Why We Need Technology Consolidation In Clinical Trials

By Dan Schell, Chief Editor, Clinical Leader
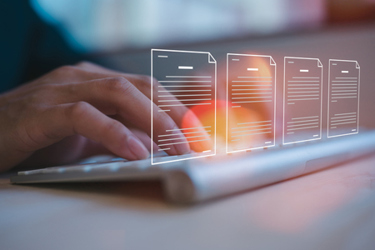
“If you were asked to design the perfect clinical trial process, I don’t think you’d end up with what we have today,” says a data management expert from a Big Pharma that I recently spoke with. “Sure, we’ve evolved from paper trials to a more digitized landscape, but it’s a patchwork of systems, and we're all trying to knit the data together.”
Figuring out how to interconnect disparate systems and standardize data has always been a primary function of a data manager’s role. But in the past decade, the number of data points that need to be collected for a trial has grown exponentially. New protocol designs that incorporate, for example, ePRO and eCOA systems, are fueling this growth as is the proliferation of exploratory endpoints. Gone are the days when data was collected, entered into a database, cleaned, and then shared at a later point in time. Now, a plethora of internal stakeholders want access to trial data as soon as it’s generated (or soon thereafter), not only for safety and medical review, but also for determining if a protocol needs amended or adjusted. “Trying to keep up with this volume and pace of change means we [i.e., data managers] need to be constantly evaluating and implementing newer technologies,” the expert says.
TOO MUCH TIME AND EFFORT DEDICATED TO MOVING DATA
As we all know, there is no silver bullet to solving all these data-related problems. My contact did say that tech vendors like Veeva (there are plenty of others) are essential to helping the industry reduce this current patchwork of systems and maybe even move to single sign-on. Some of the questions data managers should be asking include:
- Are there two systems with functions that are slightly aligned?
- Can they be one system in the future?
- Can we aggregate some systems or data?
- Can we create a cloud platform infrastructure so we're not plugging data in from here to here?
“All of that takes time and effort to implement and to monitor. You can't just set up an integration and walk away; you have to keep an eye on it to make sure it's working. We need this sort of consolidation as we go forward, because it's very hard to speed up when you're still connecting things.”
When talking about streamlining the protocol development process and making it more efficient, the expert talks about how information from a trial protocol often will be used to “build” the EDC and set up the CTMS. “There's no flow of information with that scenario. If we move to having a digital starting point of the visit structure and the needed assessments, you can just use that data flow to set up the EDC, CTMS, and other downstream systems. Today, we spend a lot of effort moving data from one system to another, interpreting it and often replicating it. We shouldn't be here, but we are, although we're trying to get to a point where we can leverage that information downstream. If we can do that, then we can automate the system setups, which will save time.”
THE ROLE OF TODAY’S DATA MANAGER
Beyond the technology itself, we talked about the role of the data manager in today’s trials. My contact believes, for example, that data managers should be involved early in the protocol design process to validate that what is being proposed is technically feasible. This is especially important with complex trials such as basket, umbrella, or DCTs where the source of the data varies.
Those complex trials have created the need for more specialized roles within data management. Some of those roles include dedicated SDTM (Study Data Tabulation Model) programmers, clinical trial data managers who are project managing and leading the trial, and people dedicated to data cleaning.
“When I started in data management, roles were more consolidated,” the expert says. “You had a kind of data manager who did everything from system setups to project management to data cleaning. Hopefully in the future, as things simplify in the system landscape and we’re able to automate more by using tech like AI and machine learning, we should be able to again consolidate some of these roles. But right now, you still need someone who is the face of data management on a study team and then have more technical people looking after system setups and data flow and SDTM mapping.”