What's The Risk Of Letting Researchers Use LLMs?
A conversation with Jakob Alme, head of digital health, Sage Therapeutics
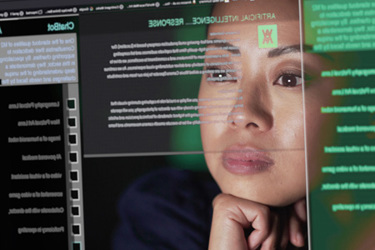
Large language models (LLMs) and generative AI have ushered in a new era for various sectors, and clinical research is no exception. The accessibility of public AI infrastructure has empowered life sciences professionals to explore novel approaches to drug discovery, literature reviews, protocol design, and more. However, this democratization also introduces critical questions about data security, intellectual property, and the evolving sophistication of AI applications.
In this interview with Sage Therapeutics' Head of Digital Health Jake Alme, we explore the current landscape of AI use in clinical research, examining the shift from basic information retrieval to more complex directive applications. Alme also discusses the inherent risks associated with using public LLMs in a highly regulated industry and discusses strategies for mitigating those risks.
Clinical Leader: How are public and private AI models being used in the clinical research space?
Jakob Alme: LLMs and generative AI are relatively new to the public domain, and what they first did is democratize the ability for the layperson to access AI, which meant that anyone, including life sciences professionals, could start using public domain infrastructure to answer queries. Anything that doesn't inherently disclose confidential intellectual property is relatively safe for the public domain. This would include, for example, general questions on industry status, competitive analysis, or literature reviews. We can continue to use some models for documentation purposes or speeding up the logistics of anything that would be an industry standard practice, protocol design or something like that. Anything that's external-facing or unrelated to organizational data is probably okay for the public domain, and you'll also benefit from that large language model's ability to interpret and be trained on data sets that you do not have available to you.
Do you think people first began using it as a glorified internet search, and now we're transitioning into more directive exercises?
That's safe to say. When OpenAI launched ChatGPT, it seems like it did become the default interface for asking quirky questions. It was very much, “I'm going to ask a question, give it some parameters, and let it figure out what it wants to spit back out.” You could give it a task and add a few steps that you would normally have to do yourself, and now the manual legwork required to complete the search is offloaded already.
But now we've entered into the territory of it giving you the answer you're looking for and giving you results of a build, such as “Please build me a slide deck based on this topic.” It's gone from basic queries to creating something from scratch based on information in the public domain.
This is probably the next fundamental change that the life sciences industry will have to adjust to and adopt. It’s probably very similar to when everything went from paper records to digital — you could either get on board or be left behind.
What are the risks of pharma employees using a public LLM?
The short answer is intellectual property disclosure. The long answer is what the model is going to do with that information. So, not only could someone look up the information that you've just entered either intentionally or unintentionally into the public domain, but now the models are going to be trained on that. If it's an open source LLM, it will start training on any new information that comes in, and your data that you've just entered is now a part of that. Someone could query directly for information you don't want to share, and they could also unintentionally end up having it included in their results.. And those are both dangerous, because industry trade secrets are always important to keep for the sake of innovation.
How should companies be mitigating those risks?
It starts with a fundamental level-setting on what artificial intelligence is and how it works at a very basic level.. If you make sure that everyone is working from the same place about how artificial intelligence is impacting your organization, then you can move forward in creating trainings and procedures while appreciating the nuances of why one technique works better for a task than another. Ideally, you can get past the predetermined guardrails of how people are interacting and now talk about, well, why are we using it in the first place and what's are going to be the best ways?
I've also seen some companies approach it holistically with an AI working group or some dedicated team of cross-functional employees that have both domain expertise and are also representative of their function. When you're looking at use cases across the company, you have the voice of each of those represented. So, each vertical isn't doing something entirely different. Now it's all aligned ideally from one central group that's keeping oversight, not for the sake of saying yes or no, or “this is bad” or “this is good,” but rather ensuring people are doing it safely the way we expect them to.
What if something is entered into the public domain mistakenly? Can you retract it?
Not that I'm aware of. Maybe some models are different, but with standard ChatGPT, if something gets entered, it's archived in their data warehouse forever, roughly speaking. There may be different policies emerging with the industry-specific models. We'll probably see more of that evolving, but those are more than likely to be private anyway. So, if it's in the public domain, it's the same as whispering a trade secret in a crowded bar after work and someone hears you. There's no way to take that back.
Which begs the question, are companies, pharma or otherwise, equipped with the tech infrastructure and staff to oversee and train employees on the use of AI?
