The 5Vs Of Collecting Clinical Data
By Steve Chartier, Society for Clinical Data Management; Patrick Nadolny, global head, clinical data management, Sanofi; and Richard Young, Society for Clinical Data Management
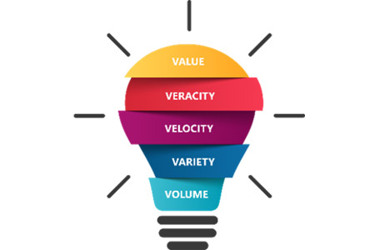
The evolution of clinical research and supporting regulations, as well as massive advances in technology have fundamentally changed what clinical data is. As we define the future beyond traditional electronic data capture (EDC), we need to rethink our approaches and understand how the “5 Vs” of data are reshaping clinical data management (CDM).
First and foremost, not all data is created equal. Therefore, our data strategies need to be commensurate with the risks, complexity, and value of the data collected. Additionally, data security and personal data protection are key elements that must be strategically anticipated. If the true value of this data is to be realized, it must be collected and captured in a consistent and timely manner that considers all five “V” dimensions: volume, variety, velocity, veracity, and value.
Volume
In 2012, Tufts1 estimated that on average, Phase 3 studies collected close to 1 million data points. Today, we measure mHealth data points in the billions. This dramatic increase demands the adoption of new strategies to improve the collection, processing, and archiving of data supporting this new scale. CDM must re-imagine its practices to efficiently move from managing a few data points per case report form (CRF) to managing more than tens of thousands of data points generated per patient per week.
Figure 1 shows the expected volume of actigraphy data generated by wearables (in blue) compared to data generated from site visits (in orange), which is barely visible on the figure by comparison. The protocol requires 260 patients to be treated for six months. The enrollment period is estimated to last six months. With wearable devices set to transmit data every minute, wearables would generate a pulse reading more than 68 million times throughout the study, with a spike at almost 375,000 readings per day. In comparison, pulse would only be generated 3,380 times through site visits, assuming patients visited every two weeks, with at most 260 readings in a week across patients.
With the incredible increase in data volume, CDM must be diligent and secure, using quality by design (QbD) to define what really needs to be collected to support the protocol hypothesis vs. all data that can be generated through new technologies. Not all data generated by devices may be useful for statistical or further exploratory analysis. In the case of wearables, CDM may consider retaining the 68 million pulse readings as e-Source data while only retrieving data summaries at regular intervals (e.g., every hour or day). Data collected may only include key data characteristics (e.g., min, max, average, standard deviation, number of observations generated, etc.), aggregated (e.g., by hour) to better support downstream activities such as safety monitoring, data review and statistical analysis.
Fig 1. Daily volume of actigraphy data from wearable vs. weekly e-CRF pulse data
Variety
With more than 200 new health apps added to app stores every day,2 it is not surprising that sponsors are increasingly using digital health technologies in clinical research and leveraging apps to collect a variety of data, including reported outcomes and other real-world data (RWD). However, most experiments with digital health have been confined to Phase 4 trials, reflecting the perceived risk of incorporating digital measures into pivotal trials until they are validated and pressure tested.
This is unfortunate, as those technologies can improve the efficiency of clinical research in many ways. Solutions for identifying sites, targeting and recruiting the right patients, collecting reported outcomes, gaining digital consent, screening patients remotely, and conducting decentralized trials have all proven to be effective and useful. First and foremost, they benefit patients by removing enrollment barriers and enabling breakthrough medical advances, especially for rare diseases. As clearly seen during the COVID-19 pandemic, patient-centric solutions such as telemedicine and home nursing also benefit sponsors by reducing on-site activities, optimizing site selection, speeding up enrollment, easing data collection, and supporting rapid decision-making through immediate access to data.
To manage this growing volume and variety of data, we must develop new clinical data science (CDS) principles as an evolution from traditional CDM including, new data collection tools, and data review and data analytics strategies. As an example, patient-centric data collected as eSource is almost impossible to modify once they have been generated. This means that feedback on the data quality and integrity of this variety of eSources needs to be provided at the time of data generation. After data is generated, CDM will rarely be able to send a query to request its correction. So, remaining data anomalies will likely need to be tagged and explained. However, will data tagging be enough to deliver reliable data to reach sound conclusions required for regulatory approval? Beyond data tagging, the U.K. Medicines & Healthcare products Regulatory Agency (MHRA) introduced the concept of “data exclusion.”3 This means that “unreliable data” with a potential of impacting the reliability of the trial results could be excluded based on a “valid scientific justification, that the data are not representative of the quantity measured.”3
Additionally, in accordance with good recordkeeping and to allow the inspection and reconstruction of the original data, “all data (even if excluded) should be retained with the original data and be available for review in a format that allows the validity of the decision to exclude the data to be confirmed.”3 Even if not widely used yet, data tagging and exclusion may become a standard practice within CDM to support the generalization of eSource and DCTs.
