AI Is Ready To Impact Clinical Trials

By Ed Miseta, Chief Editor, Clinical Leader
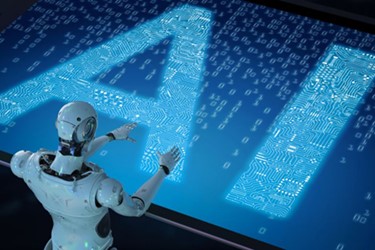
Artificial Intelligence (AI) is making its way into the realm of clinical trials. While most of the talk I hear seems to center on clinical trial recruitment and using AI to mine electronic medical records (EHRs), that application seems to only scratch the surface. Experts predict monitoring drug adherence, pre-emptive risk monitoring, decision-making, diagnostics, and process optimization are other areas where the technology is expected to make an impact. By the middle of 2020, the AI market for healthcare is expected to top $35 billion, and big names such as Microsoft, Google, and IBM are already collaborating with top universities to further AI.
We engaged experts from four of the largest companies in the industry to provide insights on the implementation of AI in clinical trials and the challenges companies are facing. This is part one of a two-part article. Part 2 of the article can be seen here. The experts are:
Lucas Glass, global head in the Analytics Center of Excellence at IQVIA
Craig Lipset, former head of clinical innovation for Pfizer
Victor Lobanov, VP, informatics solution development at Covance
Mike Montello, SVP of R&D technology at GSK
The opinions expressed in this article are the opinions of the individuals themselves and do not necessarily reflect the views of their respective companies.
Ed Miseta: Can you provide an example of new capabilities that more modern data science has opened in your organization?
Craig Lipset: One of the most exciting areas for modern data sciences in medicine development has been protocol design and optimization. Development organizations have long made use of diverse data during protocol development, including epidemiologic data, past performance data, and intelligence data. Modern data science and 21st century approaches have both expanded the diversity of data used as well as enabled smarter use of available data. For example, protocol designers today are expanding their data resources with both real-world data to optimize eligibility criteria and understand available patients, while teams are also engaging directly for active patient insights.
Rather than these data all landing as incremental data feeds to protocol authors, AI and thoughtful algorithms are increasingly supporting teams with proactive data-driven insights. The opportunities in this area will continue to expand with the adoption of the TransCelerate Common Protocol Template and the opportunities of AI-driven increased automation downstream of the protocol. Modern data science applied to protocol development will accelerate the creation of smarter studies, with fewer protocol amendments and greater confidence that trials will more efficiently understand the efficacy and safety of new medicines.
Mike Montello: At GSK, a combination of capabilities, orchestrated together as a technology platform, powers data science. The capabilities include human genetics and functional genomics data, high-performance cloud computing, advancements in machine learning and deep learning algorithms, collaborations including 23andMe, and domain and scientific expertise. Orchestrated together, these capabilities power analytics which have the potential to improve the probability of success of new medicine as they enter clinical development. We have leveraged these new capabilities at almost every point in the pipeline, from early discovery through to patient identification.
Victor Lobanov: Open source availability of advanced machine learning techniques, such as convolutional neural networks (CNNs), and pre-trained deep learning models, like Bidirectional Encoder Representations from Transformers (BERT), opened up exciting opportunities for the drug-development industry. We can now develop and deploy robust image analysis solutions for biomarker evaluation and natural language processing (NLP)-based techniques for unstructured text processing with modest effort and training data requirements.
The immediate opportunities we are focused on include identification of eligible patients for clinical trials, automation of imaging-biomarker analysis, and forecasting trial performance through a deeper learning from historical data and study protocols. For example, Covance is using a fine-tuned BERT NLP model to automate extraction and codification of the eligibility criteria from protocols to enhance study feasibility assessment, planning and forecasting. The traditional predictive analytics capabilities also have improved thanks to the greater availability of data-modeling tools, convenient cloud-based computing infrastructure, and a new generation of trained data-science professionals.
Lucas Glass: Modern data science has opened an opportunity to support sponsor companies with drug discovery. Drug discovery has traditionally been a laboratory and bench science domain. However, with an explosion of AI in clinical development, we can now make important contributions as a human data science company. We were already utilizing machine learning for disease modeling and phenotyping. It was only a minor pivot to then leverage our data assets and data scientists for supporting drug discovery AI research.
Miseta: Focusing on the area of clinical development, what areas are most suited for disruption with predictive analytics, machine learning, and AI? And what makes these areas so well suited?
