A New AI Collaboration Eliminates Tedious Research Reviews

By Ed Miseta, Chief Editor, Clinical Leader
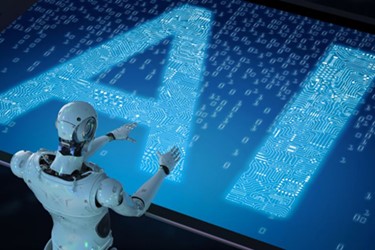
The Jackson Laboratory (JAX) is a non-profit biomedical research institution that has been in operation for over 90 years. The institution is headquartered in Bar Harbor, Maine with facilities in Sacramento, California; Ellsworth, Maine; Farmington, Connecticut; and Shanghai, China. The goal of The Jackson Laboratory is to discover precise genomic solutions for disease and empower the global biomedical community in the shared quest to improve human health.
In recent years, biomedical research has faced a daunting challenge. A bottleneck, caused by knowledge creation, blocks scientists and physicians from staying up to date on specific genomic advances and quickly matching patients with treatment options and clinical trials.
Part of CKB – now available externally – is utilized by physicians to gain insights on clinical trials and for patient recruitment. Physicians needed to know how to interpret their patient’s tumor mutations to be able to recommend a treatment option. They needed to know what each mutation would do, why it caused a cell to grow the way it did, and how to stop it with various targeted therapies. The situation gets even more complicated when trying to identify the level of evidence in scientific journals.
“Imagine you're a physician,” says Mockus. “You read a patient report and discover there are five or six different DNA mutations in this patient's tumor. You try to figure out what those mutations do and how it's causing the tumor to grow. And there are a dozen or so therapies on the report as well. If you want to get that patient into a clinical trial, how do you know which is the right one?”
Evidence-Based Medicine
That is where evidence-based medicine enters the picture. According to Mockus, that physician would have to go through the process of examining the available evidence to determine what targeted therapies are available to target that mutation in their patient’s tumor type.
Published literature in scientific journals and professional guidelines contains a lot of data. That data is curated into CKB, a searchable database where JAX subject matter experts store, sort and interpret complex genomic information. Previously, it was difficult, perhaps impossible, to keep up with the sheer volume of papers published every day.
“If you look across all biomedical literature, there are almost 4,000 papers published every day,” says Mockus. “Physicians would have to determine which ones are cancer related, determine which ones mention the mutation they are looking for, and figure out if they are connected to a targeted therapy. The real problem is data volume. It’s like taking a physician who is already busy and asking them to look for the proverbial needle in a haystack.”
According to Mockus, the first step in managing the data is identifying relevant pieces of literature. The next step involves teasing apart that information. That involves knowing the size of the patient cohort in the study, and the technique, technology, and controls used in the study. “For example, we would want to know if there was one patient of 1,500,” she says. “We also know not all assays that use next generation sequencing are the same.”
To get a handle on this Big Data problem and identify relevant literature to curate into the CKB, The Jackson Laboratory formed a collaboration with Microsoft. This collaboration utilizes AI technology from Microsoft’s Project Hanover. Hanover, from Microsoft’s healthcare division, is still in the development phase. It uses natural language processing to machine read the published literature and make a prediction ranking for relevancy.
The Jackson Laboratory compared Hanover to human curators. A drug was selected and a human curator, using a manual process, was able to identify and triage 823 relevant papers and two relevant patient responses. The tedious process took almost eight hours.
Hanover, using its machine reading and ranking capability, was able to whittle down 43 relevant papers and 22 relevant patient responses in a matter of seconds.
The Only Limit Is Imagination
The Jackson Laboratory is leveraging the Hanover technology as part of the Clinical Knowledgebase curation process. Hanover takes new papers, including information on cancer mutations and drugs and predicts their relevancy using machine-reading. This technology accelerates the rate at which JAX subject matter experts sort, interpret, and curate the most important data into CKB. According to Mockus, everybody benefits from Hanover because CKB subject matter experts can perform their jobs faster and more efficiently by focusing on what really matters – interpretation of the data.
Once a user gets caught up on all the stored information, they will be able to opt to only see new information that is added. “Microsoft works closely with us to add features,” says Mockus. “One of the features gave us the option of only viewing clinically relevant papers or only preclinical papers. We also have the ability to toggle certain parameters.”
“This is not a black box AI tool,” adds Mockus. “We know exactly how it works. We can reproduce it and continue to improve it. There are a lot of so-called AI tools that are just using AI as a buzzword. The products are not doing any type of AI machine learning. That means it is difficult to replicate the process or understand how they work. What we have built is a solid foundation and framework that uses big data and disruptive AI technologies. Right now, I believe the possibilities are endless in terms of what we can apply this technology to. I think it will enable rare disease matching, manuscript identification, and mutation matching across different phenotypes in diseases. Moving forward, we will be limited only by our imagination.