Furthermore, CDM is tasked with integrating both structured and unstructured data from a wide range of sources and transforming them into useful information. Integrating, managing, and interpreting new data types such as genomic, video, RWD, and sequenced information from sensors and wearables requires new data strategies and questions the centricity of traditional EDC systems. The key questions are where and how, both logically and physically, should these disparate data sources be orchestrated to shorten the gap between data generation to data interpretation?
Additionally, even though not new, the implementation of audit trail review (ATR) is gaining momentum in supporting study monitoring. This is also fueled by the more frequent focus on audit trails from GCP Inspectors. Those can provide critical insights on how the data is being collected, leading to the identification of process improvements or lack of understanding of the protocol instructions, up to the rare cases of manipulation from data originators.4
Velocity
To support real-time data access, we need to understand, prioritize, and synchronize data transactions into appropriate data storage at increased volume, speed, and frequency. Data from wearables for instance, can be generated 24 hours a day, seven days a week. Taking the example in Figure 1, up to 375,000 pulse readings could be generated in a day assuming data is transmitted every minute. This would grow to 22.5 million pulses with data transmitted every second. In a world where real-time data is expected, it is not surprising that connectivity has become a core component of software development.
Application programming interfaces (API), used for web-based systems, operating systems, database systems, computer hardware, mHealth, and software libraries, are enabling automated connectivity in new ways. This is moving the focus from data transfer to data integration. The integration of a high volume and variety of data at high velocity is technically possible, but is it necessary? So, CDM should evaluate the pros and cons for every data integration. We also need to stretch our thinking and expectations, because APIs do not just connect researchers, they provide a platform for automation.
Regardless of the data acquisition and integration technology being used, we need to synchronize the data flow velocity to our needs across all data streams. As a patient’s data is highly related to one another, we need to review and correlate multiple data sources simultaneously. As an example, it would not make sense to reconcile two data sources extracted months apart.
Fig 2a. Data transfer frequency vs. data reconciliation/consolidation
Referring to the simple theoretical in Fig. 2a, the data could be synchronized and therefore optimally reconciled only every two weeks. As shown in Fig. 2b, changing the eCOA transfer frequency from every other day to three times a week would at least enable weekly optimum data reconciliations and reduce the data refresh workload.
Fig. 2b. Data transfer frequency vs. data reconciliation/consolidation
The need for data synchronization would be true for all other data-driven activities, including ongoing data and safety reviews, risk assessments, etc. Synchronizing data flows would prevent rework resulting from data refresh misalignments. Additionally, moving forward, synchronizing data velocity will be more frequently driven by remote working practices. How we integrate data cleaning into site-driven workflows is playing a critical role in our ability to be agile. As an example, performing source data verification (SDV) during the COVID-19 pandemic forced us to look at alternative solutions to remotely synchronize data, documents, and processes with the sudden loss of physical access to sites. These new remote practices will in future require CDS to explore new data review capabilities with sites, beyond simple query-based clarifications.
Veracity
We can associate veracity with the key attributes of data integrity and particularly ALCOA+ (Attributable, Legible, Contemporaneous, Original, Accurate, Complete, Consistent, Enduring, and Available). Veracity also can be associated with some of the attributes of data quality such as data conformity, credibility, and reliability. In this context, CDS needs to establish proactive measures to secure the authenticity and security of the data. This is becoming critical in the world of e-Source and RWD, where data can rarely be corrected and where anonymization is increasingly challenging and critical.
First, we must not let perfection become the enemy of the good, especially where “good” is fit for purpose and good enough. If veracity maps a journey toward fit-for-purpose, we must assess how far we pursue perfection for each data type. Directionally, the concept of quality tolerance limits (QTLs) is a good example of a fit-for-purpose and measurable quality framework that can be used across data streams. Additionally, with the adoption of risk-based approaches, not all data may be subject to the same level of scrutiny. Different quality targets may be acceptable across different data types and sources. CDS will need to not only manage data but also determine and enforce fit-for-purpose data quality standards. In this setting, we can define a positive and a negative goal for data veracity. Positively, we can aim to deliver data veracity not to exceed a set tolerance limit (e.g., not exceed x% of missing data). We also can assign a negative target, where we attempt to remove any issues (e.g., address all missing data) that would alter the end analysis. It is often the case that this latter goal (“negative”) will be easier to define in our cleaning strategy, as defining a quality target requires historical information and may be perceived as subjective. However, trying to eliminate all data issues may be neither attainable nor desired for non-critical data. So, CDS must learn how to set up measurable and objective quality targets by truly representing our data veracity objectives.
