The Evolution Of Biomarker-driven Approaches To Clinical Trials
By Ketan Patel, Clarivate
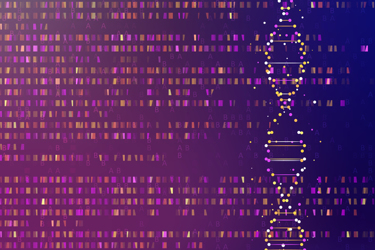
Over the past three decades, the life sciences industry has observed considerable progress in the development and application of precision medicine, particularly in oncology. Greater understanding of the specific and varying genetic bases for cancers has driven the tailored approach and provided greater opportunities for clinical trials to take advantage of patient genetic variability for recruitment and segmentation purposes. However, this can also introduce challenges in clinical trial planning. In this article, historic data provide insights into the evolution and future of biomarker use in precision medicine.
Precision Medicine For Better Oncology Care
At the very basic definition, precision medicine provides the information about what drug to prescribe, at what dose and when, for which patient. The traditional “one-size-fits-all” approach to the development and prescription of treatments has failed many patients. For example, when prescribed outside of the precision medicine tenet, pembrolizumab (Keytruda) helps only 1 in 6 people,1 and lenalidomide (Revlimid) helps only 1 in 3 people.2 For many clinicians, finding the best treatment for a patient is often through trial and error.
Precision medicine focuses on individual variations in genetics as well as differences in environments and lifestyles that will affect the efficacy and safety of a given treatment. With a shift toward a more personalized model, we also gain efficiencies throughout the drug development life cycle (by designing trial protocols that include only those patients most likely to respond) to the clinic (where better care can be provided to the patients in need of treatments).
Role Of Next Generation Sequencing
Next generation sequencing (NGS) technology is considered to have revolutionized genomic research. This DNA sequencing technology has enabled sequencing of an entire human genome in only a day, compared with previous methods, such as the Sanger sequencing technology, which could take more than a decade to provide results. Moreover, NGS can achieve more sensitive results with a smaller sample. With the incredibly fast advancements in NGS technology, its costs have decreased, opening the possibilities for an array of comparisons based on a broad range of factors, including mutations that could not previously be captured.
This greater and cheaper access to NGS testing has expanded its use to clinical laboratories, where gene panel testing has enabled therapy decisions based on genetic variants, particularly in oncology (Table 1). The impact on precision medicine, from risk assessment to early diagnosis, prognosis, and treatment, has been dramatic.
Table 1: Role of NGS to Guide Cancer Therapy3
Exciting capabilities in this space include the ability to simultaneously test all possible mutations in a set of genes that can affect disease outcomes. For example, patients with wild-type KRAS advanced or metastatic colorectal cancer treated by anti-epidermal growth factor receptor (EGFR) antibodies can become resistant to the therapy when activating mutations occur in any of the following: KRAS, NRAS, PIK3CA, BRAF. With NGS, all four genes can be tested simultaneously, with only a small amount of sample, to guide therapy decisions.4
Oncology Biomarker Discoveries
NGS-driven understanding of genes and their role in health and disease along with the increased testing capabilities have expanded the use of biomarkers across the research and care continuum. Biomarkers based on measurable levels of circulating markers in blood, plasma, and serum, as well as those identifiable on imaging, have been used for decades. Genome-based measures provide greater scope to identify individual differences in disease. This becomes particularly important in oncology, where genetic mutations play a central role.
Herceptin (trastuzumab), a monoclonal antibody, was one of the first targeted cancer therapies to be approved by the FDA in 1998 as treatment for HER2-positive metastatic breast cancer as a combination first-line therapy and on its own as second-line therapy. Since then, other drugs targeting the HER2 protein have been developed and approved, including tyrosine kinase inhibitors (TKIs) such as neratinib, lapatinib, and tucatinib. After discovering that other cancers also exhibit HER2 protein overexpression or HER2 gene amplification, the use of these drugs has been expanded.
