5 (Fairly Simple) Statistical Analysis Plan Elements To Implement In Your Clinical Study
By Elaine Eisenbeisz, Omega Statistics
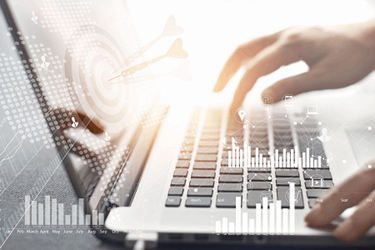
My father used to say, “If you are going to do something half-assed, don’t do it at all.”
This advice is perfect for approaching a research study. Planning on the front end can save headaches and wasted effort in the long run.
Certainly there are times when a page or two of statistical considerations inside of a protocol is sufficient and a 40-page statistical analysis plan (SAP) is a bit much. But regardless of the size of the study, proper planning with careful considerations of the “what-ifs” along with the “whys” will help in troubleshooting the inevitable deviations from protocol that occur in research.
In this article I will present five often ignored elements of a solid statistical analysis plan that help to address those “what-ifs” and will make troubleshooting the inevitable deviations easier. As you will see, a little thoughtful consideration of what could go awry up front will keep you from pulling out the duct tape and hammer to jerry-rig after the fact.
Element 1: Assure an adequate sample size by considering the desired effect size
Thirty subjects per group is a good rule of thumb for a large enough sample size, if you are referencing a textbook and using a t-test to compare mean differences between two groups of subjects (e.g., a t-test would be used to test the mean difference between product and placebo groups).
In real life, depending on the nature of the tests used and the comparisons you want to make, you often need much more than 30 subjects per group. Not including enough subjects will result in a study that is underpowered. There is nothing more disappointing to me than to see a very large difference in an endpoint between the treatment and control groups, but a p-value greater than that magical p <.05 we all love to see. A p-value is the probability (the “p” in p-value is actually short for “probability”) that, assuming the null hypothesis is true (the null hypothesis is the one that states there is no difference), one would see the observed results or greater if the test was to be reproduced with the same steps using a different sample of subjects.
For example: Using a t-test for a mean difference between product and placebo groups on the endpoint of platelet counts, I have a mean difference of 15,000 and a p-value of .05. This means that there is a 5 percent probability that if I were to conduct the same study with a different sample of subjects, I could expect to see this big of a difference between the groups, and still call them equal. Since 5 percent is small, it would be rare to see such a difference in platelet counts between two groups that are supposed to be equal, so we would call this difference statistically significant. On the other hand, statistics works in a way that you can beat the system and get a very low p-value if you enroll many more subjects than are needed to power the analysis. This type of study is overpowered. In a highly overpowered study you can find statistical significance in all of your endpoints, even on the tiniest effects. However, I rarely see an overpowered clinical study because each enrolled subject increases the monetary and temporal costs. But, theoretically, it is possible to achieve significance with a very large sample.
The effect size is a very important part of determining the sample size that is best for the study. Many investigators I work with want to design a study to find a significant difference between the product and placebo. Of course, we want that low p-value. But the focus should be on the desired differences or associations in the outcomes between the two groups. This difference or association, the effect size, could be the mean difference. For an overall survival endpoint, the effect size could be a median difference of survival times between groups, or perhaps a log ratio of two hazard rates. Correlation coefficients are effect sizes, too. It is important to know the size of the effect that is considered clinically significant before we can determine a sample that will show statistical significance. So for the simple product versus placebo comparison, a good first question would be “What is the smallest difference between the two groups that would be meaningful in practice?” This is the same as a statistician asking, “What effect size do you want to use to power this study?”
Aren’t sure about the test (Difference in medians? Difference in proportions? Correlation? Something else?) or effect size (Median difference of two years? 10 percent improvement? Correlation coefficient of .40?)? Then check the literature. Google is a good friend at times like this. But the investigator knows best.
Want to DIY a power analysis to determine your sample size? GPower is free software you can download online. If you can pay, PROC POWER in SAS software is also useful, especially when backed up with simulation studies. And PASS software by NCSS is a fan favorite of researchers.
Element 2: Attrition happens; plan for it early
I strive to power a study for a desired effect and add a cushion, at least 10 percent, for attrition. And, if the study is years long, I would probably increase the attrition percentage a bit more. Again, Google is our friend, and a review of the literature can give an indication of attrition rates in similar studies. Another way to save a study from the ravages of attrition (and other unusable data) is to plan for it at the beginning in the protocol or SAP.
It is often wise to establish two study populations in clinical research: (1) intention-to-treat (ITT) and (2) per-protocol (PP). Using two populations for data analysis will allow for better testing of efficacy endpoints, and efficacy is what usually matters most to the researcher. I especially recommend this approach for superiority and non-inferiority trials. Here is a brief reminder of the two types of subject populations:
ITT population includes all subjects who were randomized into the trial, no matter what happens to them afterward. ITT populations can have incomplete records on the efficacy outcomes.
PP population includes only subjects who completed all treatments or requirements needed for determining the efficacy endpoints. PP populations will have complete records on the efficacy outcomes.
This concept is surprisingly easy to include in a study plan, and it will allow you to study the efficacy of treatment on those who complete the full regimen, while still using the ITT population for the safety analyses. A simple section in the protocol and/or SAP is usually all that is needed. Here is an example:
5.1. Analytical Populations The safety population consists of all subjects who participated in at least one site visit after randomization into the trial. The per-protocol (PP) population consists of all subjects who completed all site visits and had no protocol deviations that (in the judgment of the principal investigator) would have invalidated their efficacy data. |
If it is after the fact and you didn’t plan for two populations, then get your hammer and duct tape and Google “sensitivity analysis.”
