5 Ways AI Is Changing Clinical Research — And How To Embrace It
By Bryce Nichols
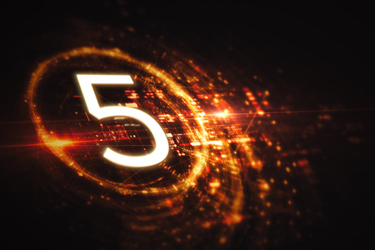
Clinical research isn’t preparing for AI; it’s already being shaped by it. From trial design to patient reminders, AI is quietly becoming infrastructure. It’s reshaping workflows: speeding up processes, automating the mundane, and helping trials adapt around patients. By staying current and mindful of use, we can strive to truly augment skilled workers and not replace them.
This article outlines how AI is currently transforming clinical trials, introduces real tools already in use, and offers practical guidance for clinical professionals — especially CRAs and site teams — looking to upskill in an AI-enhanced environment.
Where AI Is Making A Real Impact
1. Patient Recruitment and Enrollment
AI can rapidly review millions of electronic health records (EHRs) using natural language processing (NLP) to identify eligible participants. Deep 6 AI reports ~96% matching accuracy; Inato instead touts 50%-90% prescreening accuracy. However, real-world gains depend on clean EHRs and thoughtful inclusion criteria. We’re arriving to the point of advancement where even unstructured data can help models deliver results. Done right, this tech empowers sites to reduce workloads, accelerate enrollment, and broaden access to underrepresented patients.
2. Protocol Design and Trial Planning
Machine learning (ML) algorithms analyze past trials and real-world evidence to optimize study protocols. Platforms like Saama and nference help teams identify ideal endpoints, flag impractical eligibility criteria, and even simulate trial outcomes. The result? Protocols that are more likely to succeed and require fewer costly amendments.
3. Site Selection and Feasibility
Site feasibility can be seen as guesswork and spreadsheets. AI models are triangulating EHRs, provider density, and regional demographics to predict site performance with growing precision. Tools like Power convert complex protocol criteria into plain language and compare them against patient inquiries to match trials with high-performing sites. This improves enrollment and reduces trial delays.
4. Trial Monitoring and Data Quality
AI supports risk-based monitoring by identifying anomalies in real time: delayed data entries, missing visits, or unexpected lab results. Some systems can even compare case report forms (CRFs) with source documentation to catch discrepancies early. This proactive approach improves data integrity and reduces surprises at database lock.
5. Patient Engagement and Retention
AI-powered decentralized trial solutions, like Medable, can help patients stay on track with study activities. These tools can send medication reminders, collect patient-reported outcomes, and deliver 24/7 support to patients experiencing technical difficulty. Reducing site burden and improving participant experience leads to better retention and compliance.
AI Limitations Are Real
AI tools hallucinate. They mirror human bias in their training data. They require thoughtful oversight and healthy skepticism. Research professionals and adjacent collaborators who blindly trust outputs may find themselves explaining preventable misalignment. The tech is promising but it’s not all wise, and we can’t forget it in our collective excitement. Sponsors and sites must also ensure AI usage complies with GCP, data privacy laws, and audit trail requirements. Automation can’t become a black box.
How Clinical Professionals Upskill In AI
AI won’t replace research professionals — but it will amplify those who upskill. That shift is already reshaping the workforce. Those who engage with AI tools now will help lead the transformation of clinical trials.
Here are five steps to begin:
- Build Foundational Knowledge: Start with understanding what AI is. YouTube accounts like IBM Technology do a great job of helping beginners put deep learning, machine learning, AI, and generative AI in their respective mental boxes. Building on that knowledge, Google DeepMind offers an amazing selection of AI ethics videos that are essential for understanding the gravity of AI misuse. Learn what tokens, context windows, and hallucination are in relation to AI. Read peer-reviewed trial-AI papers; start with the McKinsey brief, “How artificial intelligence can power clinical development.” I use a mixture of Data Camp and SkillSoft’s various AI courses to learn the inner workings of AI and prompt engineering. Start with free resources and work your way into more focused subscription-based learning.
- Explore Tools Firsthand: Use general-purpose AI tools like ChatGPT, Claude, or Perplexity to develop reflexive ability. Within the various aforementioned companies, there are different models, each skilled at specific tasks. As you explore these tools, you’ll reflexively know which models are best for the following: writing, thinking, building dashboards, or processing large data sets. Find out if your organization has an approved-for-use model and will allow direct use with internal documents.
- Examine Your Workflow: Identify repetitive tasks that consume time. Could AI help with prescreening, prioritizing site visits, or query resolution? I’ve cut hours from my week by streamlining protocol analysis, generating routine outreach/training, and simplifying monitoring visit calculations. For the adventurous, you can build tools — functional dashboards, purpose-built calculators — that reduce manual work and can be used by others.
- Join or Launch a Pilot: Volunteer for AI-related initiatives in your organization. No pilot available? Propose a small project, like using AI to analyze recruitment data or predict visit compliance. No organization in the industry should be ignoring AI use-case exploration.
- Stay Informed: Subscribe to industry newsletters, join LinkedIn groups, or follow companies working in this space. Ongoing exposure helps keep your knowledge relevant. I recommend strong voices like Allie K. Miller on LinkedIn, Alex Northstar (@NorthstarBrain) on X (formerly Twitter), and Ethan Mollick on LinkedIn for sharp yet deeply practical insights. AI progress moves far faster than major publications can keep up with and users like the ones mentioned here will keep you ahead of the curve.
The goal is momentum first, not mastery. Small actions compound quickly.
Conclusion
AI is already making processes faster, improving data quality, and reducing barriers for patient participation. For CRAs, site staff, and investigators, this moment represents a professional pivot point.
The ability to work with AI tools to solve fundamental problems, and not over-engineer solutions, will define the next generation of clinical research leadership. AI isn’t likely to take your job now…but it will change the shape and density of our workforce. Clinical leaders who upskill with AI become force-multipliers; everyone else risks obsolescence.
About The Author:
Bryce Nichols is a senior clinical research associate and consultant who merges regulatory compliance, I-O psychology, and data analysis to build resilient teams and improve trial outcomes. With experience across rare disease, cardiology, and vaccine studies, he specializes in transforming CRA-site dynamics into high-functioning partnerships. His work centers on optimizing workflows, strengthening team performance, and embedding audit readiness into daily operations. Bryce brings a systems-level lens to clinical research — training staff, reducing deviations, and turning compliance into a strategic asset. Passionate about leadership development, burnout prevention, and data-driven improvement, he helps sites and sponsors move from firefighting to foresight. In his spare time, he enjoys dancing salsa and bachata and solo travel