Leveraging AI To Assess Clinical Trial Technologies
By Jeffrey S. Handen, Ph.D., and Nate Regimbal, Grant Thornton LLP
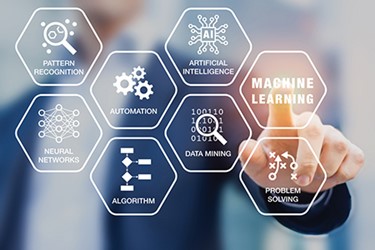
As part of our ongoing series detailing an approach for the rapid assessment and prototyping of digital and other clinical trial technologies,1 we introduce here the use of artificial intelligence (AI) and machine learning (ML) to optimize clinical study design and execution. The previous article presented the development of a ~70+ step configurable clinical study model that defines the time and costs associated with the individual steps that make up a “typical” clinical study, from clinical development planning (CDP) to clinical study writing (CSR) and that allows for user-defined customizations.2 With this model, clinical study teams can identify those steps most amenable to “digital/technological” intervention(s) and the hypothesized ROI. Applying AI and ML concepts then allows an efficacy assessment of the proposed intervention(s) — a probability of success (POS) assessment, if you will.
What Are AI And ML?
In its simplest definition, AI is the simulation of human intelligence by machines, and ML is getting those machines, or computers, to perform without explicit programming. In development for decades, these concepts have been popularized through the use of AI in such ventures as world-class chess competition, where IBM’s Deep Blue supercomputer first beat the reigning world champion and Chess Grand Master Gary Kasparov in 1997, and the television game show Jeopardy!, where in 2011, IBM’s latest foray into AI, the Watson supercomputer, routed the best human contestants. More recently, with the advent of exponentially increasing availability of both data, i.e., Big Data, and computing power, AI has moved on to perhaps more practical applications ranging from self-driving cars and agriculture to medicine and finance. Estimates of current investments in AI development exceed $30 billion,3 with projected ROI measured in the trillions.
The “engine” behind AI is ML — a subfield within data science. ML algorithms detect patterns and learn how to make predictions and recommendations by processing data and experiences, rather than by receiving explicit programming instruction. The algorithms also adapt in response to new data and experiences to improve efficacy over time.4
A detailed treatment of how AI and ML work is not the intent this article, but perhaps AI and ML may be best explained by summarizing what they can do. AI capabilities can be divided into five categories — with each category providing examples of usage scenarios (types of use cases).
Image created based on information from the Microsoft Azure website.
Data Considerations In AI And ML
AI and ML data science techniques and technologies enable the detection of relationships and value levers within and across information and data that would be difficult or impossible to find using traditional business informatics tools or via descriptive analytics techniques. With AI, highly instrumented ML models can detect not just primary and secondary relationships across data objects and AI-detected concepts, but also tertiary and quaternary relationships and beyond. AI model evolution via inherent machine learning capabilities means that AI is able to unlock hidden values within and across data sets — whether it’s your own data or the data of partners or subscription content providers.
Because of AI’s ability to find relationships across data, clinical researchers can leverage AI use cases that utilize data both within and across data sources.
External data sources include public and subscription data such as:
- Freely accessible online databases like PubMed, SciFinder, DrugCentral, DrugBank, and more generic abstracting indices like Google Scholar
- Elsevier, Clarivate, Wolters Kluwer, Thompson Reuters, Frost & Sullivan, and other similar commercial subscription databases
- MedWatch/FDA AERS, EU-ADR
- Health authority correspondence data, e.g., regulations, guidances, and inspection and audit findings, etc.
- Social media sites such as Twitter, Facebook, WebMD blogs, and online patient forums
- Clinicaltrials.gov, EU Clinical Trials Register
Internal data sources are proprietary and affiliate data contained in clinical systems, such as EDC (electronic data capture), ePRO (electronic patient-reported outcome), CTMS (clinical trial management system), eTMF (electronic trial master file), and LIMS/LNs (laboratory information management system/laboratory notebooks).
