The Risks Of AI In Clinical Research From A Trial Management Perspective
By Donatella Ballerini, GCP consultant
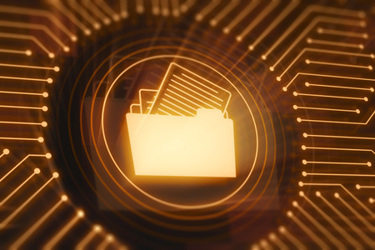
While AI presents remarkable opportunities for efficiency and innovation in clinical research, including eTMF management, it is not without risks. From hallucinations (false or misleading information) to data privacy concerns, research professionals must use AI responsibly, especially when handling regulated, high-stakes clinical trial documentation.
Understanding these risks is not about rejecting AI but about ensuring that its use remains ethical, compliant, and supervised. In part one of this series, “How AI Models Can Actually Improve eTMF Management,” we explored how AI is shaping eTMF management. Now, in part two, we’ll explore the key challenges of integrating AI into eTMF management and how to mitigate them.
Reliability And Accuracy — When AI Hallucinates
The first time I read about AI hallucinations, I was struck by how both powerful and fragile this technology can be. AI models don’t “lie” intentionally, but they can generate plausible-sounding yet incorrect information — a risk that cannot be ignored in regulated industries like clinical research.
For example: Imagine asking ChatGPT to suggest a filing location for a regulatory submission. If the AI incorrectly classifies the record, blindly trusting its answer could lead to compliance findings and quality issues.
So how do we mitigate this? With critical thinking. AI is a tool not an authority. Users must always verify AI-generated recommendations. One way to do this is through prompt refinement, which involves adapting your questions or command to provide to the AI model, which in turn can significantly impact the quality of the response.
Having taken multiple courses on prompt engineering, I find it fascinating how minor tweaks in phrasing can yield vastly different outputs. The secret to maximizing AI’s value lies in asking precise, well-structured questions—a skill that combines creativity and logic.
Take this example of a too-vague prompt: “Where should I file this TMF document?” With such a vague prompt, the AI model could file the essential record, such as a protocol deviation, in the wrong zone, section, and even artifact of the TMF Reference Model, making it unretrievable or anyway improperly indexed. A more refined, structured prompt would be: “Based on the latest version of the TMF Reference Model, under which artifact category should a protocol deviation notification from a site be filed?” By improving how we interact with AI, we can reduce errors and enhance the reliability of AI-assisted processes.
Data Privacy And Security Risks — The Compliance Minefield
Some clinical trial records contain patient data and proprietary study information. This makes data privacy and security a top concern when using AI tools. Any AI model handling clinical trial documentation must comply with strict regulations, including:
- GDPR (General Data Protection Regulation) – Governs data privacy in the EU.
- HIPAA (Health Insurance Portability and Accountability Act) – Ensures patient data protection in the U.S.
- 21 CFR Part 11 – Regulates electronic records and signatures in FDA-regulated research.
In addition to complying with the above regulations, users should also be aware of and avoid allowing the model unauthorized access to patient data. AI tools must not process or store identifiable patient information without encryption and access controls. Coupled with this is a concern over data leakage.
Content generated by AI in a secure environment, and that might contain company data, proprietary data, or personal information, should never be copy-pasted into public AI models without ensuring confidentiality.
Safe use of AI for clinical research includes using secure, enterprise AI models that comply with clinical data protection laws, avoiding sharing confidential or patient-related information in prompts, and ensuring access controls and audit trails for AI-assisted documentation processes.
Accuracy and Reliability – AI’s Knowledge Gaps
AI is trained on large datasets, but its knowledge is not infinite or infallible. This creates two major risks: regulatory misinterpretations and incorrect classifications. With regulatory misinterpretations, an AI model may oversimplify or misinterpret evolving regulatory guidelines. And incorrect record classifications may occur if an AI model is not fine-tuned for clinical research; it may suggest the wrong TMF artifact.
To address these risks, users should always verify AI-generated recommendations against official regulatory sources (e.g., ICH-GCP, EMA, FDA guidance, CDISC TMF Reference Model), continuously update AI training models with the latest clinical research regulations, and maintain human oversight to correct AI errors before they become compliance risks.
Regulatory And Ethical Considerations — Humans Must Have Oversight
Regulatory agencies, including the FDA and EMA, are closely monitoring AI adoption in clinical research. As AI-driven automation becomes more prevalent, it must align with ethical and compliance standards. Therefore, users should focus on three core competencies in its use: transparency, oversight, and bias mitigation.
For transparency internally and externally, AI-generated content must be reviewed and validated before being used in official records. Along the same lines, use of AI should always involve human oversight; an AI model should enhance decision-making, not replace human expertise. Finally, to address and reduce bias, users should train AI models on diverse and unbiased datasets to prevent skewed risk assessments.
Best practices for addressing regulatory and ethical concerns include supporting any AI-assisted process with a “human-in-the-loop” approach, where AI suggestions are reviewed, adjusted, and approved before being finalized in clinical trial documentation. AI is neither a magic bullet nor a threat — it is a powerful tool that, when used responsibly, can transform eTMF management and clinical documentation. The key to leveraging AI effectively is understanding its risks and implementing safeguards to mitigate them.
AI Is A Powerful Ally, Not A Human Replacement
AI is actively reshaping clinical trial management, optimizing efficiency, and enhancing inspection readiness. However, its true value lies not in replacing human expertise, but in augmenting it, allowing professionals to shift their focus from routine tasks to strategic decision-making and innovation.
The future of AI in eTMF management is not about automation alone but about collaboration. AI-driven tools can streamline documentation, flag compliance risks, and enhance data accessibility, but human oversight remains irreplaceable. By striking the right balance between technology and expertise, the clinical research industry can accelerate drug development, improve regulatory compliance, and, most importantly, bring life-changing treatments to patients faster.
About The Author:
With 17 years of experience in the pharma industry, Donatella Ballerini first gained expertise at Chiesi Farmaceutici in the global clinical development department, focusing on clinical studies in rare disease and neonatology. Later, in global rare disease, Donatella served as a document and training manager, where she developed and implemented documentation management processes, leading the transition from paper to eTMF. In 2020, she became the Head of the GCP Compliance and Clinical Trial Administration Unit at Chiesi, ensuring all clinical operations processes complied with ICH-GCP standards and maintained inspection readiness. In 2021, she joined Montrium as the head of eTMF Services, where she helps pharmaceutical companies in eTMF implementation and process improvement, and also works as an independent GCP consultant. Donatella has been a member of the CDISC TMF Reference Model Education Governance Committee since 2023 and the CDISC Risk White Paper Initiative since 2024.