntechOpen. doi: 10.5772/intechopen.92613
- Rahman, A., Hossain, M.S., Muhammad, G. et al. Federated learning-based AI approaches in smart healthcare: concepts, taxonomies, challenges and open issues. Cluster Comput 26, 2271–2311 (2023). https://doi.org/10.1007/s10586-022-03658-4
- Zhang, Fan, et al. “Recent Methodological Advances in Federated Learning for Healthcare.” arXiv:2310.02874 [cs.LG]. 4 Oct. 2023.
- Zhaoyi Chen, Xiong Liu, William Hogan, Elizabeth Shenkman, Jiang Bian. Applications of artificial intelligence in drug development using real-world data. Drug Discovery Today, 2020. PMID: 33358699 DOI: 10.1016/j.drudis.2020.12.013
- “An AI Model Can Help Predict Adverse Events From New Drug Combinations.” AACR Cancer Researchers / Other Health Care Professionals, 8 Apr. 2022.
- GDPR Local. The Newest Trends in Data Protection and GDPR in the Age of AI in Clinical Trials. 2024, February 21).Retrieved from GDPR Local.
- Mai, B., Roman, A., & Suarez, A. (2023, June 20). Forward Thinking for the Integration of AI into Clinical Trials. Clinical Researcher, 37(3). Retrieved from ACRP Home News & Press
About The Author:
Dr. Sandy Kim has over 5 years of patient care experience in outpatient settings, coupled with a passion for clinical research. Her most recent work in the biotech industry focused on oncology, where she made significant contributions to cutting-edge research.
Dr. Kim actively collaborates with pharmacy and research healthcare professionals, as well as platform start-ups, to explore innovative and collaborative approaches in clinical research. She is particularly interested in pharmacy outreach within community settings and integrating AI advancements into clinical trial systems. Dr. Kim is a clinical research advocate, driven by her commitment to patient safety and ethical research. She actively promotes a cooperative approach involving community and independent pharmacy services in clinical trials.