How Machine Learning Will Eliminate Failures In Clinical Trials

By Ed Miseta, Chief Editor, Clinical Leader
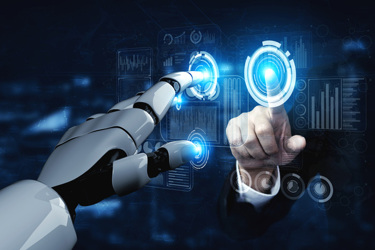
One of the hottest topics in drug development is the use of AI in clinical trials. AI is defined as a science concerned with building smart machines capable of performing tasks that typically require human intelligence. AI’s full potential will not be realized for at least another decade, but machine learning (a component of AI) is a technology that is available now. Still, judging by the amount of content and webinars I see on this topic, it seems many in the industry are still trying to understand how it will be used and why it may be a game changer when it comes to trial conduct.
If you have a small amount of data from two variables, it is easy to plug that information into a spreadsheet, graph it, and look at the relationship between the variables. That process becomes more complicated when you have multiple variables and millions of data points. For years, the number of data points collected in a trial has been growing, and one study notes the amount of data collected in a trial is currently growing by 40% per year. That big data problem makes the industry a perfect fit for AI and machine learning solutions.
I have interviewed several experts on the topic and the best description I have heard of its potential use in trials came from Elvin Thalund, director of industry strategy at Oracle Health Sciences. Thalund recommends looking at machine learning as a telescope that allows you to see into the future.
Every clinical study comes with many pitfalls that can derail the trial. And every trial failure increases the time and cost of bringing a new treatment to patients. If pharma companies were able to look into the future, they could identify those pitfalls in advance and avoid or eliminate them. Best of all, this does not simply apply to late-phase trials. We can use that telescope to predict future results before any trials have even started. The telescope can be done when companies first start to develop their clinical development plan.
A New Approach
The current trial process is time consuming and costly. It costs, on average, $2.6 billion to bring a new treatment to patients. And each approved drug must cover its own costs as well as the costs of other treatments that failed the trial process.
Anyone familiar with the current drug development process knows it is difficult and loaded with challenges. It starts with a clinical plan and development of a protocol. Sites are then asked how many patients they can recruit. Some recruit the number they promise. Some get more or less than the number forecasted. Many trials are cancelled for not recruiting enough patients. Approximately 50% under enroll patients. Approximately 10% will not enroll a single patient.
Now imagine this scenario: You are developing a treatment for pancreatic cancer. You are able to use a machine learning solution to determine whether a molecule might be a good candidate for that disease. It could also be used to determine if the number of patients you require for the study is attainable. Next it will tell you which cancer patients would be the best fit for your product, based on personal characteristics and characteristics of their tumor. You can also eliminate those patients who will likely not benefit from the treatment. Incidence of disease is not the same in all areas of the world, so you can determine which countries will most likely provide access to the patients you require. Once you identify the right countries in which to perform your trial, you can also identify which clinical sites in that country will be most successful at recruiting the patients you require. You could also determine which CRO would be the best fit for your study, based on global presence, expertise, and past performance. And best of all, that required information could be gleaned by using hard data, not by human guesswork.
A Bright Future, Available Now
If a company were able to do all that, trials would be more successful, they would take less time, and the number of failures would plummet. The cost of trials, and the corresponding cost of medicines, would also fall. That, in a nutshell, is the promise of AI and machine learning. One AI expert I spoke to noted that $2.6 billion average cost to bring a new drug to market could drop by as much as one-third.
Much of these potential savings could be realized now. The roadblock currently preventing this is existing business structures and processes. Machine learning is being utilized too late in the process, oftentimes after critical decisions are made. Today, country and site selections are made after a study has been approved by portfolio management, which often leads to rescue studies, as study teams are given a square peg (study) and asked to put it in a round hole.
Machine learning is available and ready to prevent the need for rescue studies. Key features[1] needed to do accurate study feasibility (for example, determining which countries and sites are required), are available in the Clinical Development Plan. Machine learning could be used at the portfolio level of planning to make more detailed study design decisions. By using machine learning in the planning process, those studies that are more likely to result in failure would not be approved or would be approved with a different study design. For example, a trial might be approved with a decentralized study design as opposed to a traditional study design.
To get to the point where AI and machine learning will have a significant impact on trial conduct, much more needs to be done. What I keep hearing from key opinion leaders is that we do not have a technology problem. The technology works and vendors are ready to assist you in this endeavor. But what pharma does face is a human understanding problem. For AI to assist with clinical development, researchers and trial planners need to understand the technology, know what data is required, know how to access the right data, and most importantly, know how to ask the right questions. There are challenges that need to be overcome, but the future of clinical trials is bright and will only get better with the use of AI and machine learning.
[1] Indication, Phase, Subject populations, Key inclusion/exclusion criteria, approximate Subject numbers and Treatment durations