In the same human epidermal growth factor receptor (ErbB) family as HER2 is EGFR, aka HER1. EGFR is also frequently mutated or overexpressed in many cancers, including glioblastoma, lung cancers, colorectal cancer, head and neck cancer, and breast cancer. EGFR-inhibiting drugs include the TKIs erlotinib, gefitinib, and lapatinib and the monoclonal antibodies cetuximab and panitumumab.
Therapeutic mechanisms are closely linked to the biomarkers HER2, EGFR, KRAS, BRCA1/2, and PD1/PD-L1 for cancers such as breast cancer, non-small cell lung cancer, and ovarian cancer. In addition, 76 biomarkers have been identified as potential indicators of tumor invasiveness, including S100 proteins, annexins, galectins, CATD, TGM2, gelatinases, fibronectins, proteoglycans, TGFBI, FGG, APMAP, and more.5
Biomarker-Driven Therapy And Clinical Trial Decisions In Oncology
With the increasing number of biomarkers, in addition to their greater sophistication, they are commonly being incorporated for disease profiling and for diagnostic, therapeutic, and prognostic purposes in oncology (Figure 1).
Figure 1: Trends in the use of biomarkers for oncology therapy and research (Source: Cortellis Drug Discovery Intelligence)
The percentage of oncology clinical trials using biomarkers has steadily increased over the last 10 years (Figure 2). The slight decrease since 2017 could be partially explained by the increase in studies of immune-oncology drugs at that time; these trials do not require a biomarker because of their universal application.
Figure 2: Trends in the use of biomarkers in oncology clinical trials (Source: Cortellis Clinical Trials Intelligence)
Early introduction of biomarkers in trials can front load drug development with an understanding of the disease biology at the patient level to help with prediction of drug efficacy, dosing, and potential treatment toxicity (Figure 3).
Figure 3: Trends in the use of biomarkers in each phase of oncology clinical trials for trials that started in 2010-2019 (Source: Cortellis Clinical Trials Intelligence)
However, the decrease in biomarker use in Phase 3 trials represents a potential missed opportunity, even for trials in which the drug is expected to benefit all patients. Biomarker use could facilitate stratified analysis later in the study, particularly if efficacy results for the broader population are poorer than expected.
A good example of this is the use of pembrolizumab, an immunoglobulin G4 (IgG4) monoclonal antagonist antibody to PD-1, for treatment of non-small cell lung cancer (NSCLC). Over a series of trials (KEYNOTE), while the initial trials evaluated pembrolizumab in all patients with advanced NSCLC, stratified analysis showed greater benefit in patients with ≥50% tumor PD-L1 expression. This informed later trials designed to evaluate pembrolizumab as first-line therapy, a designation it was granted 2016; at that point, it was the only anti-PD-1 therapy to be approved in the first-line treatment setting for patients with advanced NSCLC and high PD-L1 expression. Nivolumab, another IgG4 monoclonal antagonist antibody to PD-1, was being evaluated at the same time as a potential treatment for NSCLC. However, stratification was not performed, and its approval as first-line treatment (+ ipilimumab) for patients with advanced NSCLC with ≥1% tumor PD-L1 expression only just occurred in May 2020.
Patient Stratification
Trial efficiencies can also be gained by designing a protocol that uses biomarkers to inform patient recruitment or to stratify patients during enrollment — to ensure a good patient-therapy match and reduce the attrition rate. When we know how to select patients for a given clinical trial, required sample sizes decrease, reducing the time to get the drug to market and increasing the success rates. This is especially true for oncology trials, for which the probability of success increases to 10.7% with biomarker-led stratification compared with 1.6% without biomarker-led stratification.6
Enriching the population in this way should increase the proportion of responders, ultimately reducing the required sample size and speeding recruitment. An example of this is a Phase 3 trial of crizotinib, an oral ALK inhibitor in patients with ALK-positive non-small cell lung cancer. With a response rate of 85%, only 255 patients are required, which is significantly fewer than the ~2,000 patients required if the response rate was 24% (Figure 4). This has also been shown broadly across oncology clinical trials with biomarker-led patient selection, in which higher response rates in early trials were associated with shorter drug development times.7
Figure 4: Reduction in the sample size with biomarker-led patient recruitment
The approval of crizotinib to treat ALK-positive NSCLC just three years after the ALK rearrangement was reported as a target in lung cancer is an example of the feasibility and efficiency of a personalized approach (Figure 5).8 Moreover, ceritinib, another ALK inhibitor, was granted accelerated approval after Phase 1 testing (42.7 months) as second-line treatment in patients with ALK-positive NSCLC after treatment with crizotinib,9 and pembrolizumab (anti-PD-1) was also approved after Phase 1 testing as second-line treatment in patients with BRAF V600-positive melanoma after treatment with ipilimumab and a BRAF inhibitor.10 This is a marked improvement from the historical timelines for developing targeted therapies. For example, BCR-ABL inhibition for chronic myeloid leukemia (CML) was discovered in 1960 but was not approved until 2001, 41 years later and six to eight years after the patents were filed.