Element 3: Multiplicity? At least consider it
Multiplicity can arise in a study due to multiple primary endpoints, multiple comparisons, or multiple time points (repeated measures). Multiplicity is a big reason for Type I error, which is seeing significant findings for an effect when it is truly not there. Many corrections are available to adjust for Type I error. An easy one to use is the Bonferroni correction, where you divide your alpha level and by the number of tests you perform. However, this adjustment and many others are quite conservative. Being too conservative in controlling for Type I error could result in Type II error, which is not seeing significant effects that are really present. So goes the give-and-take of a study design and analysis … you can’t have it all!
It is desirable to avoid or reduce multiplicity as much as we can, especially for a confirmatory study. (It is not a big issue for exploratory designs.) Three steps that can help during the design phase are:
- specify one primary endpoint (adjusts for multiple variables)
- specify one primary post-hoc or contrast test (adjusts for multiple comparisons)
- use summary measures such as area under the curve for time (adjusts for repeated measures).
Sometimes it is not possible to have just a few tests. According to the FDA guidance:
In confirmatory analyses, any aspects of multiplicity that remain after steps of this kind [the above steps] have been taken should be identified in the protocol; adjustments should always be considered and the details of any adjustment procedure or an explanation of why adjustment is not thought to be necessary should be set out in the analysis plan.1
So, basically, one needs to consider the multiplicity and document what, if anything, will be done to control for it.
Element 4: GIGO (garbage in, garbage out)! Mind your data!
Remember that Google is your friend? Mine too! Read this article I found online about the regulations and best practices for data management:2
http://doi.org/10.4103/0253-7613.93842
Following is a data cleaning checklist I like to use as a general reference.3 Each study has its own anomalies and needs, but at a minimum this checklist is a good start:
Screening Data Checklist
1. Inspect univariate descriptive statistics for accuracy of input
a. Out-of-range values
b. Plausible means and standard deviations
c. Univariate outliers
2. Evaluate amount and distribution of missing data; deal with any problems accordingly
3. Check pairwise plots (if needed) for nonlinearity and heteroscedasticity
4. Identify and deal with non-normal variables and univariate outliers
a. check skewness and kurtosis, probability plots
b. Transform variables if needed/desired
c. Check results of transformations
5. Identify and deal with multivariate outliers (if needed)
a. Variables causing multivariate outliers
b. Description of multivariate outliers
6. Evaluate variables for multicollinearity and singularity (if needed)
Element 5: SAP after the fact
OK, so you didn’t write an SAP and have completed the study. You know there may be some issues to deal with. Perhaps you lost some subjects and now have holes and unequal sample sizes. Or something else didn’t go as planned and you are not sure if the plan will still hold. All may not be lost.
A blinded review can be done by the statistician after data is collected and prior to breaking the blind. A blinded review means the statistician does not know the subjects’ assignments into the treatments. So, now you may need two statisticians, one for the blinded review, and another to perform the analyses.
The SAP can be reviewed and updated, or even compiled from scratch, during a blinded review.
However, the SAP should be finalized, meaning signed by everyone necessary (sponsor representative and statistician at the very minimum) before breaking the blind.
Further, only the findings that were planned for and amended in the protocol can be confirmatory.
Summary
Low p-values are worthless if the study is improperly designed and analyzed. Some thoughtful planning prior to initiating the study can help a researcher anticipate and control for the inevitable deviations from protocol that will occur. Special consideration should be given to issues that may compromise study power and issues of subject attrition, because efficacy outcomes are best informed by subjects who have fully complied with the protocol and have completed all treatments.
It would be wise to, at a minimum, think through the possibilities of things that could go awry in the study and have a contingency plan written in the SAP early on. Or if this was not done, to review the data and protocol before the blind is broken. Remember, even the best-laid plans often fall short. But if you think ahead, you may save money on duct tape and hammers, and your study will be well-informed from the beginning.
References
- FDA Guidance for Industry, E9 Statistical Principles for Clinical Trials (1998) http://www.fda.gov/downloads/drugs/guidancecomplianceregulatoryinformation/guidances/ucm073137.pdf
- Krishnankutty, B., Bellary, S., Kumar, N. B. R., & Moodahadu, L. S. (2012). Data management in clinical research: An overview. Indian Journal of Pharmacology, 44(2), 168–172. http://doi.org/10.4103/0253-7613.93842
- Tabachnick, B.G., & Fidell, L.S. (2013) Using Multivariate Statistics, 6th ed. Boston, MA: Pearson Education, Inc.
The DIY researcher can find a comprehensive statistical analysis plan template at
http://www.cuh.org.uk/sites/default/files/research/CCTU_TPL007_SAP_template.doc.
About The Author
Elaine Eisenbeisz is a private practice statistician and owner of Omega Statistics, a statistical consulting firm based in southern California.
Eisenbeisz earned her B.S. in statistics at UC Riverside, received her master’s certification in applied statistics from Texas A&M, and is currently finishing her graduate studies at Rochester Institute of Technology. She is a member in good standing with the American Statistical Association and a member of the Mensa High IQ Society. Omega Statistics holds an A+ rating with the Better Business Bureau.
Eisenbeisz works as a contract statistician providing study design and data analysis for private researchers and biotech startups as well as for larger companies such as Allergan and Rio Tinto Minerals. Throughout her tenure as a private practice statistician, she has published work with researchers and colleagues in peer-reviewed journals. You can reach her at (877) 461-7226 or elaine@OmegaStatistics.com.