Applying AI And ML To The Configurable Clinical Study Model
So how does one apply these concepts to our configurable clinical study model? In brief, once users have identified potential interventions, e.g., new technologies or potential levers through the clinical study model in a sensitivity analysis to quantify hypothesized ROI, they can assess the POS of the potential interventions using machine learning powered search to identify trends and insights from real world data and real world evidence to corroborate or refute the efficacy of the potential interventions. Take, for instance, the use cases of assessing the POS of streamlining a potential step in the clinical study process or a particular study design or cohort identification. Examples include:
- In designing a rare disease study of subcutaneous immunoglobulin for chronic inflammatory demyelinating polyneuropathy (CIDP), a disease that is estimated to affect only about 30,000 patients each year in the U.S.
- What can AI tell me about similar studies on CIDP, possibly with other interventions?
- What can AI tell me about the CIDP patient population in general?
- What can AI tell me about studies using subcutaneous immunoglobulin, in other populations/studies?
- What can AI tell me about rare disease studies in general?
- Using AI to identify very specific patient populations/target cohorts characterized down to the inclusion/exclusion level.
- Using AI to identify trends and insights in discrete process steps, e.g., site initiation or serious adverse event (SAE) reconciliation in a particular therapeutic area, disease indication, or study design, etc.
Demystifying AI via pragmatically answering the what, why, and how of AI can be used to explore these use cases. Historically, this required significant financial and organizational investments in developing and implementing strategies and deploying a road map. However, due to recent and rapid technological and methodological advancements, lower touch alternatives now exist. Here we present one such rapid, low-cost approach — the minimum viable product (MVP).
The MVP approach leverages the Software-as-a-Service (SaaS) model to deliver AI-as-a-Service (AIaaS) using Agile methodology, negating the need for large investments in AI tools and platforms. In the MVP approach, internal and external data sources are first grouped together in a “data supply chain” and fed into the AI engine containing the focused use case-specific ML algorithms. These engines can run in the cloud or behind your firewall.
The outputs of the AI engines are then delivered to human users of existing or new use case-specific applications. The AI insights can also be sent to other systems. For example, if the AI models predict that a certain proposed digital intervention to a lever in the clinical study model will either fail or cause an increased risk of deviations, the AI can send an alert to a user. The models can also instruct other systems and/or users to update contingency planning and CAPAs. Such would be a type of prescriptive analytics use case — utilizing AI-powered near-real-time optimization.
Leveraging The Output
By leveraging this pragmatic AI approach, clinical research professionals can select the most relevant interventions to pilot either virtually in “conference room” exercises, in separate studies, or in real life by assigning different arms of a study to different technology or process interventions, analogous to different treatment arms but without the necessary statistical rigor or GxP implications. In this way, the hypothesized efficiencies can be confirmed or disproved in a quantifiable fashion without having to incur any switching costs associated with rolling out a new technology to an entire study or portfolio. Organizations can further assess the qualitative impact and cost through a very brief Net Promoter Score (NPS) survey of the user experience and behaviors of all stakeholders (e.g., patients, sites, sponsors), which can be combined with the quantitative pilot results in decision making. In our next and final article in this series, we will explore this NPS approach through a look at the change management aspects of introducing new (technological/digital/process) “interventions” into the clinical study.
References:
- “Assessing And Implementing Clinical Trial Technologies: A 3-Step, Adaptive Approach,” J.S. Handen, Clinical Leader, March 5, 2019
- “Building and Applying a Configurable Clinical Study Model,” Handen et al., Clinical Leader, April 25, 2019
- “Artificial Intelligence. The Next Digital Frontier.” McKinsey Global Institute, 2017
- “An executive’s guide to AI,” McKinsey & Company.
About The Authors
Jeffrey S. Handen, Ph.D., is a director in the Life Sciences practice at Grant Thornton LLP and a leader in R&D transformation. He has published in multiple peer-reviewed and business journals, presented at numerous industry conferences and scientific meetings as an invited speaker, and served as past editor in chief of the Industrialization of Drug Discovery and Re-inventing Clinical Development compendiums. Handen has more than 20 years’ experience in pharmaceutical and biotechnology R&D, process re-engineering, and systems and process implementation. He is responsible for overseeing business process improvement and solution architecting for optimizing both clinical development and discovery. You can contact him at jeff.handen@us.gt.com.
Nate Regimbal is a digital transformation consultant at Grant Thornton LLP, helping companies define and implement digital and organizational strategies and solutions that enable business and growth objectives. Regimbal has served life sciences and medical technologies clients for more than 12 years in consulting and business development roles. Previously, he served in industry, managing instrumentation facilities in academic and biopharma R&D.