AI Moves Forward, Despite Challenges And Pitfalls

By Ed Miseta, Chief Editor, Clinical Leader
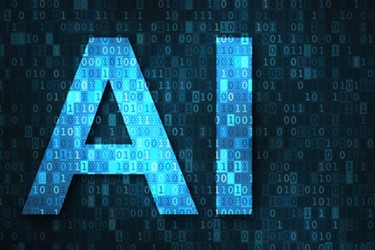
Artificial Intelligence (AI) is finally making its way into the realm of clinical trials, but not without challenges that need to be overcome. In part two of this roundtable discussion on AI, our experts examine some of the challenges arising, operationalizing AI at the point of care, and the barriers that must continue to be overcome. Part 1 of the article can be seen here.
We engaged experts from four of the largest companies in the industry to provide insights on the implementation of AI in clinical trials and the challenges companies are facing. The experts are:
Lucas Glass, global head in the Analytics Center of Excellence at IQVIA
Craig Lipset, former head of clinical innovation for Pfizer
Victor Lobanov, VP, informatics solution development at Covance
Mike Montello, SVP of R&D technology at GSK
The opinions expressed in this article are the opinions of the individuals themselves and do not necessarily reflect the views of their respective companies.
Miseta: Have you faced challenges with enriching vs replacing existing processes with AI and automation? How have you addressed these challenges?
Glass: We have faced and will continue to face challenges on a regular basis. The key to overcoming them is trust. We build healthcare algorithms for healthcare experts who all have an understandable bias towards evidence and transparency. We address this challenge in three ways. First, agile software development. The domain experts are involved in the algorithm development and are regularly directing and redirecting the research. Final decisions still lie with the product managers, but we have found it much easier to convince physicians to use an algorithm when they are involved in the development. Second, we address this issue with white box AI. Algorithms that are interpretable are easier to sell to a client who is skeptical of AI/machine learning . However, there are occasions when white box algorithms require a trade-off in accuracy that we are not willing to make. Third, augmented intelligence. In certain instances, we only make recommendations to the experts. We then track when and why the recommendations were rejected so that our algorithms can learn. This has allowed clients to watch the algorithms improve over time which enables us to gradually gain increased trust.
Lipset: Much of the life science industry tends to be "additive" -- some of the increasing complexity of trial protocols can be attributed to the introduction of new approaches without necessarily removing the old. Many times, this is viewed as a strategy for risk-mitigation -- teams are reluctant to "burn the boats" and opt to keep both old and new approaches running concurrently. With so many diverse stakeholders involved -- including regulators, investigators and patients -- it is understandable that there would be some fear to go from additive to replacing. The industry can only realize the full potential of digital tools today through confidence and commitment, moving from thoughtful experiments to implementation at scale including retiring outdated approaches.
Montello: Every technology has an adoption curve, and in GxP operations, the adoption timeline for any change is long. To address the adoption challenge, the best approach is to focus and prioritize discrete activities to automate. For example, in pharmacovigilance this could be auto-classifying an adverse event. In trial master file processes, it could be checking the quality of a document which was scanned by a CRA at an investigator site. Privacy is another challenge, balancing the depth of de-identified patient data and risk. In addition, an agile culture that takes smart risks is also critical to move next generation technology forward.
Lobanov: The key challenges with applying AI and AI-driven automation in clinical development are access to labeled training datasets, validation of continuously learning algorithms and integration of the AI solutions into existing data flows. Training machine learning algorithms requires a lot of data. For example, tuning NLP models to recognize medical conditions, drug names, thresholds for laboratory results and negations in subject eligibility criteria from clinical protocols requires detailed human annotations, which takes a deliberate effort to produce. AI systems have the ability to continuously learn during use, which presents a challenge of when and how these systems should be validated. In one instance we have taken the approach in which the NLP model annotates data for the human operator to verify. This approach allows us to collect additional training examples and periodically update and re-validate the model. True continuous learning in a fully automated scenario requires first gaining experience and trust with AI-augmented approaches and evolution of the regulatory framework. The recently published FDA discussion paper on the “Proposed Regulatory Framework for Modifications to Artificial Intelligence/Machine Learning (AI/ML)-Based Software as a Medical Device (SaMD)” is a welcome step in the right direction.
