Adaptive Seamless Design For Phase 2/3 Studies: Basic Concepts & Considerations
By Elaine Eisenbeisz, Omega Statistics
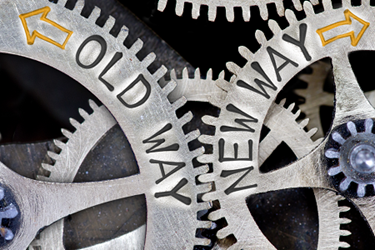
An adaptive design is a design that allows for modifications to the processes and statistical procedures of a clinical trial, usually at set intervals established in the trial protocol. Adaptive designs are useful for increasing efficiency and lowering costs associated with clinical research. Studies incorporating adaptive design techniques can be useful in redirecting subject allocation to concentrate on the most promising treatments or in stopping a trial early for futility. The temporal and monetary savings resulting from adaptive designs make them of great value in drug and medical device development.
However, many regulatory professionals and statisticians find the FDA guidance on adaptive designs, and the nuts-and-bolts of implementation of adaptive designs, difficult to interpret. Adaptive designs are based on Bayesian statistics rather than the traditionally used frequentist statistics. Frequentist designs require the assumption that we do not know anything until we randomize our subjects into treatment groups and run a full study before assessing outcomes. Bayesian statistics allow the researcher to use a priori knowledge, information we can assume we know already, as well as the information obtained during the course of a trial, in making decisions. Hence, we can use Bayesian methods to adapt the conduct of our trials.
I certainly cannot address Phase 2/3 adaptive designs in total in one article. But it is my hope that the information I share will give those interested in adopting the adaptive design a bit of guidance, and comfort, with the concepts of the process. And because all trials are different, just as with the traditional frequentist designs, there is not a one-size-fits-all approach to adaptive design.
Plan For An Adaptive Design When Writing The Protocol
Not all changes in the design after study initiation are considered adaptive design. The FDA defines some types of changes as reactive revisions. A reactive revision is a change that was made due to unplanned findings during an interim analysis or revisions based on information that is received from a source external the study. Thus, it is imperative to consider the possible outcomes for each interim analysis when planning the protocol and to write out, as thoroughly as we can, the next steps that will be taken for each of the outcomes.
If the revisions to a study design are not incorporated into the protocol at the outset, reactive revision could invalidate a study. There are exceptions to this standard. According to FDA guidance, “In cases of serious safety concerns, and particularly in large studies, revising the study design may be critical to allowing the study to continue.”1(pp17-18)
Seamless Design Vs. Adaptive Seamless Design
A seamless design combines two separate trials (individual Phase 2 and Phase 3 trials) into one trial. This type of design is called operationally seamless.
An adaptive seamless design makes use of information (data) from patients enrolled before and after adaptation (pulls together data collected in both the Phase 2 and Phase 3 trials) in the final analysis. Thus, an adaptive seamless design is inferentially seamless.
The primary purpose of using the adaptive seamless design is to combine both the dose selection and confirmation phases into one trial, so information from the learning stage (Phase 2) can be combined with the confirmatory analyses of Phase 3.
Adaptive Seamless Design Can Reduce Time And Costs
Since we can combine Phase 2 and Phase 3 data in an adaptive seamless design, we can use data from the Phase 2 trial to inform Phase 3. This is the Bayesian way of thinking. Combining the information allows us to:
- More efficiently use patient data to infer strong conclusions
- Reduce the number of patients who must be enrolled at Phase 3, thus saving time and money
- Improve selection of target doses and participants in Phase 3
- Investigate possible covariates between short-term endpoints derived from Phase 2 and long-term clinical outcomes in Phase 3
- Continue to follow patients on terminated treatment groups from Phase 2 throughout Phase 3, providing more information on time effects of treatment as well as safety
- Change or cut treatments during the study, resulting in patients having a greater chance of receiving safe and efficacious treatment.
Two Important Statistical Considerations
The analysis of adaptive seamless designs must account for biases introduced by the process, most importantly:
- Multiplicity due to repeated testing, which can increase Type I errors (seeing significance just by chance, not real significance)
- Bias of treatment estimates due to combining data from independent stages in the study.
There are many ways to handle multiplicity. Two common methods are a Bonferroni correction and the closed testing approach.