Additionally, It is no longer possible to use manual processes based on listings or patient profiles to confirm data veracity from such a large volume of disparate data coming at such high velocity. It is necessary to implement different strategies moving beyond data filtering and trending to strategies based on storytelling visualizations and statistical and machine learning (ML) models, as well as leveraging intelligent automations. Interrogating such data may require different technology expertise, such as NoSQL (Not only Structured Query Language) or semantic automation.
Eventually, we also will need to secure the veracity of data on systems that we do not directly control, such as EHRs with their disparate and complex data structures, like genomic data, medical imaging, unstructured data and documents, metadata, and sequenced data.
Value
Importantly, CDM needs to maximize the relative value of any one data point in this ocean of data. In the current CDM context, we value quality data enabling the reliable interpretation of the trial’s results. In the context of CDS, the value of data goes beyond integrity and quality to ensure its interpretability. To leverage the full potential of the data we have, we must look beyond its original purpose. During a clinical trial, we collect data to validate the hypothesis of the protocol and, ultimately, obtain market authorizations. Once databases have been locked, most pharmaceutical companies will only reuse them for regulatory purposes (e.g., annual safety updates, integrated efficacy and safety summaries, market authorization in other countries, etc.).
Figure 3. The data value extraction journey
However, to unleash the full value of clinical trial data, sponsors must proactively anticipate what will be needed in the future. It means that we need to seek patient authorization up front, through unambiguous informed consent forms, to use their data for purposes other than the scope of the protocol. Some companies are beginning to reuse clinical and health data in new ways, influencing others to seriously consider it.
Examples include:
- Creating synthetic arms either from past clinical trials or from RWD
- Engaging and retaining patients by feeding them study-wide data summaries during study conduct
- Creating machine learning training data sets to improve operational processes such as automating query detection or enhancing the reliability and accuracy of endpoint assessments
- Extracting real-world evidence from real-world data to gain insights on how to improve standard of care or better understand drug effectiveness in a real-world setting
At the end of the day, through the application of proven data strategies, we can leverage emerging technologies to extract the full value of data for all stake holders (i.e., patients, sites, sponsors, regulators, caregivers, and payers) and defeat data silos, the enemy of our value extraction journey.
Figure 4. The 5Vs data journey from collection to value generation
References
- Tufts, November 2012, Clinical Trial Complexity, available at https://www.nationalacademies.org/documents/embed/link/LF2255DA3DD1C41C0A42D3BEF09
89ACAECE3053A6A9B/file/D774AC7AEFEE0E80425328941C08CF3E6CE97D0BF748 - IQVIA, November 2017, The Growing Value of Digital Health: Evidence and Impact on Human Health and the Healthcare System. Available at https://www.iqvia.com/insights/the-iqvia-institute/reports/the-growing-value-of-digital-health
- MHRA, March 2018, ‘GXP’ Data Integrity Guidance and Definition, Available at https://assets.publishing.service.gov.uk/government/uploads/system/uploads/attachment_data/
file/687246/MHRA_GxP_data_integrity_guide_March_edited_Final.pdf - SCDM and eCF, Audit Trail Review, An Industry Position Paper on the use of Audit Trail Review as a key tool to ensure data integrity Available at https://scdm.org/wp-content/uploads/2021/04/2021-eCF_SCDM-ATR-Industry-Position-Paper-Version-PR1-2.pdf
About The Authors:
Steve Chartier is a contributing author for the Society for Clinical Data Management (SCDM) innovation committee, as well as executive director of data engineering at LogixHealth. Chartier has 35 years of technology experience with almost 20 years across life sciences and CROs. Chartier is a passionate about data strategy and analytics and is an expert in building and nurturing technology organizations to deliver state-of-the-art products data products and services to enable data-driven decisions.
Patrick Nadolny has almost 30 years of industry experience across pharmaceutical, device and biologics as well as technology solution development. He is a pragmatic leader focusing on technology, innovation, strategic planning, change management, and the setup of new capabilities. Nadolny is the global head of clinical data management at Sanofi. In addition to his SCDM board member role, he leads the SCDM innovation committee, which released many papers on the evolution of clinical data management toward clinical data science.
Richard Young is an SCDM committee member and the vice president, Strategy Vault CDMS, at Veeva Systems.