Seeing Firsthand The Transformative Impact Of AI On Pharmacovigilance And Clinical Research
By Aashna Goyal and Guriqbal Singh, RegWeb Consulting Services Inc., Canada
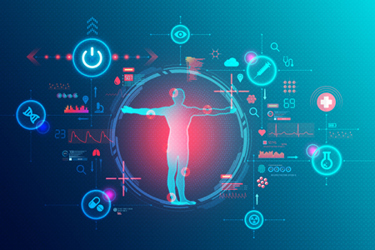
Pharmacovigilance plays a crucial role in ensuring the safety and efficacy of pharmaceutical products by detecting, assessing, and preventing adverse drug reactions (ADRs). Clinical research has long been the backbone of medical advancements, providing the necessary evidence to approve new therapies and improve existing ones. Both fields are data-intensive, requiring high accuracy and robust methodologies. However, with the exponential growth in data and the increasing complexity of healthcare, traditional methods are becoming increasingly insufficient to handle the volume, variety, and velocity of information generated today.
In recent years, AI has emerged as a powerful tool to address these challenges. AI encompasses a range of technologies, including machine learning (ML), natural language processing (NLP), and data mining, all of which can process vast amounts of data faster and more accurately than human counterparts. This paper explores the profound impact of AI on pharmacovigilance and clinical research, as well as how we have used it at RegWeb.
AI In Pharmacovigilance: Revolutionizing Drug Safety
Applications Of AI/ML In Data Management For Pharmacovigilance
In our experience, we have seen how AI and ML tools, like ARIS G and ARGUS, have significantly enhanced case intake and processing efficiency. ARIS G, with its ML algorithms, automates the identification of duplicate cases, ensuring only unique cases are processed, which greatly reduces manual review time and improves data integrity. Additionally, it aids in anomaly detection, offering an effective alternative to traditional quality assurance methods.
We’ve also worked extensively with ARGUS Automation, which has streamlined case management tasks. Its automation features help process adverse event reports swiftly and flag inconsistencies that might be overlooked in manual systems. For instance, ARGUS prioritizes cases based on severity, reducing human error and enhancing timely reporting.
Furthermore, AI/ML within ARIS G and ARGUS have facilitated more thorough causality assessments in individual case safety reports (ICSRs), improving our ability to identify potential safety concerns early and ensuring more accurate and efficient regulatory reporting.
Automated Signal Detection And Case Processing
Pharmacovigilance involves monitoring, collecting, and analyzing data related to drug safety. AI, particularly ML, streamlines signal detection — the identification of potential safety concerns from vast data sources such as clinical trials, EHRs, and spontaneous reports. We have observed how the integration of ML tools in signal detection improved the speed and accuracy of identifying potential safety signals. By automating the classification and extraction of data from unstructured sources like medical records and patient reports, we can detect adverse events with much greater efficiency than by using traditional methods.
In one instance, leveraging these AI tools allowed us to identify an emerging safety issue significantly faster, enabling the team to respond promptly and mitigate any risks associated with the drug. This capability is invaluable in ensuring patient safety and reducing the time it takes to react to potential adverse drug reactions.
Predictive Analytics For Risk Management
AI’s predictive capabilities offer immense value in risk management by forecasting potential safety issues. Through ML algorithms, we can analyze historical adverse event data, identify risk factors, and predict the likelihood of future adverse reactions. In our experience, using predictive models has been particularly helpful in identifying safety concerns early in the drug approval process, thereby reducing risks associated with both pre-market and post-launch phases. This proactive approach to risk management helps safeguard patients and provides more accurate forecasts of safety issues.
Enhanced Data Integration And Real-Time Monitoring
AI facilitates real-time monitoring of drug safety across global populations by integrating data from multiple sources such as social media, EHRs, and pharmacovigilance databases. We have worked with AI-driven platforms that analyzed large volumes of structured and unstructured data in real time, enabling us to detect emerging safety trends sooner. By integrating data from various sources, AI provided a comprehensive view of a drug’s safety profile, helping us identify previously unnoticed ADRs. This ability to monitor safety in real time is crucial for timely interventions and maintaining patient safety.
AI In Clinical Research: Accelerating Drug Development
While AI has transformed pharmacovigilance, it is also reshaping clinical research by improving trial design and patient recruitment.
Faster Patient Recruitment And Enrollment
One of the major bottlenecks in clinical research is patient recruitment. Traditional recruitment methods are time-consuming and often fail to meet enrollment targets. AI is transforming patient recruitment by using ML algorithms to analyze EHRs, genomic data, and other patient data sources to identify suitable candidates for clinical trials. In our work, we’ve seen how AI can analyze vast data sets quickly, ensuring we recruit the right patients for clinical studies. This data-driven approach reduces recruitment time and increases the likelihood of enrolling patients who meet the study’s specific criteria, ultimately enhancing the efficiency of clinical trials.
Optimizing Clinical Trial Design
AI technologies can revolutionize clinical trial design by enabling the development of adaptive and personalized trial protocols. In our experience, ML models that simulate different trial scenarios and predict patient responses have been invaluable in optimizing clinical trial designs. These tools help identify the most appropriate biomarkers for patient stratification, select optimal dosing regimens, and define better endpoints. By refining these aspects of trial design, AI not only improves trial efficiency but also increases the likelihood of achieving meaningful clinical outcomes.
