The Next Three Years Of Clinical Trials: DCTs, RWE, And Beyond

By Ed Miseta, Chief Editor, Clinical Leader
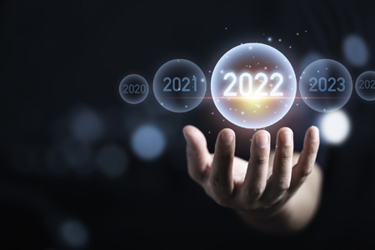
Recent years have seen unprecedented innovation in the clinical space. Precision medicine, cell and gene therapies, decentralized trials, real-world data, and the promise of artificial intelligence (AI) and machine learning (ML) are just a few of the reasons to be excited about the future of clinical research. But what can we expect to see in the next three years, and what are the challenges sponsor companies will need to overcome?
A webinar hosted by IBM Watson hoped to answer those questions. The discussion featured Lorraine Marchand, general manager of life sciences at IBM Watson Health; Nimita Limaye, research VP, Life Sciences R&D Strategy and Technology at IDC Health Insights; and Greg Cunningham, director of the RWE Center of Excellence at Eli Lilly and Company. The three shared insights into what we might expect to impact trials over the next three years.
In this article the panel discusses precision medicine and real-world data. In part 2 of this article the panel looks at the future of decentralized clinical trials.
The Growth Of Precision Medicine
The first game changer the panel discussed is the advancement of precision medicine. It has moved from exploring single gene mutations to performing research using combinations of genes. This change has the potential to bring better drug targets forward and get the best products to patients faster.
“This has been playing out in the last decade in oncology real-world evidence,” notes Cunningham. “We've seen an evolution in precision medicine as we've built out the patient record. As we have done that, the marketplace has evolved rapidly, particularly for electronic medical record data and genomic data.”
Pharma companies were happy to get their hands on electronic medical record data. When genetic test results were combined with that data, researchers gained the ability to look at a single mutation and develop better patient outcomes.
Where precision medicine will continue to evolve in 2022 and beyond is the growing use of genetic testing in oncology. This will provide the industry with more data about patients. With more genes at their disposal, researchers can look at groups of genes and the complex combinations of gene mutations. This has the potential to open the door for tools like artificial intelligence to help researchers analyze the complex number of permutations.”
RWD Creates More Efficient Research
Next the panel discussed RWD and the ability to utilize it across several use cases from discovery and development to commercial. Limaye likes the prospect of being able to create a data exchange where researchers can bring together claims, clinical, EMR, and genomics data directly from patients to create an intelligent and digital patient health record. That record gives researchers the digital equivalent of a real-life patient which can be used as a natural history or synthetic control arm in randomized control clinical trials.
These data can allow drug developers to track patient response to drugs and look at outcomes after being exposed to new therapies. The promise of data and technology is using tools like AI to advance therapies and get them to patients faster. This will be done with better information and a much more efficient way to perform drug development and track and monitor outcomes in patients.
Big data has been a topic of discussion in pharma for years. The volume of clinical data is now growing exponentially. Approximately 30% of the world's data volume is being generated by the healthcare industry and by 2025, the compound annual growth rate will hit 36%. That's 6% faster than manufacturing, 10% faster than financial services, and 11% faster than media & entertainment.
In addition to getting bigger, data is also getting broader. Researchers can not only look at a patient’s medical history but can now consider factors such as social determinants of health and behavioral data.
Since most EHRs do not include genomic data, researchers need the ability to look at patient data more holistically. Type 2 diabetes was one example discussed. Today, 40% to 70% of it is genetically inherited and there are over 500 different genetic loci which could be involved in causing the disease. The earlier strategy of looking at genetic risk scoring was single trait. That is now transitioning to multi-trait research with an integrated view that will drive a precision medicine strategy. This creates an interesting situation where drug discovery will continue to get more specific and focused towards an individual while also getting bigger and broader.
The Challenge Of RWD
With access to RWD, drug developers can benefit from data they may not have known existed. Although the data is rich and robust, it can be difficult to access. One of the biggest challenges the industry faces is data stored in silos. The panel notes data is stored in patient claims, electronic medical records, in lab apps, images, and genetic files on a smartphone. Having the technology to tap into those sources to identify quality data is the primary challenge.
The data must be de-identified for patient privacy, cleaned, curated to remove noise, and enriched, which means bringing together the various components that will be meaningful to drug development. That will allow researchers to have a patient record that is useful across pharma, from development through to commercial. An exchange would enable that exact process – a platform where various entities can bring their data to have it linked, integrated, cleaned, and enriched, creating a data package that can be plugged into studies.
An important component of that exchange is the data being housed in a place where various third parties can feel comfortable bringing their data to match it with data from other third parties.
Cunningham cites lupus as an example of where pharma could benefit from such an exchange. “I would like to have a complete data set of lupus,” he says. “Lupus is an autoimmune condition, and a quintessential data set could be used for a number of uses, such as preparing a Phase 1 trial, selecting patients, or understanding patient responses to different therapies when designing studies. Specific data sets could be created for each therapeutic area, and pharma companies need that hard work of bringing the data together removed.”
Data Assembly And Analysis
Currently, drug developers spend 80% of their time assembling data and 20% of their time analyzing it. The situation must be flipped so that 80% of the time is spent performing analysis. The panel recommends rethinking how health records are created. The healthcare and life science industries require the ability to easily put data together. That comes back to investing in data standards everyone can agree upon. With the right standards and technology, the industry can spend its time improving lives as opposed to assembling data.
The FDA has indicated it is aware and supportive of the fact that pharma needs use RWD in drug discovery. The industry now needs to create the interoperability, standards, and methods to ensure that data can be included in regulatory submissions. This evolution may be akin to the critical path initiative. When the FDA embraced the idea of the critical path and allowing more in silico modeling of clinical trial design and development, it took the industry almost 10 years to adopt and apply the guidance.
The FDA has said it recognizes the importance of RWD, but that acknowledgement has resulted in few approvals. Looking at the use of synthetic control arms and RWD in regulatory submissions over the last five years shows just 10 submissions and all were in oncology. Only one was a successful submission, and the rest were rejected because of lack of completeness of the data.
Those numbers should tell the industry the FDA is not going to dictate how to get to approvals. The industry is going to have to figure out the interoperability and how to apply the standards. Regulators are always going to require quality data. Industry will need to enrich the data and create the cohort that is going to be equivalent to a patient in the real world.
In part 2 of this article, the panel discusses the role of technology in clinical trials, how decentralized trials will continue to evolve, what capabilities sponsor companies will need, and whether decentralized trials might offer cost benefits to companies.