What Can Advanced Analytics Do For ClinOps?
By Ed White, head and senior director of trial optimization & advanced analytics, and Randy Krauss, head and executive director of metrics, analytics, and performance, Merck & Co.
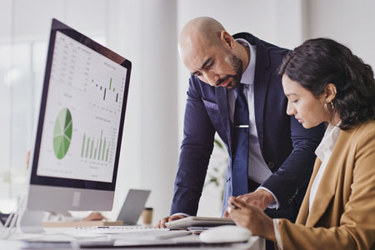
Approximately 30 years ago, a large provider organization sought to develop metrics and insights into a 100,000-member insured population as this provider organization accepted a risk contract from an insurer. This exercise included an effort to identify subjects from the population with high-risk chronic conditions so we could implement programs to mitigate the individuals’ risks. The tools and technology available at the time required what seemed like endless hours of programming, computation, and analytics over a week into the weekend to calculate the defined set of 10 to 15 metrics and a patient list for three to five chronic conditions.
Today, with the advent of technology and data, we now have tremendous power such that the above exercise could be accomplished in a fraction of the time while processing more than double the quantity of data! This progression of technology and data has yielded a new field of data science, or advanced analytics, to unlock insights and actions in the world of business.
Advanced Analytics For Clinical Operations
Advanced analytics (AA) for clinical research operations involves the application of progressive technical and computational techniques to enhance the efficiency, effectiveness, and outcomes of clinical research operations. AA leverages statistical modeling, machine learning, data mining, and other methods to analyze and extract insights from diverse biomedical and clinical research data sources.
Here are some key applications of advanced analytics in clinical research operations:
- Predictive Modeling: Advanced analytics techniques can be used to build predictive models to forecast important clinical research metrics such as patient recruitment rates, dropout rates, and adherence to study protocols. These models can help researchers optimize study designs, identify potential challenges, and make informed decisions about resource allocation.
- Patient Segmentation and Personalized Medicine: Advanced analytics can assist in identifying patient subgroups based on various demographic, genetic, and clinical characteristics. This segmentation enables researchers to develop personalized treatment approaches, identify potential responders or non-responders to therapies, and design targeted clinical trials.
- Real-Time Monitoring and Adverse Event Detection: Advanced analytics can be employed to analyze real-time data generated during clinical trials, such as electronic health records (EHRs), wearable devices, and patient-reported outcomes (PROs). By monitoring data in real time, researchers can identify adverse events (AEs), detect safety signals, and make prompt adjustments to study protocols as needed.
- Protocol Optimization: Advanced analytics can guide the optimization of clinical study protocols by analyzing historical data and identifying areas for improvement. It can help identify factors that impact study outcomes, refine inclusion and exclusion criteria, and facilitate evidence-based decision-making during study design.
- Data Integration and Interoperability: With the increasing volume and diversity of data in clinical research, advanced analytics plays a crucial role in integrating and harmonizing data from multiple sources. It enables researchers to combine structured and unstructured data, such as EHRs, genomics data, imaging data, and clinical trial data, to gain comprehensive insights and support research initiatives.
- Risk Assessment and Management: Advanced analytics techniques can be used for risk assessment and management in clinical research, including predicting potential risks, identifying risk factors, and developing risk mitigation strategies. This helps ensure participant safety, regulatory compliance, and the successful execution of clinical trials.
- Operational Efficiencies and Optimization: Advanced analytics can reveal previously unknown patterns in data, especially in working with unstructured data for clinical operations. Through advanced analytics, data scientists can predict where the business will be going and/or understand the root cause of patterns in operational data. With this understanding, corrective mitigations can be tested and implemented to attain desired results.
By leveraging advanced analytics, clinical research operations can be optimized, resulting in improved study designs, enhanced participant outcomes, and accelerated drug development processes.
How Predictive And Prescriptive Analytics Can Drive Value For ClinOps
There will always be a need for traditional metrics and descriptive and diagnostic analytic reports and tools to measure and understand operational performance in clinical operations. Advanced analytics moves organizational business intelligence beyond the descriptive and diagnostic exercises of dashboarding and reporting (what or was and why) to customized predictive and prescriptive solutions (what will be and what we need to operationally change) to facilitate organizational understanding from data and information. Additionally, advanced analytics seeks to provide business insights using progressive statistical, data manipulation, and technical programming techniques to unlock business insights from internal data sources and/or external data assets procured to drive business value.
For ClinOps at our company, we have worked with advanced analytics in several areas to drive value from clinical operations:
Institutional Performance
For many years we have had a wealth of internal clinical operational data available. Our key issue was the lack of a universal definition of how we quantitatively define institutional performance via a singular metric using this data. Before implementing the Institutional Performance Tool (IPT), many different functions throughout the organization had localized, non-vetted definitions of institutional performance. Most of these definitions and calculations were also maintained by colleagues with suboptimal data literacy. (More on data literacy and its essential role in advanced analytics later.)
For our IPT initiative, we gathered key organizational stakeholders to align on the quantitative/analytical definition of a high-performing institution. Once we aligned on this definition, we worked with data scientists to apply this definition to the data so each institution's performance could be measured, ranked, and placed in one of five performance categories. The solution, with visualizations, was deployed via a web-based platform so all users could transparently evaluate and compare institutions globally. Institutional performance results can be used to understand relative institutional performance, highlight dimensions of an institution’s performance, and prioritize institutions for participation in our clinical trials.