Some are and some aren't. Those who were exploring artificial intelligence before large language models came out probably have a leg up on those that weren't. But the main difference is getting employee behavior to align with the safety and guardrails of using artificial intelligence in their day-to-day work when they are not a specialty user who has been previously building machine learning models or even pattern analysis. Those things are historically what artificial intelligence has been used for. And now we've entered a new territory where it's open to the layperson.
The smaller companies or the those just starting to look into it, they're going to have a little bit of a delay. When it comes to the model for success between governance, use cases, and who owns the product, they're going to rely on what comes out in the landscape before they make any decisions. Some of them will get it really right, and some of them will get it really wrong. There's going to be a spectrum of companies where some are highly rigid and regulate their internal use very strictly, and then there are those that are more lax and want to see what the employees can come up with and why it might actually be a value add to their organization as opposed to a risk.
Do you have any tips for companies that are at the starting line and trying to figure out how they should be using LLMs?
The best thing to do first is to identify what use cases you have for artificial intelligence. You can go all the way from pre-discovery compound work to enterprise G&A functions. Figure out the highest priority and what value artificial intelligence could add. And then, step two, you have to do the hard part of figuring out how to integrate the technical stack within your own layer of software. That's a big challenge for larger organizations that have systems across the entire company. They're probably custom instances of public software that they've tweaked for their own parameters of use, such as clinical trial management systems. You're going to have to figure out how your information technology or your business team implements and maintains the technological infrastructure. We want to figure out how that specific instance of artificial intelligence is going to play with all of the other functions that your software team is already maintaining.
And then step three is governance and policy, making sure that your employees understand the different AI techniques like a large language model and generative AI versus regression analyses and machine learning. And they have to know how to safely interact with it.
The last step is actually the most fun, which is where your organization gets to use it and be turned loose safely to figure out what works and what doesn’t. For example, a translational scientist looking to find a target interaction on a molecule instead of combing through the proprietary database of thousands of compounds. They’ll ask generative AI to query the database and tell them which binding site would work with a given molecule, and it will spit back papers that match that question.
Now, users are beginning to tell product builders what they would like to see out of AI. And it's certainly going to be a race to implement all the features that every organization wants, because you want to serve as many customers as possible. Then we have discrete use cases for AI, and just like every other technological advancement that the life sciences industry has seen in clinical research, there'll probably end up being a few big providers, some bespoke smaller ones, and then the very niche ones that are focused on a specific use case.
Is there anything that you can share about Sage’s use of AL and LLMs?
I can't disclose anything specific about the way we're working, but generally, we are taking a moderated approach to introducing some of these AI capabilities, and it's starting at the general administrative level. We didn't go right into “I want to do preclinical discovery with generative AI” or “I want to do claims analysis with an LLM.” We started with the very basics that our tech stack is already offering, like Microsoft’s Copilot. Zoom’s assistant, and so on., etc. Those are easier because you're already using the system, so while it's not necessarily as easy as turning a switch on, it feels like that to the user.
The next step is addressing the organization's needs to do an astronomically large task or if we're having trouble with resourcing. We need to augment or supplement with something to cut the volume of work down. And that's where you have to start assessing who's building what, and what the risks are of implementing one versus another. And that's more of a technological landscape question than a governance one. We have a working group and a couple of domain experts in our digital enterprise capabilities function conducting learning sessions. The next step will be addressing those niche workflows and things that may not be high value in the short term but long-term are just going to be the cost of doing business.
Bonus question: What is your favorite LLM, and what do you use it for?
In my personal life, I enjoy playing around with some of the image generation tools. Those are getting better. It used to be that you couldn't even get a picture with someone with five fingers on it… Now they're getting a little bit more accurate. I don't think we're quite there yet, but it's fun to have visuals in your head come out without you ever having to have any artistic ability.
Some other models I'm really interested in, like Claude from Anthropic, are more on the healthcare side. I don't know that it's quite reached implementation in biotech or biopharma, but clinical documentation and note-taking for the chart will be highly transformative. I'm interested to see where that goes, not only for the ability to take some burden off the site staff, but also now that we have that information included when someone wants to do longitudinal retrospective work with claims or chart abstractions, that information is no longer just sitting unstructured in the EMR notes. Now it's a little bit easier to query, and that historically has been a big challenge for people and a higher price for anyone that's interested in conducting that sort of research.
About The Expert:
Jakob Alme joined Sage Therapeutics in 2021. As the head of digital health, he is focused on rethinking care and how it is delivered to help patients and families impacted by brain health disorders. In this role, he leads the function’s efforts in implementing digital health strategies across the organization, ranging from preclinical and translational through development, commercialization, and on-market planning. Prior to his time at Sage, Alme led the digital health asset design for immunology at AbbVie and also held roles in clinical operations, compliance, and data sciences. He holds a BS in health and human performance from the University of Montana.