Leveraging RWD With AI To Enable Diverse Recruitment In Clinical Trials
By Lakshmi Sankar and Isabelle Cheung, PA Consulting
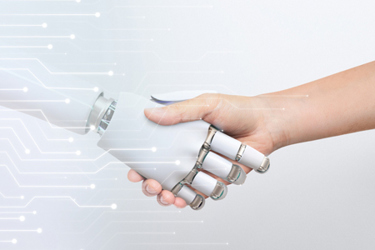
Patient diversity in clinical trials is posing a major challenge for today’s pharma companies. In the U.S., only 4%–6% of oncology trial participants are Black and 3%–6% are Hispanic, despite making up 15% and 13% of cancer patients, respectively. These statistics highlight why clinical trial researchers and sponsors are focusing more than ever on ensuring clinical trials are more representative of the populations they serve. Our recent survey of 2,000 respondents across the U.S. sought to understand public attitudes toward clinical trials, revealing that three-quarters of those surveyed believe that more diversity in clinical trial recruitment will increase the effectiveness and suitability of drugs for a broader population.
Patient diversity is a moral and public health obligation that needs to be addressed swiftly. The updated draft guidance from the FDA on Diversity Action Plans now paves the way for mandated trial diversity. By helping clinical trial sponsors submit Diversity Action Plans, the FDA is working to ensure the adequate participation of relevant and underrepresented populations in clinical trials and enable the analyses of data collected from clinically relevant populations.
Clearly, a diverse cohort of research participants is vital to developing inclusive studies that are representative of the target population. This is especially key for understanding patients’ healthcare needs and challenges in underrepresented communities. The challenge for pharma companies is to enhance DEI in clinical trial research by using real-world data and evidence, leveraging AI to more efficiently and intelligently source data to improve DEI design.
Applying Real-World Data And Evidence To Improve Clinical Research Diversity
The current gold standard for evaluating drug safety and efficacy is through randomized clinical trials (RCT). However, data shows that there is a large gap between the populations examined in most clinical trials and the broader patient population. Therefore, it is important to consider how study results can be generalized for use by the patient population once the drug or medical product is approved, since these clinical trials often lack diverse representation.
In the U.S., the epidemiological data that is often needed to inform RCT eligibility criteria is derived from the U.S. Census Bureau’s race and ethnicity data. However, to develop more inclusive RCT eligibility criteria, researchers can collect and analyze real-world data (RWD) from patient registries and electronic health records to reveal any differences and patterns in the diagnosis, treatment, and response within diverse populations. The 2023 publication in Clinical Trials: Journal of the Society for Clinical Trials, which examined clinical trial diversity across 495 GSK and ViiV trials involving over 100,000 participants, highlighted how utilizing U.S. Census Bureau race and ethnicity data may not accurately represent the percentage of the population that could be affected by a given disease. The retrospective study revealed that while this data states that 13.4% of the U.S. population is Black or African American, RWD (of disease epidemiology) showed that 17% of the U.S. population with asthma, 7.1% of those with COPD, and 55.3% of those with HIV are Black or African American. This suggests that incorporating external RWD may better represent a real-world demographic for a given disease.
As well as its input in study design, RWD can be used to reveal regions that have under-recruited and under-enrolled patients, influencing where sponsors may geographically set up specialty clinics or community practices to tap into specific patient pools. Assessing and segmenting disease incidence across different groups can help researchers target specific groups to improve trial diversity. Beyond finding patients for trials, RWD and real-world evidence (RWE) can be used to monitor the performance of a drug or device post-regulatory approval and passively track patient health outcomes and behaviors post-launch, particularly in underrepresented groups. If harnessed in the right way, RWD can produce evidence that is more representative and generalizable for future trials than data collected from RCTs.
While there is a growing awareness of the challenges in accessing robust, reliable data, pharma companies are also grappling with how to explore the need for potential heterogeneous treatments and the varied responses to treatments across racially and ethnically diverse patient populations. The use of RWD could be the answer to this challenge — specifically when paired with the ability of artificial intelligence to automate, scale, and accelerate the use of RWD for this purpose.
Leveraging AI To Drive Valuable Insights
Today, with tools such as electronic consent forms, virtual visits, digital tracking and testing, and remote patient monitoring, digital health technologies (DHTs) are being integrated into the end-to-end clinical trial life cycle, providing organizations with an even bigger opportunity to leverage their source data (RWD) to improve DEI design in clinical trials.
Data can be paired with AI and machine learning algorithms to integrate unstructured data from health records, registry and historical trial data, and RWD from DHTs to drive compelling insights on who to recruit. For example, natural language processing (NLP) techniques can be used to extract relevant information (e.g., patient eligibility) from unstructured data sources, allowing for the incorporation of valuable patient data that may not be captured in structured fields. AI algorithms can then analyze vast patient data sets from diverse sources to ensure a thorough and fair matching process. Machine learning models can also be designed to predict a patient’s clinical outcome. This allows for a more targeted recruitment of patients who are high risk or more likely to respond to treatment. AI can also be used to analyze multimodal data, including genomic information, to select the ideal patients for clinical trials — an approach that has the potential to reduce required sample sizes while maintaining statistical power.
There is no denying that leveraging RWD and RWE holds great potential in identifying participants and sites. Embedding RWD and RWE into clinical trial design and site selection is one way in which the recruitment of diverse participants can be enhanced, particularly in populations that are typically underrepresented and hard to reach. RWD and RWE provide researchers with a fuller analysis of healthcare needs by population. Establishing and scaling RWD and RWE across clinical operations and site selection will help pharma companies and clinical research organizations ensure that the research produced is robust, enabling the outcomes to be equitable, diverse, inclusive, and representative of diverse communities. This is the ultimate goal of true patient centricity.
About The Authors:
Lakshmi Sankar is an organization strategy expert working in healthcare and life sciences at PA Consulting. Her key expertise lies in leading large-scale business and organizational transformations across the clinical R&D ecosystem. Her current work focuses on talent strategy, workforce of the future, leadership development, communications, and cultural change to build sustainable, inclusive organizations and create long-term value.
Isabelle Cheung is an organizational agility expert at PA Consulting. She has expertise in enterprise-wide transformation, with a focus on patient-centric design. With experience across the healthcare value chain, Cheung is equipped and passionate about helping healthcare and life sciences organizations unlock the benefits of agility so they can deliver value faster and create more engaged workforces.