Can Data Help Improve Diversity In Clinical Trials?

By Dan Schell, Chief Editor, Clinical Leader
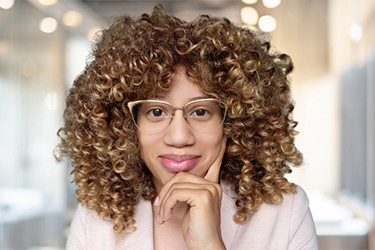
Thanksgiving is a time of year when we find ourselves in the company of family members we rarely see. Aside from eating a massive dinner and trying not to nod off while watching football, one of the unspoken traditions is simply catching up with everyone. Inevitably, this leads to a question like, “What’s new at work?” This year, when faced with that query, I may have … overdid it.
Instead of quickly explaining that I was now focused on writing about clinical trials, I ignored the blank stares after that comment and double downed with a full-on recounting of a recent interview I had done with Denise Bronner Ph.D., director of diversity, equity, and inclusion in clinical trials at Johnson & Johnson Innovative Medicine (JJIM). My enthusiasm was likely a reflection of Bronner’s own passion for the subject (i.e., DE&I initiatives in pharma), which I know sounds cliché considering … well, duh, it’s her job to talk about DE&I. But she went in so many different directions than I expected, and her message never drifted into that hacky, standard platitude you hear over and over from pharma execs — “We’re great because we care about our patients and getting a diverse cross section of patients involved in trials.” Yuck.
Leverage Data From Multiple Sources
Usually when I talk with someone about DE&I in pharma, the crux of the conversation revolves around the effects of better community engagement or empowering patients to be more knowledgeable about participating in clinical trials. Instead, Bronner talked a lot about data: where to get it, why much of it is flawed, and how we can leverage new sources of it to strategically attack the industry’s DE&I problem.
She starts by noting that inclusion/exclusion criteria tend to be very restrictive; the byproduct of the practice of templatizing protocols and only making minor changes for the drug being developed. The problem is that those templates may be based on historical demographic data that is old or incomplete and not representative of new research that includes other races. As an example, she brings up a word that dominates much of her argument — access. “This is something people have a hard time wrapping their heads around because they are so used to relying on prevalence and incidence data,” she says. “Look at IBD [inflammatory bowel disease]. When examining the access piece of it, you start to uncover disparities that non-white patients have to deal with, such as not having a specialist within their community or bias from physicians.” For the latter, some doctors may rule out the possibility that a black or Hispanic patient could even have a disease like IBD simply because the historical data doesn’t support such a diagnosis. Consequently, they may be labeled as having something like IBS, and thus, not entered into the system (i.e., ICD 10 code) correctly. “That claims dataset is going to be skewed,” she says. As further support of this conclusion, Bronner cites a 2019 study that showed a 134% increase in IBD diagnosis for non-whites from 1970 to 2010.
Beyond prevalence/incidence data, Bronner suggests analyzing de-identified datasets from population-based claims databases (e.g., IBM MarketScan, Optum Insight Databases, KOMODO Health) as well as census data and trial site locations. Having all that information allows you to see diagnosis, labs, scans, and other medical-related information along with demographics, location, and lifestyle data all to help determine if there are any trends. In addition, she notes there are companies such as Acclinate that have data about connecting with communities of color, or Power, which offers information about what clinical trials are available, but in a simpler format for patients. Access to that kind of info has an additional benefit — it can then tell you what separates your trials from others.
“You need to consider the datasets you are using when designing a protocol,” Bronner says. “Are they too U.S.-centric, for example. After all, the U.S. market is very different than other markets around the world, especially when it comes to privacy issues.”
AI Is Not A Silver Bullet
Any discussion of leveraging multitudes of data inevitably leads to someone mentioning AI as the solution for how we can make sense of all those ones and zeros. In this case, it was me who asked about AI, but Bronner made a good point in her response. Namely, AI is only as good as the data it is pulling from. “We're never really going to have a full picture; there’s going to be some gaps,” she says. “But with social media, Fitbits, apple watches, etc. you're getting so much more data now. You just have to be very mindful of what the system is being based on, and figuring out how you can fill in that missing data.”
It was at this point in our interview that the discussion segued into one of those unexpected directions I mentioned earlier. It was a more long-term, future-focused approach to improving diversity in clinical trials, and I’ll cover it in part 2.