7 Ways To Modernize Statistical Analytics To Drive Innovations For Patients
By Daniel Woodie, Merck; and Min Lee, Amgen
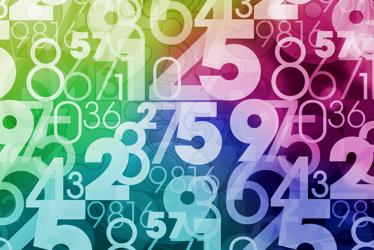
The increasing number of data sources collected as part of drug development and commercialization has vastly increased the amount and types of data biopharmaceutical companies collect. This has increased the need and urgency for technology that can process large amounts of structured and unstructured data to support regulatory filings.
Many companies have existing standard operating procedures for common off-the-shelf commercial offerings. However, the increasing complexity of data analysis that is now required often means that companies need to modernize their statistical offerings through nontraditional (e.g., open source) software.
As such, TransCelerate BioPharma recently produced a Modernization of Statistical Analytics (MSA) Framework that can be used as a methodology that demonstrates to health authorities that these nontraditional software capabilities are reliable. Our goal is to build confidence in cutting-edge analytical programs, which will ultimately help bring new medical breakthrough products to patients more quickly.
This framework has been developed to provide practical guidance on how to demonstrate that an analytics solution produces credible results and is suitable for use in the regulatory process. TransCelerate has garnered a few key takeaways for sponsors to consider when looking to introduce nontraditional statistical analytical tools to their clinical trial processes.
Below are seven tips that can be used to help implement a modernized statistical analysis environment:
1. Focus On Accuracy, Reproducibility, And Traceability
Prioritizing these three core principles of the MSA framework helps ensure that the system is able to support regulatory decision-making and generates reliable results. For our purposes, accuracy is defined as the measure of correctness of software libraries that are used to generate results from an MSA environment, not the programs that developers write using those software libraries. Reproducibility then is demonstrated by showing a statistical analysis output that can be recreated from the original data set along with the associated environment, including all artifacts and dependencies. Traceability refers to the ability to trace inputs to outputs, with the main goal being to provide evidence that connects data, code, and environment to the final output that is produced.
It’s important to note that the three principles do not work in isolation — rather, they must be fully integrated together to achieve success. Ensuring this full, end-to-end control will provide health authorities with confidence that the MSA system is reliable.
2. Build On Legacy Systems
While the concepts in the MSA framework can be basic in theory, they may prove challenging to implement as current environments in biopharmaceutical companies often use existing legacy technology, which can perpetuate a mindset to reinforce the status quo and thus can act as a check on the evolution of analytical programming languages.
For companies looking to modernize their legacy systems, creating a set of best practices and exploring potential use cases before implementation can be invaluable. Preparing materials such as these can also help develop support among senior leadership, which will allow for the modernized systems to be introduced easier and seamlessly.
3. Create A Hand-Off Between Business And IT
IT traditionally builds and maintains computing environments based upon SOPs and other defined processes that ensure reliability, which might be different than the tools or automated processes followed on the business side. As such, there are risk and change management aspects to consider since these two sides of the coin may not be following the same process for the statistical analysis environment.
Collaboration across the organization can expedite timelines and improve the implementation of new technologies that have actual impact on business outcomes. A crucial factor to ensure a smooth transition and hand-off between business and IT is for the senior leadership of both units to create a partnership early on and define clear roles and responsibilities for the cross-functional team members.
4. Create And Document Any Efforts As Part Of The MSA
Creating a culture that automatically collects, collates, and shares documentation on how analytical processes are implemented is crucial. This will help demonstrate to stakeholders in this environment, including health authorities, that the lineage of dependencies relied upon by statistical analysis systems are valid. Documentation guidelines, best practices, and processes need to be clearly defined and required as part of the MSA analysis from the beginning of the programming life cycle. Programmers should be documenting as they develop, test, and report.
5. Create Clear Roles Within An MSA Environment
There are various roles involved in creating an MSA and moving it forward. From statisticians to engineers and clinical quality experts, modernization of statistical analysis requires many roles to work together to advance and improve the statistical computing environment.
Open communication among business functions is imperative to identifying and assigning these roles, and delivering the MSA framework depends heavily on collaboration among working teams. As mentioned above, one of the critical key success factors is ensuring that roles and responsibilities are clearly defined. Communication and collaboration ensure effective coordination between stakeholders, and, ultimately, help deliver an effective MSA environment.
6. Develop A Testing-Driven Programming Strategy
The level of testing required for the MSA environment is critical to ensure its success. There is a balance between too much testing – that leads to an inflexible environment and can hinder a programmer’s ability to leverage the packages they need for deliverables – to too little testing that may compromise confidence in the software’s reliability. A thorough examination of where risk is high versus low and experimentation will be key to strike the right balance. A key component of obtaining the right balance is to have cross-functional alignment and input from multiple teams such as quality, compliance, biostatisticians, programmers, IT, etc.
7. Focus On Culture
None of this is possible without embracing a mindset for innovation. Support for modernization must come from all levels of the organization, including senior leadership. This is crucial to conduct the necessary changes in order to remain on the cutting edge of statistical analysis.
Collectively, these seven tips — outlined further in our framework document — can help companies implement these technological innovations that ultimately improve the ability to bring lifesaving medicines to patients more quickly.
While each organization will have to determine its own desire for change and willingness to balance any risks, embracing a culture shift toward modernizing statistical analysis will be crucial to the ability to bring medicines to patients more quickly. As data sources will continue to expand in the coming years and decades, TransCelerate’s MSA framework will allow stakeholders to stay ahead of that trend.
About The Authors:
Daniel Woodie currently is a senior developer at Merck in the engineering group supporting the research labs. He is the IT technical lead for building the computational platform for the biostatistics group to use open-source tools for regulatory use. Daniel holds master’s degrees in both statistics (M.S.) and psychology (M.A.) from The University of Texas at Austin and a bachelor’s degree in psychology & biology from Texas A&M University at College Station. He serves as team co-lead for TransCelerate’s Modernization of Statistical Analytics Team.
Min Lee is the head of technology within Amgen’s Global Statistical Programming department, responsible for the development of strategy, tools, and processes to support the use of technology to improve the conduct of clinical trial analyses. Min has previously served as an information technology professional for Amgen’s Research and Development Informatics department, where he managed Amgen’s SAS grid platform and established Amgen’s first R platform for Clinical Development. He serves as team co-lead for TransCelerate’s Modernization of Statistical Analytics Team.