Novartis Turns To Digital Technologies For Clinical Trials

By Ed Miseta, Chief Editor, Clinical Leader
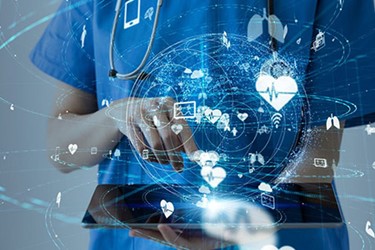
New and innovative technologies are making their way into clinical trials. But which have the greatest potential to transform studies?
"We are currently seeing emerging digital technologies which have an incredible potential to unlock the next chapter of medical innovation,” says Jake LaPorte, head of digital development for Novartis. “Artificial intelligence (AI) and the Internet of Things (IoT) are two disruptive but complementary technologies that are beginning to find broad applications, not just in pharma but in all of our society. They’re likely to have profound effects on the underpinnings of our economy and our society more broadly, much as the internet has.”
According to LaPorte, it’s only logical that new technology platforms, such as AI, machine learning, and the IoT will impact healthcare and, more specifically, pharma. Massive computing power and sophisticated algorithms provide us the opportunity to improve the drug discovery and development process.
Clinical IoT refers to the ability of patients to wear mobile devices and sensors that will capture and provide a stream of quality, nearly real-time data to researchers. AI is the technology those researchers will use to analyze the data and look for information, insights, or patterns. It has been defined as machines being able to perform “smart” tasks that are characteristic of human intelligence. Machine learning is a term that refers to the ability of AI algorithms to learn and develop without being explicitly programmed.
“At Novartis, these technologies can mine our vast chemical, biological, and clinical data sets to generate insights and find new targets in ways that are really beyond what scientists alone can do,” states LaPorte. “I actually see a day in the not too distant future where AI techniques are leveraged to design novel molecular entities with a high probability of success in a clinic.”
ALL WE NEED IS GOOD DATA
In clinical trials, clean, curated data is all that is needed for AI to start producing insights. LaPorte describes data as the fuel for AI. As patients begin to use wearable devices, those sensors will provide researchers with much larger data sets and more real world data. That real world data will complement the discrete data sets collected by nurses and physicians in clinics.
“These larger data sets will allow AI algorithms to start gaining more insights into medical and biological breakthroughs,” says LaPorte. “That is the high-level context I am looking at when devising our digital program at Novartis. Right now there are limited options for clinical-grade sensors that are appropriate for clinical trials like the actigraphy watches available from Philips designed to track patient activity. I believe the data we collect will continue to improve as we see more of these devices making their way into trials.”
Furthermore, LaPorte believes the clinical IoT community is starting to recognize that we are witnessing the dawn of a unique digital health signature that is specific to each person and will be composed of manifold health measurements. These measurements will continue to evolve over time and will eventually have a data density that far exceeds almost any other current health data set. It will obviously include measurements like activity, blood pressure, and heart rate. However, it will also include data on how you’re interacting in society (i. E. what people have you called today), the locations you have visited, and how the burden of your disease is affecting your social and economic contributions. We may even see more advanced digital measurements like nerve impulses and EKGs recorded continuously.
“All these things will combine to create a unique digital signature,” notes LaPorte. “Imagine when we are able to take this data and scale it up. It will give us a tremendous amount of information that we can analyze to develop new understandings of disease progression and pathways. That knowledge will allow us to create much more tailored drug development programs that focus on specific areas with a much better understanding of the disease pathology behind them.”
NEW ALGORITHMS ARE A GAME-CHANGER
Prior to the advancements of AI and machine learning, researchers worked only with “dumb algorithms.” They were designed by humans to interpret the data fed into them. With machine learning, algorithms can now learn how to interpret data more precisely and accurately.
![]()
"That knowledge will allow us to create much more tailored drug development programs."
Jake LaPorte
Head of Digital Development, Novartis
“This is the beauty of AI,” says LaPorte. “By ingesting millions and millions of data points and images, we can start to find things that we would have otherwise not seen with the human eye or with the dumb algorithms used in the past.”
LaPorte cites two examples of the technology in use. A university recently designed a machine-learning algorithm that looked at brain scans of healthy patients and those with Alzheimer’s. Researchers were able to train the algorithm to detect Alzheimer’s disease earlier than what would have otherwise been possible by a physician. The algorithm also was able to determine which mild cognitive impairment images would actually lead to Alzheimer’s, leading to greater options for treatment.
Another example involves work being done at Novartis using ophthalmology clinical data that it had collected related to age-related macular degeneration. The company is now working with the University of Vienna in Austria to have an algorithm examine images of the retina. Although the work is still in preliminary stages, the machine-learning algorithms already have been able to detect nuances in the images that could be used as biomarkers. The biomarkers can predict the disease 12 to 18 months prior to the traditional symptoms of age-related macular degeneration presenting themselves.
“When you start using these new technologies, it creates real value for patients,” states LaPorte. “We will soon be able to predict the progression of diseases much earlier and then develop interventions that provide a better prognosis to patients. These technologies also will help R&D folks think about new ways to understand disease pathology and new targets to create therapies around.”
DESIGN BETTER TRIALS
LaPorte believes new technologies will soon be pervasive in clinical development. For example, Novartis has created a proprietary machine-learning predictive-analytics platform called Nerve Live. To create the platform, Novartis partnered with QuantumBlack, which rose out of Formula One racing. QuantumBlack started out collecting data from races and using machine learning and predictive analytics to optimize race operations. It has since become part of McKinsey & Company and expanded into life sciences and other markets.
“We have started to bring Nerve Live online and input clinical operations data,” says LaPorte. “In pilot cases we have found that it can help our global drug development teams plan and simulate country-allocation scenarios for clinical studies, such as choosing the best sites, tracking trial enrollment, and predicting patient enrollment curves. Nerve Live is now fully operational, with five modules, including the trial footprint optimizer, already rolled out and available, and future modules in development.”
The whole pharma industry is focused on patient-centricity, and LaPorte believes AI will help with that mission, as well. “Wearable devices will now allow us to take more patient measurements remotely. Eventually we are going to get better data coming back from these devices. That could potentially reduce the signal-to-noise ratio and actually reduce the number of patients we will need in a trial.”
- CLINICAL IOT (INTERNET OF THINGS) - Patients wearing mobile devices and sensors that capture and provide a stream of quality, nearly real-time data to researchers .
- AI - Technology used to analyze large datasets gathered from patients to identify information, insights, or patterns .
- MACHINE LEARNING - Refers to the ability of AI algorithms to learn and develop without being explicitly programmed