3 Ways Biotechs Can Lower The Cost Of Clinical Trials
By Artem A. Trotsyuk, Ph.D., LongeVC
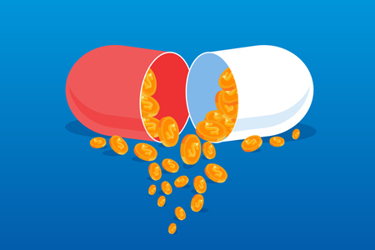
Biotech companies are well on their way to revolutionizing our medical care. In the next decade, we should see the availability of personalized cancer treatments, the use of nanotechnology, and even the re-engineering of our metabolomes become mainstream — pending, of course, their successful clinical trials. The clinical trial stage is challenging to many startups and scaleups, not least because of the costs.
Yet, several trends are emerging that indicate biotechs are using new technologies, and a more entrepreneurial approach, to reduce the expense of their clinical trials.
As backers of several clinical-stage biotech companies, as well as frequent reviewers of investment presentations for companies seeking to begin trials, we at LongeVC have identified the most innovative models to be: running a "pseudo trial" with AI; using synthetic data to streamline confidentiality approvals; and decentralizing the site of the trial through mobile providers or by holding virtual meetings. Together or separately, these approaches can help biotechs lower the cost of their clinical trials.
Rising in popularity is the concept of running a pseudo trial using AI. This is also called a pseudo-experiment and should not be confused with "pseudo-" or "quasi-" randomization. (Further reading on pseudo-trials can be found in the European Journal of Public Health.) Done correctly, we know these approach the rigor of a randomized clinical trial, yet cost far less. Involving AI reduces the costs even further. A well-known example is using AI to streamline preclinical drug discovery, making it more likely that candidates brought to clinical trials will be successful. However, the advent of open-source tools like Scite contribute to this cost-saving as well by making it simpler for biotech companies to check their claims against established bodies of research. The use of pseudo trials, with or without AI, is most economical when completed prior to a clinical trial, but biotechs can undertake them at any time to better prepare for the next phase of research.
The next cost-saving measure undertaken by many biotechs is the use of synthetic data. Patients, and patient data, are required for a successful clinical trial. Yet it represents a two-fold risk to the biotech's bottom line: the ability to retain patients and the ability to access valuable data without exposing patients to risk. Despite the industry's best efforts to retain patients for the entirety of a trial, upward of 30% of patients may drop out, which impedes the trial's ability to be completed. This is a major financial risk, but it pales next to the financial risk of improperly exposing patient data. Many biotechs address this by anonymizing data sets, but this process also can accidentally remove data that is relevant to the study. Another cost element is getting access to patient data sets in the first place, which can increase costs significantly.
Synthetic data streamlines the entire patient data process (thereby saving costs), sidesteps issues of confidentiality and privacy, and still protects the accuracy of results — while reducing the risk of trial dropout. Access to synthetic data should also grow in the coming years. In 2022, the open-source GANerAID library was published, and we will see more AI and data companies begin to offer synthetic data construction this year.
Lastly, biotech companies are cutting the cost of their clinical trials by integrating decentralization into their designs. This is usually done by making as many meetings/evaluations virtual as possible (a helpful after-effect of the virtual care offered during the COVID-19 pandemic), as well as decentralizing trial "interventions." This looks like, for example, having blood draws done through mobile providers or setting up an ecosystem of approved measurement companies that are available throughout the region of the clinical trial that patients can easily get to at their convenience. Another option can be for patients to mail in samples, and providers of this service also rapidly increased in availability in the years after the pandemic.
All of these adaptations reduce the time cost for the patient, which should reduce dropout risk (and therefore make the trial more likely to be completed), but they also save costs for the biotech company in terms of reduced physical locations and working hours to collect valuable data. If your trial still requires physical presence at a single location, I recommend you revise it immediately. While it may increase up-front costs in planning and infrastructure, it will save your trial in the long term.
In conclusion, biotech management teams planning to begin the clinical trial process in 2024 should consider adopting some or all of these models. By pre-planning with AI, utilizing synthetic data, and decentralizing your trial design, it is possible to cut costs while maintaining research excellence. In doing so, you are setting your biotech company up for success in what may be its most critical stage.
About The Author:
Artem A. Trotsyuk, Ph.D., is a partner at LongeVC, an early-stage investment firm specializing in biotech and longevity. Alongside this role, he is also serving as Stanford University’s AI Ethics fellow and advises biotechnology companies and startups.