RWD/RWE: To Replicate Or Continue To Learn In The Real World?
By John Cai, MD, Ph.D., executive director of real-world data analytics and innovation, Merck & Co.
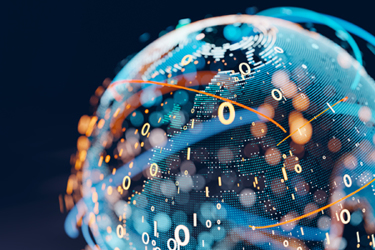
Real-world evidence (RWE) derived from real-world data (RWD) was first coined by the pharma industry about 12 years ago to address the “fourth hurdle.” It is no longer sufficient to demonstrate to regulatory agencies a product's safety, efficacy, and quality, which represent the first three hurdles. Manufacturers must now demonstrate both clinical effectiveness (is the new product better than currently available alternatives, including no treatment?) as well as cost-effectiveness (is the product good value for money?) to gain market access and reimbursement for a pharmaceutical, medical technology, or biotech product. These days, in addition to using RWE for clinical- and cost-effectiveness, i.e., the fourth hurdle, RWE is also now being used for regulatory reviews of safety, efficacy, and quality. For instance, the FDA now considers RWE as part of the evidence package for submissions seeking authorization to market new medical products, including new drug applications (NDAs) and biologics license applications (BLAs).
Several studies have been conducted to examine if RWE can replicate findings from randomized clinical trials (RCTs). In a 2019 JAMA network open study, authors examined 220 U.S.-based trials published in the seven highest-impact journals and found that only 15% of these trials could be feasibly replicated through analysis of electronic health records (EHR) or administrative claims. Multiple such studies have been driven by the RCT Duplicate Initiative, with a goal to match the results of published trials with non-interventional studies (NIS) based on healthcare databases. In its first results published in circulation in 2020, differences between RCT and corresponding RWE study populations remained, despite attempts to emulate RCT design as closely as possible. In addition to asking such replication questions, researchers may ask what can be learned from differences between RCT evidence and RWE, and decision makers may even ask whether such differences can be predicted. It is a mindset change to shift from replication to continuous learning, which in turn may inform different types of decisions.
This article will cover different types of decision-making using RWD/RWE across pharma companies, healthcare providers (HCPs), healthcare systems, payers and health technology assessment (HTA) agencies, regulators, and patients (as illustrated in Figure 1).
Figure 1. An illustration of the RWD/RWE journey from healthcare systems to research communities and back to for decision-making. RWD: real-world data; NIS: non-interventional studies; RWE: real-world evidence; HTA: health technology assessment agency; HCP: health care provider.
How Do Stakeholders Currently Use RWD/RWE?
- Healthcare providers (HCPs) use RWD/RWE for their decisions on treatment plans and orders of tests, procedures, and prescriptions for individual patients. At system or population levels, healthcare providers also could use RWD/RWE to adopt/adapt/contextualize clinical practice guidelines and develop clinical decision support tools.
- Payers use RWD/RWE for their decisions on drug formularies, health plan coverages, and reimbursements of individual claims.
- Patients themselves could also search the internet for relevant information or use smart apps that leverage RWD/RWE for their decisions on treatment preferences and medication adherence.
- Regulatory agencies such as the FDA could review RWD/RWE as a supplement to RCT evidence for marketing authorization, in addition to using RWD/RWE to monitor post-market safety and adverse events.
- Health technology assessment (HTA) bodies could review RWD/RWE to make positive or negative reimbursement recommendations to payers.
- Pharmaceutical companies could use RWD/RWE in their internal decision-making for: 1) research decisions in drug discovery and preclinical development; 2) clinical trial design and interpretation; 3) innovative, new treatment approaches in the real world; and 4) commercialization.
Decision Support with Replication Efforts: Use RWD/E To Confirm RCT Results
Replication efforts generate RWE from RWD with one major question in mind – can RWD/RWE replicate the RCT results? In terms of decision support, these efforts aim to defend the decisions that have been made based on the RCT evidence by the following stakeholders:
- HCPs may use RWD/RWE to confirm that RCT evidence is applicable to individual patient care. When individual patient outcomes in the real-world setting are consistent with those from the RCTs, it would give HCPs more confidence to apply such RCT evidence to their clinical practice. However, when individual patient outcomes in the real-world setting are inconsistent with those from the RCTs, HCPs may or may not have the resources or interest to do further research to generate RWE.
