6 Ways AI Is Transforming The Life Sciences (Already)
By Rupen Mehta and Stephen Morehouse, PA Consulting
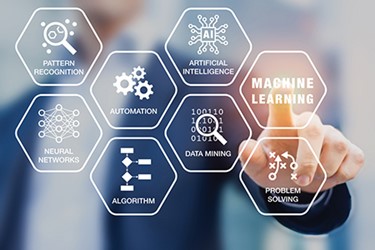
Most in the life sciences agree that artificial intelligence (AI) will reshape the sector from R&D through commercial. Although AI has only just left the starting gate and most activity is in exploratory phases, some benefits are starting to emerge. And there are pockets of early adopters trailblazing new approaches and seeking a competitive edge to accelerate products to market, improve patient outcomes and care, and drive cost efficiencies.
We have been studying AI early adopters and will explore how life sciences firms already apply AI in their organizations and how you can start to push your organization to explore a future not yet imagined.
Understanding The Key Components And Capabilities Of AI Technologies
Before we begin, it’s important to have a common language and understanding of what we mean when we say, “AI.” Is AI the same as machine learning? Where does robotic process automation fit? Oxford dictionary defines AI as “computer systems able to perform tasks normally requiring human intelligence such as visual perception, speech recognition, decision-making, and translation between languages.”
From our perspective, AI can be viewed along a spectrum. On one end there is rule-based automation. At some point, someone said the water block in a toilet is artificially intelligent; it knows exactly when to open the valve to let water in and exactly when to stop doing that. That’s an intelligent process. It is rule-based automation: if X, then Y. And there are people who say AI is just lots and lots of business rules, and if you have all the rules then you have a system that is intelligent. From our perspective that is where robotic automation, business process management systems, and rule-based management systems would sit. Think of this as things you get bored doing at work and would like to automate away.
On the other end, you have technologies such as natural language processing (NLP), machine/deep learning, chatbots, and virtual agents. NLP focuses on the interaction between computers and humans through language, from deriving meaning and sentiment from speech and text to generating language-based responses. Machine/deep learning uses historical facts to uncover patterns in that data, i.e., teaching an algorithm to recognize patterns and with the hypothesis that whatever happened in the past is a good predictor for the future. And AI mimics the cognitive functions of a human, building rules that are adaptable on the fly. In the end, AI is about making decisions. Yes, it’s making sense of a visual image, a sound, or speech, but it’s the decision-making that is important.
Exploring The “Art Of The Possible” For AI To Accelerate Products To Market
There are numerous areas where the life science industry uses AI effectively today. The following sections describe six of those areas.
1. Advancing Diagnostics
Histopathology image analysis and automated diagnosis were ripe for AI, given the technological progress in digitalization of complete histology slides, which permit all microscopic magnifications. AI and pattern recognition, combined with complex algorithms and automated immunohistochemical measurement systems, have advanced pathologists’ ability to oversee the analysis and concentrate on more-difficult cases.
2. Advancing Research Of New Products
Life sciences companies are exploring how AI can be leveraged to identify new indications for existing products or research new candidates. Examples include, but are not limited to:
- Using sophisticated learning algorithms to mine real-world structured and unstructured data to uncover insights can lead to the identification of new mechanisms of disease, potential new line extension, and design for preclinical experiments.
- Knowledge gaps of how candidates act on proteins to aid design of new drugs can be filled.
- Knowledge can be extracted in real time from commercial, scientific, and regulatory literature, allowing researchers to identify competitive white space, eliminate blind spots in research, and discover disease similarities.
3. Accelerating Drug Development
Across the industry, product development timelines range from seven to 10 years from discovery to launch, with sights set on reducing them to five to seven years. Advancements in AI and machine learning to reduce the time it takes to develop, manufacture, and launch new patient therapies support the goal of reducing overall product development timelines. Scientists are integrating research data, lab data, and clinical data, in combination with new information sources (e.g., social media and wearables) across the drug development spectrum, creating a holistic picture of the drug development candidate. Improving ways to acquire and mine data in real time allows scientists to use AI and machine learning to make improved decisions faster, which will accelerate the product development and scale-up process.
