Tackling AI Copyright Challenges In Pharma And Clinical Research
By Becky Upton, Ph.D., president, Pistoia Alliance
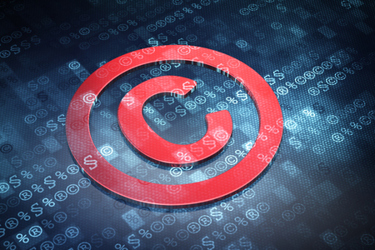
The life sciences industry is rapidly embracing AI, with a recent survey from the Pistoia Alliance finding that 83% of professionals now use generative AI in their workflows. The surge is unsurprising, given AI’s potential to accelerate processes across pharma and clinical research. From streamlining resource-intensive R&D processes such as lead identification and optimization, to powering analysis of complex genetic and real-world patient datasets, AI is increasingly recognized as a powerful tool that enhances human-led work.
However, as AI adoption grows, so do concerns around regulation and compliance. The majority (85%) of respondents cited AI legislation and regulations as a major challenge to adoption and cross-border collaboration. Copyright rules, in particular, are often overlooked or misunderstood. An AI model’s accuracy and trustworthiness depends on it being trained on responsibly sourced, legally compliant data from a mix of sources. Yet the licensing of these datasets might not allow their use in AI training, creating confusion and potential legal risks.
To ensure AI is used safely, securely, and compliantly, pharma and clinical research organizations must collaborate to address copyright and data-sharing challenges — or risk stalling innovation. To explore these challenges, we brought together experts in the field from the Alliance’s membership to highlight key risks and outline best practices for responsible AI adoption.
Why Copyright Compliance Is Critical
AI models are trained on a range of scientific data sources. Dr. Babis Marmanis, who holds a Ph.D. in applied mathematics and scientific computing and serves as chief technology officer at the Copyright Clearance Center, explains, “Generative AI outcomes improve when trained on responsibly sourced, copyrighted works. The training and fine-tuning of AI models in pharma R&D includes high-value scientific literature, and that use entails making and storing copies of these documents. In many cases, with the appropriate prompts, portions or the entirety of the original works can be recalled by end users and derivative or very similar outputs can be created. Without appropriate licenses, this creates copyright infringement risks.”
Our experts broke these risks down into two broad categories — legal and reputational. In addition to copyright infringement, patent infringement is a major concern, warns Chris McSpiritt, vice president of life sciences strategy at Domino Data Lab. Drugs are patented based on their molecular structure; if an AI model suggests a treatment candidate patented by a competitor — perhaps due to its unintentional inclusion in a third-party dataset — this could lead to inadvertent patent infringement. Without safeguards, companies may unknowingly invest in R&D for a product they cannot legally develop. McSpiritt notes that many organizations already have processes for checking patent conflicts, and the introduction of AI reinforces the importance of proficiency in this area.
McSpiritt also cites reputational risk, arguing pharma and clinical organizations rely on trust — both from industry partners and the public. If a company is found to be using copyrighted, patented, or confidential materials without permission, it could face backlash, lose future collaboration opportunities, and erode public confidence in its treatments.
Overall, ensuring compliance and using curated high-quality datasets from the outset not only prevent legal and reputational challenges, but also improve AI-driven research outcomes as Marmanis notes.
AI And Copyright: Three Key Challenges
The Pistoia Alliance’s research identified three hurdles associated with copyright that must be addressed to ensure AI is used compliantly and responsibly in life sciences.
First, there is significant regulatory complexity as AI copyright laws continue to evolve and vary widely across territories. For example, the EU AI Act introduced copyright transparency obligations, which will require AI model providers to publish detailed summaries of the content used to train models. While these regulations aim to increase transparency, they add to the already complex web of compliance requirements for pharma and clinical research organizations working across borders. Additionally, many countries have no legal framework explicitly defining whether AI-generated outputs can be copyrighted. For instance, the U.S. Copyright Office has explained that works created solely by AI without human authorship are not eligible for copyright protection. This ambiguity creates further uncertainty – if AI-generated content cannot be copyrighted, how can companies protect their AI-driven works?
Second, there is confusion around copyright applicability. Of life science professionals polled, 42% do not consider copyright before sharing or using third-party information with AI tools — a gap that could lead to infringement risks. Graeme King, VP and head of data & AI governance at Amino Data, adds that many organizations also do not consider confidentiality rules, particularly related to clinical trial reports and drug performance data.
Third and finally, lack of expertise remains a barrier, with only 40% of organizations reporting to have a dedicated team or expert focused on AI copyright compliance. Specialized knowledge on data licensing, text-mining rights, copyright, and IP legislation are becoming a must, but these skills are hard to acquire and competition for hiring experts is fierce.
Navigating Copyright Compliance: Four Tips From Experts
Experts shared the following advice for organizations to navigate the evolving copyright and AI regulation complexity.
- Know copyright rules for your data and data sources: Will FitzHugh, senior consultant at bPrescient, says, “It’s important for organizations to use appropriately licensed AI models and data sources.” King said examples of data in pharma that might present copyright challenges include literature and patent data, published research papers, conference proceedings, and post-market surveillance data. In clinical research, his examples include electronic health records, imaging libraries, clinical trial results, and real-world evidence.
- Invest in expertise: FitzHugh also notes the importance of consulting with legal teams throughout AI model development and deployment. He says, “Good practices should include making sure that data used for training is not restricted in its use, that the base model is properly licensed.”
- Establish strong governance frameworks: McSpiritt notes the importance of mitigating risk via governance frameworks and auditing source datasets and models. He says these should be added on top of current processes organizations already have for legal and copyright issues.
- Secure and test AI models: FitzHugh argues fine-tuning of all AI models should be conducted in secure local or cloud environments. Rigorous testing helps to validate outputs, assess model performance, and ensure credibility.
Driving Innovation Through Responsible AI
AI and copyright compliance are complex, evolving challenges that require cross-disciplinary collaboration between data scientists, pharma and clinical experts, as well as regulatory and legal specialists. To ensure researchers can responsibly and effectively leverage AI, the industry must work together to develop best practice guides, harmonize data standards, and invest in training around copyright compliance. Those interested in navigating regulatory uncertainty, reducing copyright risk, and unlocking AI’s full potential are invited to join the Pistoia Alliance’s AI Community.
About The Author:
Becky Upton, Ph.D. was appointed as the Pistoia Alliance’s first female president in June 2022. She is a long-time supporter of pre-competitive collaboration in life sciences and healthcare R&D and the critical role it plays in advancing science and is passionate about diversity in STEM. Becky is responsible for leading the Pistoia Alliance’s strategy and defining its future within areas of increasing importance to the industry, such as data standards, emerging technologies, diversity and inclusion, sustainability, and precision medicine. Becky has a Ph.D. in biochemistry from Imperial College and an MBA from Cranfield University.