Study Planning & Strategy
Like many ClinOps organizations, we have the ongoing challenge in study planning to select the best countries and sites for enrollment (both total enrollment and diverse enrollment), operational efficiency, and quality. Additionally, our enterprise procures external data assets that can provide insights into the above questions via medical claims, electronic medical record (EMR) data, registries, prescribing pattern histories, and epidemiology. Although we have enterprise licensing of these data assets, they were not previously used to inform ClinOps. With the addition of a data science team and access to these assets, we can transform these raw data assets into solutions that inform country selection, site selection, provider practice patterns, and patient disease progression. These insights are key in de-risking our clinical trial portfolio via driving enrollment (rate and number enrolled), identifying investigators who can enroll diverse populations, optimizing the global footprint of our trials based on prescribing patterns and epidemiology, and further understanding geographic differences in disease epidemiology, practice patterns, and regulatory environments.
Operational Efficiencies
As much of our operational data was in both unstructured and structured formats, we previously were limited to gaining operational insights exclusively from structured data. With the arrival of advanced analytics and our data science teams, we can now apply natural language processing (NLP) techniques to identify previously hidden patterns in our unstructured operational data. Examples of early success in this area are in NLP of query text and issue management for monitoring.
Data Literacy And Business Acumen Are Foundational For AA Success In ClinOps
Data literacy refers to the ability to read, work with, analyze, and communicate with data. A classic challenge in business intelligence (and, consequently, advanced analytics) is there are often two key parties that need to collaborate in delivering value using advanced analytics — data scientists and business leadership. A common knowledge gap for these parties in delivering results with advanced analytics is in data literacy — knowing how the data is generated, structured, and stored to reflect business operations. Literacy is key so this data can be used to measure business performance and drive business success.
Without sufficient data literacy, business leadership is inadequately prepared to specify what and how we should measure the business and/or build algorithms to drive success and/or performance improvement. Data scientists’ deficiencies in data literacy manifest in a lack of understanding of the business and value propositions to drive performance improvement.
To develop data literacy, business leadership and data scientists can undertake various steps, including:
Training and Education: Business leadership must understand how data is structured and reflects the business environment of clinical operations. Data scientists must understand the value propositions of the business and how data can be used to drive value for clinical operations. The ability to read a graph or data visualization is insufficient; deriving actionable insights with the ability to ask a follow-up question to facilitate understanding is important.
Practical Experience: Both data science and clinical operations leadership must persist in improving data literacy by embracing a culture of learning, both in the business of clinical operations and the technical capabilities of advanced analytics tools. As we are in a dynamic environment with ongoing technical development and increasing availability of data, a commitment to continuous improvement in this area is essential to retaining relevance.
Collaboration: Learning and delivering incremental value with advanced analytics occurs through the "bringing together" of the core data science and business thought leaders. Value comes from collaboration between these professionals to learn from their experience, exchange knowledge, and seek guidance when working with complex data sets.
By developing data literacy, individuals can harness the power of data to improve decision-making, drive innovation, and achieve organizational goals.
Looking Ahead
The progression of data availability and technology over the last 30 years has yielded a new discipline of data science to unlock insights into the modern business environment, including pharmaceutical clinical operations. Several specific business areas were discussed with specific examples presented where value for advanced analytics has been realized. A key foundational determinant of success for any clinical research operations advanced analytics initiative is the successful and ongoing development of data literacy and business acumen by business leadership as well as data science colleagues. Collaboration among these two stakeholders is key to ensuring success with any advanced analytics initiative. Finally, tactics were discussed to ensure the ongoing development of data literacy and business acumen in the ever-changing operational and technical environment for clinical research operations.
References:
- Raghunathan, R. et al. (2014). Advanced Analytics for Clinical Research. Applied Clinical Trials. Retrieved from: https://www.appliedclinicaltrialsonline.com/view/advanced-analytics-clinical-research
- Chen, Y. et al. (2019). Applications of Advanced Analytics in Healthcare Research: A Comprehensive Review. Artificial Intelligence in Medicine, 101, 101725.
- Kuhn, M. et al. (2019). Advanced Analytics for Precision Medicine: Leveraging Longitudinal Electronic Health Records in Predictive Modeling. WIREs Computational Statistics, 11(4), e1463.
- Be Data Literate: The Data Literacy Skills Everyone Needs to Succeed. Jordon Morrow (Kogan Page; 2021)
About The Authors:
Ed White is the head and senior director of trial optimizaion & advanced analytics within global clinical trial operations at Merck & Co., Inc., Rahway, NJ, USA. In this role, Ed and his team support feasibility modeling for late-phase enrollment planning as well as data science initiatives to inform study planning and larger clinical operations initiatives.
Ed began his career working in business intelligence (BI) for healthcare provider organizations. He later expanded his BI contributions to health insurance and medical device organizations before arriving at Merck & Co., Inc., Rahway, NJ, USA in 2009. Ed has held BI-related positions in several different divisions and functional areas throughout the company.
Ed received his B.S. from the University of Delaware and his MBA and MS from Temple University. He also has certifications in Business Intelligence (MCITP, MCTS) and Project Management Professional (PMP).
Randy Krauss is the head and executive director of metrics, analytics, and performance within the global clinical trial operations at Merck & Co., Inc., Rahway, NJ, USA. In this role, he and his group support the optimization activities of planned studies, as well as oversight of portfolios, studies, and processes. Before joining Merck & Co., Inc., Rahway, NJ, USA in 2016, Randy worked in similar roles at Genzyme (Sanofi), Shire, and Alexion.
Randy began his focus on this research discipline at Genzyme, where he served as director of metrics, analytics, and performance. Randy also spent four years at the Boston University School of Medicine as a lab coordinator and a biochemistry instructor.
Randy received his B.S. from the State University of New York at Fredonia and his Ph.D. in microbiology from the University of Alabama at Birmingham. He completed his post-doctoral fellowship at the University of Massachusetts Medical Center in Worcester.