Figure 5: Time to market from initial identification of a specific drug target11
Furthermore, a recent study reported significantly faster clinical development phase times when using biomarker-based strategies for oncology drug development: median of 58.8 months vs. 93.5 months for non-personalized drugs (P=.001).7 The shortest total clinical development timelines were observed for drugs that targeted a specific cancer population based on molecular characteristics: imatinib for CML (Bcr/Abl translocation; 36 months); ceritinib, as already mentioned; and dabrafenib for BRAF-positive melanoma (47.1 months). In addition to benefiting patients with earlier access to effective treatments, faster times to market can maximize the patent-limited sales period.
Although the use of biomarkers for patient segmentation in oncology trials is increasing and still significantly greater than in other therapeutic areas, the number of trials using genetic biomarkers for this purpose (e.g., BRAF, EGFR mutations) still only represents a small proportion of trials (6% of trials starting in 2019; Figure 6).
Figure 6: Trends in the use of patient segmentation genetic biomarkers in oncology clinical trials (Source: Cortellis Clinical Trials Intelligence)
This represents a potentially missed opportunity to shorten clinical trial timelines based on patient segmentation, an aim that is particularly important for oncology trials given that the median duration from Phase 1 to approval is 13.1 years, compared with 5.9 to 7.2 years for other therapeutic areas.6
Toxicogenomics
Early discovery of potentially toxic effects of drugs in specific patient subsets through toxicogenomics can also help development decisions and speed time to market — by either narrowing the patient population for clinical studies or changing drug targets to avoid the toxic effects.12 Toxicogenomics supports the early assessment of the influence of gene variants on the risk for adverse events, which would help determine the maximum tolerated dose for a given individual. A well-known example of the influence of genetic variability on drug-induced adverse events is the observed cardiotoxicity in women receiving doxorubicin chemotherapy, which is exacerbated when used in combination with trastuzumab.13
Changes In Trial Design
Clinical trial designs are also adapting. To speed the time to market, a growing number of trials are being designed to combine phases or bypass the traditional pathway. One method is to use a master protocol framework,14 which according to the FDA “uses a single infrastructure, trial design, and protocol to simultaneously evaluate multiple drugs and/or disease populations in multiple sub-studies, allowing for efficient and accelerated drug development.”15 Essentially, these designs avoid the need to develop a new protocol to test each drug or subpopulation and provide the opportunity to share a control arm or centralize data capture, contributing to greater flexibility and efficiency.
The designs follow the tenets of precision medicine by identifying those most likely to respond to a given therapy based on individual variability in genes, environment, and lifestyle. A basket trial is one in which the effect of a single therapy is investigated in multiple populations defined by disease, disease stage, number of prior therapies, genetic or other biomarkers, or demographic characteristics.14-16 Umbrella trials investigate multiple therapies, either alone or in combination, in a single population that is divided into multiple subgroups based on disease stage, number of prior therapies, genetic or other biomarkers, or demographic characteristics.
Opportunities Remain For Biomarker Use
Analysis of aggregated data from multiple sources has provided broad insights into the current precision medicine and associated clinical trial landscape, enabling data-driven strategic planning for future clinical trials. Oncology has been the leading therapeutic area in terms of biomarker development and use, setting the stage for biomarker adoption in other therapeutic areas. This includes new and adaptive designs focused on precision medicine.