I would add that clinical development often relies on systems that were developed long before today’s advances in cloud computing and micro-service architecture. Embedding AI automation into operational processes faces additional integration hurdles in system access and validation. Emergence of the new data interoperability standards like Fast Healthcare Interoperability Resources (FHIR) will be essential for the broader adoption of AI for clinical development and healthcare processes.
Miseta: There have been publicized shortfalls in the use of AI at the point-of-care. Still, this is a tremendous opportunity. What can be done to operationalize AI at the point-of-care?
Lobanov: FDA approval of the IDx-DR AI method last year for detection of diabetic retinopathy was a key milestone for point-of-care AI use. Nascent opportunities abound, including the potential for improved diagnosis in radiology and tissue pathology, where complex pattern recognition is appropriate input for machine learning. Some are even pointing machine learning to complex tasks involving resource optimization and human values, such as allocation of kidneys for transplantation. Ultimately, trust is what is most necessary for AI to become successful in healthcare – trust by practitioners as well as patients. Transparency is essential to this outcome, as illustrated by the website WhoGetsTheKidney.com, which is dedicated to organ transplantation noted above.
Glass: This is an exciting area for data science. There are a few technology initiatives aimed at making this opportunity more feasible. For example, FHIR and SMART on FHIR are striving to drive API standards that can make scalability of technology more feasible. These ecosystems are still relatively new so further efforts for these initiatives should be encouraged. More importantly, however, technology companies must integrate more closely with the physician community. There have been many well publicized collaborations between tech companies and hospital systems, but the industry needs to take the collaboration a step further. Including physicians as part of the data science teams not only ensures that the algorithms are clinically sound, it also ensures that what gets built is something that the customers – practitioners – will want.
Montello: It seems AI is embedded in every solution being offered today, to the point AI has leaped off the hype curve. In GxP and clinical settings, the production use of the technology to aid decision making including diagnosis, is still limited. The black box of AI needs validation, and insights need to be reproducible. To operationalize AI, high quality validation procedures which have been leveraged to validate software and statistical programming must be adapted and applied to software with embedded machine learning algorithms.
Miseta: What do you see as the biggest barriers in leveraging AI and machine learning to drive business impact in life sciences?
Lipset: The biggest barrier in leveraging AI in the business today is one of partnering and scale. Innovative new partnership models are needed to help companies apply modern approaches to rapidly experiment and expand the range of organizations able to work against prioritized challenges. We are seeing companies embracing some of these approaches -- from incubators and accelerators to venture investments. More of these innovative partnering models will continue to emerge, helping to increase the pool of partners and efficiency of the partnering process.
Glass: The biggest challenge is overcoming the trust gap. Physicians mistrust AI because it can often be a black box and there is a perception that it will attempt to make them obsolete. Life sciences are the domain of the clinician, and technology has been unceremoniously forced upon them. My wife, who is a physician, tells me that technology has reduced the efficiency of the patient interaction and made it less personal. Good medicine, she says, is a deeply personal business where the clinician will counsel families on matters of life and death. As we introduce AI into the life sciences, we can’t lose sight of that important function or we will not succeed.
Montello: There is a risk that a machine learning model could produce a misleading result. To enable larger scale adoption, the focus must be on engineering data flows from acquisition, annotation, through to analysis to lower the chance of error and to protect privacy. We have to work hard to standardize measurements in order to enhance data sets which could carry bias. We will work to overcome these barriers, as the technology has the potential to accelerate development and bring new, transformational medicines to patients in need.
Lobanov: There is a lot of media buzz about AI, and its potential is truly transformational. But the hype might create unrealistic expectations and disillusionment in short term. The key to success is to pick the right problems to solve that have sufficient data to learn from, meet current regulatory requirements, overcome technology and adoption challenges, and provide a clear return on investment. While most business leaders are aware of AI’s potential, there is a shortage of practical experience with AI adoption. The technology is not fully formed, and the workforce must develop much-needed AI competencies. Closing the skill gap, establishing organizational AI strategy and leadership, and focusing on business efficiency and strategic goals are necessary to drive the impact.