Real-Time Data Analysis And Decision-Making
Clinical trials generate massive amounts of data, and timely analysis is critical for decision-making. AI-powered data analytics platforms allow real-time analysis of clinical trial data, helping researchers identify trends and insights sooner. In one trial we supported, AI-driven platforms enabled us to analyze patient data in near real time, reducing the time it took to make informed decisions. This accelerated decision-making process ensured that any issues with the trial, such as safety concerns or inefficiencies, were promptly addressed, helping to keep the trial on track.
Clinical Trial Monitoring And Compliance
AI-based monitoring systems oversee clinical trial integrity and compliance, ensuring adherence to regulatory standards such as ICH-GCP guidelines. In our experience, ML algorithms have been crucial in tracking trial progress, identifying protocol deviations, and assessing the likelihood of success or failure in various trial phases. These AI tools also automate reporting and documentation processes, reducing human error and ensuring that clinical trials meet the highest regulatory standards.
Ethical And Regulatory Considerations
The integration of AI into pharmacovigilance and clinical research introduces significant ethical and regulatory challenges. Ensuring the privacy and security of patient data is paramount, and as AI systems become more involved in sensitive healthcare data, questions regarding data ownership and consent arise. In our work, we’ve been involved in ensuring that AI applications comply with both ethical and regulatory guidelines. One of the key challenges has been maintaining transparency in decision-making algorithms, as these tools become increasingly autonomous. Regulatory agencies, such as the FDA and EMA, are working to establish frameworks to govern the use of AI in healthcare. It is crucial to ensure that AI tools are used responsibly, maintaining both human oversight and regulatory compliance.
Conclusion
AI is transforming pharmacovigilance and clinical research by improving data processing, enhancing decision-making, and accelerating drug development. In our experience, these technologies have had a profound impact on both the speed and accuracy of drug safety monitoring and clinical trials. As AI technologies continue to evolve, regulatory frameworks must adapt to ensure these innovations are harnessed ethically and responsibly. Looking ahead, AI holds the potential not only to enhance clinical research and pharmacovigilance efficiency but also to significantly impact long-term drug safety and patient outcomes. By enabling more precise risk management, early detection of adverse effects, and personalized treatment strategies, AI can contribute to safer, more effective therapies, shaping a future of healthcare that is both proactive and patient-centered.
References:
- Bate, A., & Stegmann, J.-U. (2023). Artificial intelligence and pharmacovigilance: What is happening, what could happen, and what should happen? Health Policy and Technology, 12(2), 100743. https://doi.org/10.1016/j.hlpt.2023.100743.
- Salas M, Petracek J, Yalamanchili P, Aimer O, Kasthuril D, Dhingra S, Junaid T, Bostic T. The Use of Artificial Intelligence in Pharmacovigilance: A Systematic Review of the Literature. Pharmaceut Med. 2022 Oct;36(5):295-306. doi: 10.1007/s40290-022-00441-z
- Desai MK. Artificial intelligence in pharmacovigilance - Opportunities and challenges. Perspect Clin Res. 2024 Jul-Sep;15(3):116-121. doi: 10.4103/picr.picr_290_23.
- Ahire YS, Patil JH, Chordiya HN, Deore RA, Bairagi VA. Advanced Applications of Artificial Intelligence in Pharmacovigilance: Current Trends and Future Perspectives. J Pharm Res. 2024;23(1):23–33.
- Liang L, Hu J, Sun G, Hong N, Wu G, He Y, Li Y, Hao T, Liu L, Gong M. Artificial Intelligence-Based Pharmacovigilance in the Setting of Limited Resources. Drug Saf. 2022 May;45(5):511-519. doi: 10.1007/s40264-022-01170-7.
- Harrer S, Shah P, Antony B, Hu J. Artificial Intelligence for Clinical Trial Design. Trends Pharmacol Sci. 2019 Aug;40(8):577-591. doi: 10.1016/j.tips.2019.05.005.
- Askin S, Burkhalter D, Calado G, El Dakrouni S. Artificial Intelligence Applied to Clinical Trials: Opportunities and Challenges. Health Technol (Berl). 2023;13(2):203-213. doi: 10.1007/s12553-023-00738-2.
- Crossnohere NL, Elsaid M, Paskett J, Bose-Brill S, Bridges JFP. Guidelines for Artificial Intelligence in Medicine: Literature Review and Content Analysis of Frameworks. J Med internet Res. 2022;24(8)
- Gilvary C, Madhukar N, Elkhader J, Elemento O. The Missing Pieces of Artificial Intelligence in Medicine. Trends Pharmacol Sci. 2019 Aug;40(8):555-564. doi: 10.1016/j.tips.2019.06.001. Epub 2019 Jul 2. PMID: 31277839.
- Secinaro S, Calandra D, Secinaro A, et al. The role of artificial intelligence in healthcare: a structured literature review. BMC Med Inform Decis Mak. 2021;21:125. doi: 10.1186/s12911-021-01488-9.
About The Authors:
Aashna Goyal is a pharmacovigilance and regulatory specialist at RegWeb Consulting Services Inc. She holds a master’s degree in life sciences and has experience in the pharmaceutical industry with her primary focus on pharmacovigilance processes. Her expertise spans AE monitoring, maintaining compliance, and project management.
Guriqbal Singh is the founder and director of RegWeb Consulting Services Inc., based in Canada. RegWeb provides solutions for complex submission strategies concerning CMC and Clinical aspects. RegWeb provides end-to-end services for pre-authorization to post-authorization stages of a molecule's life cycle. RegWeb is also involved in advertisement and promotional material review along with systematic literature search based on the Cochrane method.