Bonferroni Correction
A Bonferroni correction is easy to calculate, but it is very conservative and may increase the chance of Type II errors (missing out on seeing significance of treatments that are truly effective).
A simple Bonferroni adjustment is performed by dividing the desired level of significance (α, usually it is 0.05) by the number of analyses performed. So, if there are a total of two interim analyses and two endpoint analyses in a study, the new level of significance would be .05/4 = .0125.
Closed Testing Approach
Another approach to adjust for multiplicity is the closed testing approach (also called the Bonferroni-Holm method), which gives a study greater power than a Bonferroni correction, especially when there are numerous interim and endpoint analyses. In essence, the closed test procedure uses the following steps:
- Consider the number of tests that will be performed (testing null hypotheses H1, H2, …Hn)
- Look at the significance (p-value) obtained for each tested hypothesis and rank them from smallest to largest
- Compare the p-values, rank by rank, using the following formula:
HB = Target α / (total number of tests – rank +1)
Test the formula up the ranked p-values until you find that all of the null hypotheses are rejected, or stop testing when you reach the first non-rejected null hypothesis. If you reach a null hypothesis that cannot be rejected, then the remaining null hypotheses up the ranks are also not rejected.
An easy to read reference on the closed testing approach is here:
http://www.statisticshowto.com/holm-bonferroni-method/
Consideration must be given to the positive bias in the treatment effect that is introduced to an adaptive seamless design by combining data from both phases.
- The bias will increase with increasing numbers of treatment groups present in Phase 2.
- Also, the bias will increase if Phase 2 includes a larger part of the final combined data (i.e., as the ratio of [# of subjects in Phase 2 / # of subjects in Phase 3] increases in value).
Computer simulations are performed to quantify the bias. If you would like to see some math, this article by Kimani, Todd, and Stallard (2013) is the easiest read I’ve found, although it really isn’t so easy:
https://www.ncbi.nlm.nih.gov/pmc/articles/PMC3813981/
I recommend trying to use a computer simulation. A computer package that can be helpful is the simstudy package in R.
Adaptive seamless designs are not easy to implement. They require intense forethought and the statistics can get quite involved. Additionally, FDA personnel and many IRB boards remain uncomfortable with almost all but the most rudimentary designs. However, the savvy researcher should at least be aware of the uses and possibilities of adaptive designs. I hope to discuss different uses of such designs in future articles.
What statistical concepts or applications would you like to know more about? Let me know your ideas for future articles by posting a comment below or by sending an email to elaine@OmegaStatistics.com.
References:
- FDA Draft Guidance for Industry: Adaptive Design Clinical Trials for Drugs and Biologics: https://www.fda.gov/downloads/Drugs/.../Guidances/ucm201790.pdf
- FDA Guidance for Industry: Adaptive Designs for Medical Device Clinical Studies: https://www.fda.gov/downloads/MedicalDevices/DeviceRegulationandGuidance/GuidanceDocuments/ucm446729.pdf
- FDA Guidance for Clinical Trial Sponsors: Establishment and Operation of Clinical Trial Data Monitoring Committees: https://www.fda.gov/downloads/RegulatoryInformation/Guidances/ucm127073.pdf
- Kimani, P. K., Todd, S., & Stallard, N. (2013). Conditionally unbiased estimation in phase II/III clinical trials with early stopping for futility. Statistics in Medicine, 32(17), 2893–2901. http://doi.org/10.1002/sim.5757
About The Author:
Elaine Eisenbeisz is a private practice statistician and owner of Omega Statistics, a statistical consulting firm based in southern California.
Eisenbeisz earned her B.S. in statistics at UC Riverside, received her master’s certification in applied statistics from Texas A&M, and is currently finishing her graduate studies at Rochester Institute of Technology. She is a member in good standing with the American Statistical Association and a member of the Mensa High IQ Society. Omega Statistics holds an A+ rating with the Better Business Bureau.
Eisenbeisz works as a contract statistician providing study design and data analysis for private researchers and biotech startups as well as for larger companies such as Allergan and Rio Tinto Minerals. Throughout her tenure as a private practice statistician, she has published work with researchers and colleagues in peer-reviewed journals. You can reach her at (877) 461-7226 or elaine@OmegaStatistics.com.