What Is Your AI Road Map To Revolutionize Drug Development?
By Patrick Nadolny, associate VP of clinical data management and programming, Allergan (an AbbVie company)
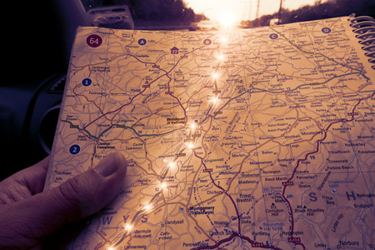
When it comes to artificial intelligence (AI), many organizations do not know where to start or waste their energy going in many directions without a clear vision. They may not even fully understand what AI is and where to leverage it. As a result, too many proofs of concepts fail to show its true value, leaving leaders dreaming of a hypothetic better drug development future. Fortunately, there is a path forward for those able to understand its potential and articulate a clear strategy.
This article will share insights on how to pragmatically initiate an AI journey using the evolution of clinical data management as an example.
Some Perspectives On AI
Some thought leaders foresee the evolution of intelligent technologies and the increase in real-time connectivity to almost everything (i.e., Internet of Things, or IoT) as the fuel leading to a fourth “industrial” revolution in the coming years. If this were to happen, one of the key levers would very likely be AI, as it would not be feasible to cope with the accompanying deluge of data otherwise. There would not be enough data scientists to generate real-time actionable insights or knowledge from it.
But like previous industrial revolutions, we need pragmatic, reliable, and large-scale solutions leading to sustainable and meaningful change. The maturity of IoT and intelligent solutions is no longer to be questioned. They have successfully invaded our homes, mobile devices, and cars. But, is drug development ready to tap into those game-changing technologies? If yes, how?
What Do We Mean By AI?
Simply explained, AI is a toolbox. Each of its tools has a specific purpose and can be used separately or together to build a solution. These tools have three major functions: automation, interaction, and reasoning. Process automation is not disruptive, as its main intent is to optimize existing practices. Nonetheless, it can still bring meaningful return on investment (ROI) by eliminating labor-intensive and repetitive tasks such as automating the serious adverse event (SAE) reconciliation for companies still having siloed safety and study databases. On the other hand, leveraging reasoning solutions can transform entire business models. One example is the use of intelligent solutions to discover new therapies to speed up drug development, which would ultimately transform the current research model.
It is important to understand that advanced AI tools need to be applied on raw material (i.e., our data), which can come in many forms and from many sources: data repositories, IoT (e.g., sensors and wearables), images, voice recordings, and unstructured data (e.g., documents such as protocols and clinical study reports).
How To Get Started With AI
From a pure business standpoint, AI is no different than any other technology. When implemented, its return needs to meaningfully exceed the original investment. It can be applied as a tactic to optimize operations and/or as a strategy to change an operating model. The key is for functional leaders to understand its potential and its applicability to define an outcome-based road map aligned with their own organization profile and vision. They should consider all potentials, from simple automations to transformative and intelligent solutions.
Mature organizations with efficient and cost-effective processes may have very little ROI from simple automations. In contrast, large organizations with manual and labor-intensive tasks may realize fast ROI with minimum investments. Automation may also be leveraged to increase quality by reducing manual hand-offs. Transformative intelligent solutions are more complex to implement than process automation. They require deep process knowledge and significant historical data that younger or smaller organizations may not yet have. It is important to understand what the requirements are before embarking on such a journey. It may be necessary to start aggregating and curating data before initiating the development of an intelligent solution. As a result, an overall pragmatic road map needs to consider all prerequisites as a foundation, including data and business process expertise. The road map must also consider the downstream impact on the operating models, partnerships, and talent acquisition and retention strategies.
Example Of A Road Map To Transform A Data Management Function
The Society for Clinical Data Management (SCDM) has released reflection papers1,2 on the evolution of the data management discipline as a result of the increased complexity of clinical trials and the opportunities offered by new technologies. The second paper2 focused on technology provided the following example of a potential road map transforming the data review process through AI technologies.