Great progress has been made in precision medicine, providing patients with more effective treatments. Yet, opportunities remain to incorporate biomarkers, and particularly biomarker-based stratification, into oncology clinical trials to shorten trial durations and increase the chances of successful outcomes.
References:
- Keytruda. Selected safety information. 2020 Available at https://www.keytrudahcp.com/melanoma/adjuvant-therapy-clinical-study-results/ Accessed September 28, 2020.
- Kaura S, Dranitsaris G. Number needed to treat (NNT) as a measure of drug benefit: Lenalidomide versus bortezomib for treatment of relapsed/refractory multiple myeloma (MM). Journal of Clinical Oncology 2012;30(15_suppl) doi 10.1200/jco.2012.30.15_suppl.e18562
- Wakai T, Prasoon P, Hirose Y, et al. Next-generation sequencing-based clinical sequencing: toward precision medicine in solid tumors. Int J Clin Oncol. 2019;24:115-122.
- Dong L, Wang W, Li A, et al. Clinical Next Generation Sequencing for Precision Medicine in Cancer. Curr Genomics 2015;16(4):253-263. doi: 10.2174/1389202915666150511205313
- Pouliquen DL, Boissard A, Coqueret O, et al. Biomarkers of tumor invasiveness in proteomics (Review) Int J Oncol 2020;57(2):409-432. doi: 10.3892/ijo.2020.5075
- Wong CH, Siah KW, Lo AW. Estimation of clinical trial success rates and related parameters. Biostatistics. 2019;20(2): 273–286. doi: 10.1093/biostatistics/kxx069
- Jardim DL, Schwaederle M, Hong DS, et al. An appraisal of drug development timelines in the Era of precision oncology. Oncotarget 2016;7(33):53037–53046. doi: 10.18632/oncotarget.10588
- Gerber DE, Minna JD. ALK inhibition for non-small cell lung cancer: from discovery to therapy in record time. Cancer Cell 2010;18(6):548-551. doi: 10.1016/j.ccr.2010.11.033
- Dhillon S, Clark M. Ceritinib: first global approval. Drugs 2014;74:1285-1291.
- Poole RM. Pembrolizumab: first global approval. Drugs 2014;74(16):1973-1981.
- BCR-ABL inhibition Gleevec. Nature Medicine 2011;17(3).
- Clarivate. Best practices in toxicology: Current perspectives for enhancing drug safety. https://clarivate.com/cortellis/campaigns/how-can-we-improve-identification-of-toxicology-related-risks-in-drug-discovery/
- Schneider BP, Shen F, Gardner L, et al. Genome-Wide Association Study for Anthracycline-Induced Congestive Heart Failure. Clin Cancer Res. 2017;23(1):43-51.
- Hu C, Dignam JJ. Biomarker-driven oncology clinical trials: key design elements, types, features, and practical considerations. JCO Precis Oncol 2019. doi: 10.1200/PO.19.00086
- Food and Drug Administration. Master Protocols: Efficient Clinical Trial Design Strategies to Expedite Development of Oncology Drugs and Biologics: Draft Guidance for Industry. October 2018. https://www.fda.gov/regulatory-information/search-fda-guidance-documents/master-protocols-efficient-clinical-trial-design-strategies-expedite-development-oncology-drugs-and
- Park JJH, Hsu G, Siden EG, et al. An overview of precision oncology basket and umbrella trials for clinicians. Cancer Journal for Clinicians. 2020;70(2):125-137. doi: 10.3322/caac.21600List
About The Author:
Ketan Patel’s background spans 15 years of experience working in industry at Lilly, Pfizer, Oracle HSGBU, and most recently at AstraZeneca. Over the years, he has led and worked on computational and data science projects in the industry, from early stage drug discovery through to clinical development, product launch, and medical affairs. Patel is currently VP of the Cortellis Product Platform at Clarivate, where he leads the development of products that provide analytics and insights from discovery through to development and regulatory approval.