4 Pitfalls To Avoid When Starting Your AI/ML Journey
By Laurie Stone and Elise Watson, Clarkston Consulting
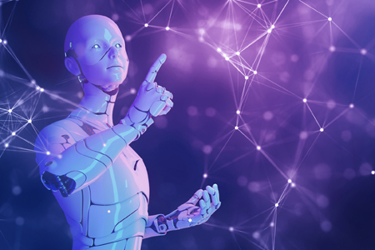
Getting a new compound or drug to market today requires life sciences companies to push the boundaries of possibilities to improve trial operations at any stage of development. With less than a 14% overall chance that a drug tested in Phase 1 reaches approval,1 it is clear that clinical trials need the analytical power, speed, and innovation required for today’s more complex therapies and diverse populations.
To highlight how biotech and pharma industries are leveraging machine learning (modern data science) to drive better study design, study teams are setting themselves up for more successful and realistic predictive outcomes with less time spent on protocol development and related amendments.
One strong use-case for artificial intelligence (AI)/machine learning (ML) is in selecting sites where there is enough of the target patient population who meet inclusion/exclusion criteria. So many trials fail not because the molecule isn’t efficacious but because the target population could not be recruited. ML-based algorithms supported with real-world evidence use claims and surveillance data to map patient populations that help identify sites with the highest recruitment potential. With ML-based algorithms, we can answer some common clinical trials questions, such as:
- Who are the most similar healthcare providers (HCPs) to our investigators in terms of drugs or diseases investigated at a local, national, or global level?
- Which of these similar HCPs work within a specific region or have affiliations to a specific hospital?
- What communities exist within HCPs in terms of shared sponsors? Are there discrete communities within competitor sponsors? Do we have inroads with these competitor communities via referrals, events, other engagements, etc.?
- What are the top sites for diseases investigated, total enrollment, number of completed trials, etc., that the client has not sponsored?
By focusing on the optimal site leveraging AI/ML capabilities, your company can reduce the number of sites involved and mitigate the risk of not meeting the required sample size.
Planning Your AI/ML Transformation Journey
With the transforming AI landscape, it is important your company take action to maximize your returns on AI-enabled R&D transformation. Key stakeholders within your company should identify a small number of high-impact AI use cases and prioritize these use cases based on feasibility within each department. It is important to remember that emerging technologies, such as AI and ML, do require a large volume of current, clean, and accurate data from different business silos to function (Refer to Image 1).
When developing your transformation strategy, consider the following best practices, such as:
- Map out existing processes.
- Define goals.
- Design a clear road map.
- Evaluate internal data science capabilities and consider collaborations.
However, when considering some of these best practices, it is also important to keep in mind strategies to tackle the following pitfalls that come with implementing AI/ML into clinical trials.
Pitfall 1: Not Employing The Proper Talent
It is important to have a skilled team when taking on your first AI/ML project to create a reliable process road map for the internal teams to follow and implement. However, one of the biggest issues is a shortage of qualified workers with the right technical skills coupled with a high turnover rate. Upskilling those already in the industry will be a key factor in improving AI and attracting skilled data scientists to roles in life sciences.
In the meantime, a solution is to work with technology partners who offer skilled AI/ML teams and have a single stakeholder own the AI/ML adoption within the enterprise. They can identify the data science and allied skills gap within the organization with reference to the goals they are trying to achieve and then work with the relevant departments to hire or build the right skills into the organization for end-to-end success.
Pitfall 2: Poor Data Affects Outcomes
No matter how good your model is, it is only as good as the data you provide. Machine learning specifically looks for historical patterns and then makes predictions based on that history. If you want the patterns in the future to change, it is important to account for that in the data or model. Machine Learning is, after all, data-driven AI, and your model will only be as good or as bad as the data you put in. The importance of reliable data cannot be overstated. Getting the right data can be the hardest part of a project, especially if you are trying something new. To start, it is important to only use and put in data that is from a verifiable source. But even with data that is from a “trusted” source, you will still need to check if it is useful (good or bad) data. It is important to kick off each project with a data profile that visualizes what kind of data you have and how it correlates with each other and your purpose. This data profile, along with an ethical understanding of each source, will ensure the data selected creates models that are built to accurately predict unbiased outcomes.
In AI, the “garbage in, garbage out” concept is critical when building algorithms. The lack of standards, naming conventions, verification, validation, and automation contributes to data quality issues. This leads to significant manual intervention that won’t scale well. Additionally, employees spend their time getting the data to a workable state instead of on data analysis. This frustrates employees, which could lead to high turnover and failed trials. There are currently no industry-wide data standards that include patient data in the broadest possible sense and from a wide range of sources – including wearable and mobile device data collected for different purposes, such as provider reimbursement, clinical research, and direct patient care.
Significant time and resources are needed to integrate data into corporate systems (i.e., data lake houses) and make it usable. Collaboration is needed between pharma/biotech companies and data and technology firms. Data may be stored in different formats using different database systems and information models, and many companies have incorporated the adoption of Common Data Model (CDM), which transforms data contained within multiple databases and sources into a common format (data model) as well as a common representation with standard terminologies, vocabularies, and coding schemes.
Pitfall 3: Absence of a Data Governance Framework
The massive amounts of data needed for AI/ML solutions are often collected in silos across different business functions with different formats and databases. And while that’s okay, the challenges companies face are inconsistent data formats, multiple views of data through different systems, human errors, and numerous repeat data entries by multiple users, which can all lead to data inconsistencies and anomalies. A strong data governance framework is needed to manage and gain better control over data assets and should include four key elements: data integrity (accuracy and completeness of the data), data storage and integration (where the data is stored and how it moves across different databases/systems), data visibility, and data security.
Pitfall 4: Absence Of Robust Change Management
Digital transformation requires a change in mindset,2 collaboration, and support. As such, it is important to anticipate challenges to ensure your company benefits from AI/ML technology. Robust change management initiatives provide a road map for AI/ML implementation that will guide your company through the change to become more data literate. Casting awareness on the importance of data within your organization will lead to increased quality of data, more accurate analytics, and ultimately overall adoption of predictions through the trust established between your employees and the data.
Looking Ahead With AI/ML In The Life Sciences
The life sciences industry has certainly not scaled or realized the full potential of AI/ML, but several use cases are available today to help start the journey. Basic science and translational research can leverage ML to propose new therapeutic molecules, analyze research output, and propose next steps. Clinical trials and observational research can leverage ML for pretrial planning (protocol development, drug regimen selection, site selection), participant management (cohort selection, patient identification, participant retention), and data management (automate data collection, improve data quality, analyze large datasets, unlock novel biological features). With greater awareness of what is available in the AI/ML landscape and an understanding of what action to take, companies can get started now to prepare for what’s yet to come for their enterprise.
References
- Wong C.H., Siah K.W., & Lo A.W. (2019, April 1). Estimation of clinical trial success rates and related parameters. Biostatistics. https://doi.org/10.1093/biostatistics/kxxo69.
- Rosenstock, S., Hidalgo, I., & Rowland, H. Consumer products trends – adopting a digital mindset. (2022, October 5). https://clarkstonconsulting.com/insights/adopting-a-digital-mindset/
About The Authors:
Laurie Stone, principal consultant at Clarkston Consulting, has 20+ years of experience in clinical operations across different stages of development, providing expertise in quality, management, and compliance in the biotech, pharma, and medical device industries.
Elise Watson is a service lead and delivery senior manager for Clarkston Consulting’s Insights to Actions team, with a background in mathematics and machine learning.