Principles For Creating A Successful ML Data Governance Strategy
By Pankaj Manon, Chief Technology Officer, ThoughtSphere and Christina Dinger, Sr. Director of Product, ThoughtSphere
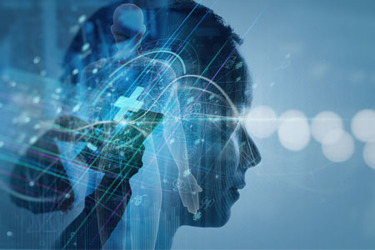
To continue bringing effective and safe treatments to patients in wake of the industry’s unprecedented surge in data, clinical researchers are capitalizing on new technological advancements. In recent years, trial organizations have implemented AI/ML models to measure data veracity, provide new data insights, and expedite data mining capabilities.
However, insights from the ML models are only as good as the data input to train the models and the governance measures put in place to monitor the models. In clinical research and healthcare, the risks of poor data quality go far beyond financial impact, with people’s lives hanging in the balance. With such high stakes, it is imperative to implement sound ML data governance processes to ensure reliable data and ML predictions are made available to clinicians and clinical research stakeholders.
Implementing best practices and data governance procedures will improve the availability and quality of training datasets and provide mechanisms to monitor and identify model degradation. Consider these key principles when developing and implementing ML governance policies.
Get unlimited access to:
Enter your credentials below to log in. Not yet a member of Clinical Leader? Subscribe today.