Montello: As software matures to leverage machine learning, logic will use annotated data sets versus hard-coded rules for decision making. There are many areas of clinical development which can benefit from the technology, and the areas which are best suited have historic, high-quality, annotated data sets. Natural language processing and translation engines can enable automation in clinical operations. Voice can increase engagement of both patients and investigators. For the next generation of risk-based monitoring, machine learning algorithms can compare metrics of an in-flight trial to past trials to predict quality issues at investigator sites and adverse reactions in high risk patient populations.
Lobanov: It has been demonstrated that AI can achieve and even surpass human accuracy in the pathologic diagnosis of cancer or in the detection of diabetic retinopathy. For example, AliveCor developed an AI algorithm that can detect atrial fibrillation and hyperkalemia using the ECG signal captured by an Apple watch. Algorithm development required processing of more than million ECGs and more than four million potassium measurements to achieve the 94 percent sensitivity. Stanford University has developed an AI algorithm to diagnose melanoma based on processing of 130,000 images of skin lesions. AI is exceptionally well suited to process huge amounts of data in search of meaningful patterns. As we continue to accumulate observational data, clinical outcomes, genetic sequences, imaging biomarkers, wearable signals and other data modalities, we will leverage machine learning and AI across the board.
Starting with protocol design, we can better utilize observational, epidemiological and safety data for the identification of the right patient population, inclusion and exclusion criteria, and sample size. For instance, the VAERS database managed by the U.S. Centers for Disease Control (CDC) and the FDA has more than 10 years of vaccine safety data and can be used to help plan clinical trials that optimally determine the efficacy and safety of a new vaccines. The Duke University Databank for Cardiovascular Disease includes a very large number of patients with acute coronary syndrome (ACS), which may be used to accurately predict the outcome of clinical endpoints and inform the statistical power of an ACS study required to demonstrate the effect of a new treatment. Cooperative groups such as the National Surgical Adjuvant Breast and Bowel Project (NSABP) have similarly large data for the breast and colorectal cancers where machine learning algorithms may be used to simulate outcomes.
Mining healthcare utilization data with machine learning and NLP approaches can facilitate identification and recruitment of patients for clinical trials. We can identify both the geographic locations of eligible patients as well as the seasonal patterns of disease incidences. For example, not only can we predict the timing of an allergy season, we can also predict the strength of the allergy season as well, thus being able to inform planning and forecasting of the trial schedule.
Using cognitive computing to automate core monitoring and management functions in the drug development process is another promising area. Automation is already used in safety processes for collection, triage and coding of adverse events in specialized databases. Automation has been proven to be faster, more accurate and more cost effective than humans. New algorithms in the works are leveraging NLP and deep learning to facilitate automated safety signal detection and risk-benefit analysis of ongoing clinical trials. AI algorithms can also monitor continuous measurements from wearable devices such as heart rate, blood pressure, and glucose levels to detect abnormal patterns and alert both patients and physicians. This can potentially have a dramatic impact on both patient safety and data quality in clinical research.
Glass: Site selection is an area in clinical development that is primed for disruption with machine learning and AI. As an industry, we are highly adept at collecting data on site performance as well as physician and facility demographics. Further introduction of multimodal real-world data via machine learning algorithms has created a lift over traditional site selection approaches – even data driven ones – that makes the old paradigm untenable. We see this shift, however, as an augmented intelligence play rather than a pure AI play. The site selection experts, empowered with the algorithms, still outperform the algorithms alone. Furthermore, if we want to continue to progress data science in the feasibility domain, as an industry we must collaborate around consistent empirical definitions of performance. Too often we see claims of faster enrollment or better site lists that would not hold up under peer review. This coordinated and disciplined data science approach to site selection and feasibility can accelerate research and start to establish gold standards in methodology and machine learning within the domain.
Lipset: Digitally-savvy medicine development organizations are pursuing several AI/ML-driven use cases. Robotic Process Automation (RPA) is one area well-suited for adoption, as organizations can often develop and validate automated solutions for repetitive tasks and ensure the legacy approaches are retired. Content generation and quality control is another popular area for use cases, with tools developed for other verticals using structured data for authoring becoming increasingly accessible. While AI in that use case may be creating content from structured data, AI can also make an impact in the opposite direction with NLP helping to bring structure to unstructured data. A prime example for NLP would be the potential to electronically source study data from free text notes. While predictive algorithms can make a great impact to study monitoring and data quality oversight, these algorithms also bring the potential to support new endpoints based upon diverse data. Perhaps among the most challenging use cases, the need for new endpoints is significant and collaborations are already showing progress to help validate digital endpoints.