Stage 1 may consist of automating manual processes using robotic process automation (RPA) to generate short-term savings and free up valuable data management experts with deep process knowledge. This process optimization stage would also result in improving quality by reducing human errors.
During stage 2, freed-up process experts would identify data review scenarios. They would also identify and curate the data required to train the ML-models. The model would likely need to leverage natural language processing (NLP) to analyze text such as adverse events and comments. Experts would then ultimately take and document their corrective actions resulting from the output of the ML-solution. At this stage, the automated process may be performed in addition to existing processes to confirm its robustness and viability and to demonstrate credibility to all stake holders, including auditors and regulators. So, stage 2 would serve as a model maturity-building phase to determine the model’s accuracy in predicting all signals.
During stage 3, the same experts would continue to refine the signal detection models and train the system to suggest corrective actions by learning from the associations between signals and actions documented during stage 2. The solution would now need to use natural language generation (NLG) to suggest understandable actions based on the context of the data anomalies. Assuming stage 2 demonstrated the expected solution reliability, the organization could start realizing operational savings by reducing overlap between the legacy and emerging models.
During stage 4, the ML-solution will use intelligent process automation (IPA) to perform actions in systems such as electronic data capture (EDC) to raise a query or a clinical trial management system (CTMS) to enter a new protocol deviation. The expert will supervise the intelligent solution commensurate with the accuracy determined at stages 2 and 3. The expert would also retrain the model as necessary.
In summary, the organization will gradually realize operational efficiency from stage 1 and build confidence in the future emerging model by taking a wise stage gate approach. At the conclusion of stage 4, the data review model could be totally transformed. The organization could leverage a virtual workforce working 24/7. In future, scaling up the number of clinical trials would not require a proportionate increase in resources but rather an incremental investment in computing power.
Where Do We Go From There?
The road map described above is not a fallacy. Some leading organizations have already entered stage 2 and determined the viability of AI-based solutions. Following such a path is not easy and requires assessing the downstream impact on processes and people. For example, data management experts would need enough knowledge of ML-model development prior to initiating stage 2. They would need to understand what AI tools to use for what purposes. They would also need to be aware of the ethical risks associated with biased training data sets, especially when using AI-based tools to support safety reviews.
Additionally, organizations would need to come up with new software validation paradigms that would demonstrate regulatory compliance to auditors and regulators. The regulators are also anticipating the rise of intelligent applications and taking action. For example, the FDA proposed a regulatory framework for modifications to AI/ML-based software used as a medical device.3 The EMA inspections office, together with the good clinical practice inspectors working group, has organized a virtual meeting on “Artificial intelligence in clinical trials — ensuring it is fit for purpose” in September 2020.
Conclusion
Intelligent applications will change the way we do clinical research way beyond data management. Similar strategies can apply to other clinical research activities, including clinical and safety reviews. Intelligent solutions could be created to automatically extract data from clinical study reports of potential acquisitions and provide safety and efficacy summaries for due diligence. AI solutions based on deep learning have already proven to be successful at assessing lesions from medical images.
There is no doubt that the prospects offered by AI are vast and considerable. But a vision that cannot be realized is just an illusion. So, to realize this potential, organizations need to be realistic and pragmatic. They need to define a focused strategy that fits them and is outcome-based. The strategy needs to consider all factors, including people, process, technology, and partnership. The key to success is to set a clear vision and define an achievable road map delivering value throughout the journey.
References:
- SCDM, June 2019, The Evolution of Clinical Data Management to Clinical Data Science — A Reflection Paper on the impact of the Clinical Research industry trends on Clinical Data Management.
- SCDM, March 2020, The Evolution of Clinical Data Management to Clinical Data Science (Part 2: The technology enablers) — A Reflection Paper on the impact of the Clinical Research industry trends on Clinical Data Management.
- FDA, 2019, Discussion and Request for Feedback, “Proposed Regulatory Framework for Modifications to Artificial/Machine Learning [AI/ML]-Based Software as Medical Device (SaMD)”
About The Author:
Patrick Nadolny is chair of the Society for Clinical Data Management (SCDM) Innovation Committee and associate VP of clinical data management and programming for Allergan, an AbbVie Company.