- Healthcare systems may use RWE to confirm that RCT-based clinical practice guidelines (CPGs) are applicable to the local systems. Assuming a healthcare system has its own RWD database to generate RWE, such confirmations would improve the adoption of CPGs locally. Alternatively, a health system may rely on other healthcare systems to generate RWE, assuming the RWE is generalizable and applicable to its own system.
- Payers may use RWE to confirm RCT-based decisions on drug formularies, health plans, and reimbursements. Payers usually have their own databases of insurance claims as RWD to generate RWE, which they may consider more relevant and informative to their decisions.
- Patients may use RWD (e.g., real-world experience from other patients) to confirm RCT-based treatment selections. Patients may not have the expertise to do data analysis but could rely on individual experience as RWD.
- Regulatory agencies typically use RWE of a product to confirm the initial RCT-based marketing authorization. This is usually done through post-marketing study commitments from the manufacturer to gather and submit additional information about a product's safety, efficacy, or optimal use.
- HTA bodies may request RWE to confirm RCT-based reimbursement recommendations on certain health technologies, including pharmaceutical products. The RWE would be better derived from the RWD in the local market or country.
- Pharma companies use RWE to confirm their own RCT results via post-marketing studies as required by regulatory agencies. These studies may be post-authorization safety studies and/or post-authorization efficacy studies.
Decision Support with Continuous Learning Efforts
Continuous learning efforts generate RWE from RWD with one major question in mind: What can we learn from the real world? These efforts are driven by the decisions to generalize the RCT evidence to broader real-world populations or contextualize it to local healthcare practice patterns, including but not limited to the following:
- HCPs use RWE to provide care to individual patients when their patients don’t really match the RCT’s selection criteria. The questions they are asking are what treatments are most effective for patients who were not really represented in the RCTs because they have different characteristics (such as age, gender, race, or ethnicity), more comorbidities, and/or different treatment histories.
- Healthcare systems may use RWE to contextualize/adapt CPGs that are primarily based on evidence generated from RCTs. By generating RWE using local RWD, they can address questions on whether and how to implement new therapies as recommended in CPGs in the context of local care pathways, even when the local standard of care (SoC) was not compared to in RCTs.
- Payers make more informed decisions using RWE on drug formularies, health plans, and reimbursements. RWE derived from more relevant RWD can help payers address questions on what care pathways are more cost-effective for the patient populations in their health plans when they are different from those enrolled in the relevant RCTs or when they would consider different comparators.
- Patients may be able to make informed treatment choices with relevant RWD/RWE. The question a patient would ask is usually: "What treatment option would work better for me when no patients like me were enrolled in the relevant RCTs?"
- Regulatory agencies may review RWD/RWE of a product after the initial marketing authorization to address comparative effectiveness questions that were not addressed by the RCTs.
- HTA bodies may review RWD/RWE after the initial reimbursement recommendations, that is, health technology reassessment. The questions to address here may be about comparative effectiveness between the technology and its alternatives.
- Pharma companies may further differentiate their products using RWE. The questions to address could again be about comparative effectiveness against comparators in the real world or whether a RWE-based tool can improve the value realization of the treatment. Alternatively, pharma companies may use RWE to inform clinical trial design internally (e.g., comparator selection).
To summarize, it is a mindset change to shift from replication to continuous learning, which in turn may inform different types of decisions. The table below compares the different types of decisions side-by-side with these two different approaches.
This article follows my presentation on the same topic at SCOPE 2022.
Disclaimers: The opinions expressed in this feature are those of the interviewee and do not necessarily reflect the views of any organizations.
About The Author:
John Cai, MD, Ph.D., FAMIA, is executive director, real-world data analytics and innovation, in the Merck Center for Observational and Real-World Evidence (CORE). He is leading a team of data scientists and outcomes researchers to generate real-world evidence and insights through innovative and advanced analytics. John has more than 20 years of experience in biomedical and clinical research across academic, biotech, and pharmaceutical settings. He received his medical training from China Medical University and his medical informatics training from Harvard Medical School. He has co-authored peer-reviewed publications in the areas of medical informatics, machine learning, clinical trials, and cancer genomics. John is a Fellow of the American Medical Informatics Association (AMIA), and also serves in the AMIA Industry Advisory Council.