4. Driving Compliance In Clinical Trial Transparency
Compliance is often a burden on companies and requires an approach to mitigate costs while meeting regulation. The European Medicines Agency’s policies 0070 and 0043 are examples of regulations that have recently been introduced requiring companies to anonymize or redact patient information in clinical submissions. While generalist automation tools are available, many do not satisfy the accuracy needed to meet the policy’s requirements. New applications are emerging utilizing advanced algorithms based on customized NLP technologies incorporating scientific-specific taxonomies and text-mining models. Using these advanced models, it is possible to identify keywords, phrases, and data patterns (such as adverse event dates) that may require redaction or anonymization. These new applications provide the higher level of accuracy required to meet the policy requirements while also automating manual activities.
5. Improving Clinical Site Selection And Accelerating Patient Identification
Nearly 80 percent of clinical trials fail to meet their patient enrollment deadlines. Combining unanalyzed historical structured and unstructured clinical trial data into advanced AI models can improve and accelerate clinical site and patient selection decisions by highlighting high-probability targets. Continuing to use advanced AI models during active clinical programs enables real-time adjustment and course corrections. Engaging high-probability success targets at clinical trial onset in combination with a willingness to make real-time course corrections increases the likelihood of meeting patient enrollment timelines.
6. Optimizing Submission Dates Using Machine Learning/Predictive Analytics
Life sciences companies are responsible for up-to-date information on the safety of their products. Pharmaceutical labels (e.g., package insert, patient information leaflets) are an important way to communicate safety information. When an update to the reference label is necessary for safety reasons, there are multiple considerations (e.g., artwork printing, production run dates) for submitting the local product label to the corresponding health authority. Most companies’ SOP timelines that govern submission due dates are more stringent than what health authorities require, thus increasing workload and cost. Combining and connecting the appropriate data points with machine learning and predictive analytics can determine an optimal submission date.
How To Start Including AI And Automation
AI represents an opportunity for life sciences companies to radically transform business at all levels — organization structures, processes, and people. However, given how extensive the opportunities are, an overall vision is needed to shape what you want to accomplish. We recommend companies to think big, start small, and scale fast.
Think Big
Identify what you want to achieve by employing AI and automation technology throughout the business. Setting a strategic direction is pivotal. Define your business needs and assess the maturity of your organization against your ambition. This identifies the gap and roadmap to reach the desired maturity. Scenario planning provides tools and techniques that help to actively explore, influence, plan for, and manage the future. It can help to explore plausible futures informed by current trends and emerging signals of change. This approach is most effective when it is highly personalized to your organization, function, or team’s future, rather than a generic one.
Start Small
Try it out; experiment. See what works and what needs adjustment. Prioritize use cases on effort and value, challenging them by defining clear hypotheses. Successful use cases result in algorithms or models that can be quickly adopted as a new way of working.
Scale Fast
Build upon the successes of your experiments. Excite the people and organization around your successes. Catalyze the change your organization needs to truly become data- and AI-driven, embrace this transformation, and expand and grow the number of AI initiatives.
Roles will change, skillsets will need to be learned or acquired, new business opportunities will present themselves, and new relationships with vendors and business partners will be required. The growth of AI in life sciences presents an opportunity to take advantage of newly available data and technologies. Patients should be optimistic that new therapies and medicines can be discovered, developed, and made available faster than ever before.
About The Authors:
Rupen Mehta is a life sciences R&D expert at PA Consulting. He is focused on applying AI and automation technologies to transform R&D operations.
Stephen Morehouse is a life sciences expert at PA Consulting. He has a strong pharmaceutical development, clinical development, and regulatory background and is focused on improving business operations and processes through the advanced use of data and analytics.